|
|
|
ABSTRACT |
The purpose of this study was to explore the reliability of estimating three-dimensional (3D) linear kinematics and kinetics of a swimmer derived from digitized video and to assess the effect of framing rate and smoothing window size. A stroke cycle of two high-level front crawl swimmers and one high level backstroke swimmer was recorded by four underwater and two above water video cameras. One of the front crawl swimmers was recorded and digitized at 50 Hz with a window for smoothing by 4th order Butterworth digital filter extending 10 frames beyond the start and finish of the stroke cycle, while the other front crawl and backstroke swimmer were recorded and digitized at 25 Hz with the window extending five frames beyond the start and finish of the stroke cycle. Each camera view of the stroke cycle was digitized five times yielding five independent 3D data sets from which whole body centre of mass (CM) component velocities and accelerations were derived together with wrist and ankle linear velocities. Coefficients of reliability ranging from r = 0.942 to r = 0.999 indicated that both methods are sufficiently reliable to identify real differences in net force production during the pulls of the right and left hands. Reliability of digitizing was better for front crawl when digitizing at 50Hz with 10 frames extension than at 25 Hz with 5 frames extension (p < 0.01) and better for backstroke than front crawl (p < 0.01). However, despite the extension and reflection of data, errors were larger in the first 15% of the stroke cycle than the period between 15 and 85% of the stroke cycle for CM velocity and acceleration and for foot speed (p < 0.01). |
Key words:
Inverse dynamics, reliability, swimming, asymmetry, Butterworth filter
|
Key
Points
- An inverse dynamics based on 3D position data digitized from multiple camera views above and below the water surface is sufficiently reliable to yield insights regarding force production in swimming additional to those of other approaches.
- The ability to link the force profiles to swimming actions and technique is enhanced by having additional data such as wrist and foot velocities that can be obtained readily from the digitized data.
- Sampling at 25 Hz with at least 5 samples before and after the period of interest is required for reliable data when using a 4th Order Butterworth Digital Filter.
|
Elite swimmers are characterised by their ability to reduce the resistive forces from the water, thus achieving fast swimming velocities with sustainable effort (Cappaert et al., 1996). Therefore the analysis of resistive forces during swimming, otherwise known as ‘active drag’, is fundamental to understanding how swimming performance can be improved. Several means of measuring active drag experimentally have been used by researchers. These include the Velocity Perturbation Method (Kolmogorov et al., 1997), and the Assisted Towing Method (Mason et al., 2011). While these methods provide an indication of active drag over the period of a complete stroke cycle, they cannot provide accurate detail of the temporal pattern of net forces acting during a stroke cycle because the instantaneous force reflects the motion of the attachment point of the force measuring device to the body, rather than reflecting the acceleration of the whole body mass. The ‘Measurement of Active Drag’ (MAD) system (Hollander et al., 1986) measures forces directly as the swimmer pushes against a series of underwater fixed plates while swimming. A concern with this system is that stroke kinematics and torques acting on the body differ from those in free swimming thereby affecting body alignment and drag. Further, the force generated by the pushing action of the upper limbs is measured without consideration of the leg kick, thus not providing an accurate representation of the swimmer’s propulsive output. Detail regarding the temporal pattern of the net force provides insight into the effectiveness of the swimming technique. For example, large fluctuations in net force indicate that the resistive force is much larger than the propulsive force and vice versa. Thus, when the acceleration of the whole body centre of mass (CM) is measured, phases of the stroke cycle in which forces are not being applied effectively and when the body encounters great resistance can be identified and linked to the technique of the swimmer to improve performance. Intra-cyclic velocity variability (IVV) has been analysed by researchers with the rationale that there is an inverse relationship between magnitude of the difference of maximum and minimum velocity within a stroke cycle and swimming efficiency or skill (Barbosa et al, 2006; Cappaert et al., 1995; Togashi and Nomura, 1992). The IVV of the hip during the propulsive phases of right and left upper limbs is frequently used to assess asymmetries in bilateral contributions to propulsion (Payton and Wilcox, 2006). However, the velocity and derived accelerations based on the motion of the hips have been shown to differ considerably from the motion of the CM (Figueiredo et al., 2009; Psycharakis and Sanders, 2009). Psycharakis and Sanders (2009) found mean errors in CM velocity of 10% for maximum and minimum velocity and 20% for the range of velocity relative to mean velocity of male front crawl swimmers. Similarly, Figueiredo et al (2009) reported RMS errors in velocity over the stroke cycle of front crawl swimmers ranging from 0.16 to 0.30 m·s1 and RMS errors in acceleration between 5.38 and 7.86 m·s2. These findings indicate that acceleration of the hip does not reflect the acceleration of the CM and cannot be used with confidence to assess effectiveness of technique in minimizing active drag. Three dimensional (3D) analysis of IVVs based on velocity of the CM derived from digitized video data has been conducted recently (Figueiredo et al, 2009; Psycharakis et al., 2010). However, assessment of velocity changes without calculating or considering forces, limits the confidence with regard to interpreting swimming effectiveness. For example, an inefficient swimmer with a high stroke frequency can have smaller IVVs than a swimmer with a low stroke frequency despite having larger fluctuations in net forces. That is the duration and pattern of the forces, and their links to the swimming actions, must be taken into account. Acceleration of the CM, and therefore net force, can be obtained using the inverse dynamics approach defined by Whittlesey and Robertson (2004) as ‘the process by which forces and moments of force are indirectly determined from the kinematics and and inertial properties of moving bodies’. The position of the CM can be obtained by modelling the body as a series of rigid links from which the CM position is obtained for each video frame. Velocity of the CM is the first time derivative of the CM position and acceleration is the second derivative. The segment mass and centre of mass locations relative to digitized landmarks defining the segment are input to a computational routine that calculates CM by taking moments that is multiplying the segment mass by the perpendicular distance (its respective x, y, or z coordinate) of the segment centre of mass about orthogonal reference axes and dividing by whole body mass. The accuracy of the estimate of the CM then depends on the accuracy of the estimates of body segment masses and their centre of mass locations. These can be improved by ‘personalising’ the data to the characteristics of the participant. Jensen (1978) developed a personalised model of the human body in which each body segment comprised a stack of elliptical zones of 2cm depth. In Jensen’s elliptical zone method, the diameters of the ellipses are obtained by tracing, with a mouse-controlled cursor, the outlines of the images of the segments from front and side camera views. By using the inverse dynamics approach in conjunction with digitized video data and body segment data obtained by the elliptical zone method, the low frequency (<4Hz) force-time profiles can be derived to within 3% of the actual forces (Sanders et al., 1999) when digitizing is conducted using automatic marker identification techniques. Thus, the inverse dynamics approach can be very useful in swimming research to provide detailed information about velocity fluctuations throughout the stroke cycle, the forces causing those fluctuations, and their links to swimming technique. To date, automatic digitizing of a whole body rigid system has been problematic due to the difficulty of tracking markers continuously in an aquatic environment. Tracking of markers is adversely affected by water turbulence, presence of bubbles/vortices and the regular disappearance of body segment markers due to the rolling motion of the swimmer, motion of other body segments, and the transition of body parts across air and water mediums. Consequently, manual digitizing is necessary to minimize errors. A major problem of applying the inverse dynamics approach to manually digitized data is that random errors are amplified with each derivative (Winter, 2009). A fourth order Butterworth digital filter is commonly used to remove random error and thereby reduce the errors in the derived velocity and acceleration data. However, the filter uses data on either side of the point being corrected and so data points near the start and finish of the data set cannot be filtered. Various means of extending the data prior to digital filtering have been tested. For example Vint and Hinricks (1996) found that errors in acceleration in the period of 10 frames from the end of the data set were reduced by extrapolating the data using either linear extrapolation or reflection. Giakis et al (1998) found that a 20 point extrapolation based on a least squares fit of the final 10 data points was superior to other methods of extrapolating including linear extrapolation, linear autoregressive modelling (D’Amico and Ferrigno, 1990), and natural spline functions. Nevertheless, an error in the last 10 points was greater than in the other regions of the data set. Therefore, to attain reliable derived data at the endpoints of the data set it is evident that additional points should be digitized rather than extrapolating from the data points in the data corresponding to the period of interest. The question of how many additional points need to be digitized to obtain accurate and reliable results is critical for two reasons. First, manual digitizing of several camera views is tedious and time consuming. This limits the number of subjects, trials, and stroke cycles that can be digitized in a study. Second, if a large data set is required, the field of view and the calibrated 3D space must be increased so that the whole swimmer is in view for the whole of the period digitized. This means that the image to be digitized is smaller, body landmarks harder to distinguish and, as a consequence, accuracy reduced. Therefore, it is advantageous to determine how many additional points are sufficient to obtain reliable results near the start and finish of the stroke cycle. While several researchers have used the inverse dynamics approach with manual digitizing to estimate three-dimensional kinematics of swimmers (Cappaert et al., 1995; Figueiredo et al., 2009; McCabe et al., 2011; Psycharakis et al., 2009; Sanders and Psycharakis, 2009) there is a paucity of information regarding 3D kinetics in swimming and the reliability of calculating them by inverse dynamics. Consequently knowledge of the patterns of net forces and their links to performance remains limited. The purpose of this study was to explore the reliability of estimating 3D linear kinematics and kinetics of a swimmer derived from digitized video and to assess the effect of framing rate and smoothing window size.
ParticipantsTwo male swimmers volunteered to participate in this study (S1: age: 18yrs; height: 1.81 m; weight: 72.6 kg: S2: 18yrs; height: 1.86 m; weight: 76 kg). S1 was a front crawl sprint specialist (front crawl: 50m front crawl long course PB 25.00s). S2 was a front crawl and backstroke swimmer (back crawl: 50m short course PB 25.26s; 100m 59.32s; 200m 2:08.2; front crawl short course PB 50m 23.32s; 100m 51.80s; 200m 1:51.81). The study was approved by the Research Ethics Committee and the par- ticipant provided informed consent.
Collection of anthropometric dataTo enable identification of the endpoints of body segments when digitizing the video recordings, black skin markers 4.5cm in diameter were applied to 19 anatomical landmarks (Figure 1). Marker locations were: the vertex of the head (using a swim cap), the right and left of the following: tip of the 3rd distal phalanx of the finger, wrist axis, elbow axis, shoulder axis, hip axis, knee axis, ankle axis, 5th metatarsophalangeal joint, and the tip of 1st phalanx (big toe). These markers defined a 13 segment body model comprising the head, neck, and trunk as a combined segment, right and left arms, forearms, hands, thighs, shanks, and feet. Each participant was photographed simultaneously by two digital cameras at a distance of 12m capturing orthogonal front and side views of the subject in accordance with the ‘e-Zone’ method described by Deffeyes and Sanders (2005) (Figure 1 and Figure 2). A bespoke MATLAB (Mathworks, Inc.) program, based on Jensen’s (1978) ‘elliptical zone’ method, was used to determine the segment masses and segment centre of mass positions relative to the segment endpoints. These data were then input to a MATLAB (Mathworks, Inc.) analysis program enabling calculation of whole body centre of mass, whole body velocity and acceleration.
Collection of video dataThe swimmers were tested on different days. Each swimmer performed their usual warm-up prior to the testing session for a period of 10 minutes. As there was a ‘no breathing’ requirement within the video capturing space, the swimmers were asked to familiarise themselves with this protocol during the warm-up. As S1 was performing just front crawl sprints and S2 was performing front crawl and back crawl the protocols differed slightly. The testing required S1 to perform 4 x 25m front crawl maximal sprints, and S2 to perform 4 x 50m front and back crawl sprints. Each sprint was initiated from a push start to eliminate any possible influence that a dive may have on the stroke kinematics. After each sprint, the swimmer swam a recovery pace 25-50m to the starting position, followed by a 2 minute stationary rest period in the water. The recovery method utilised in this study was in accordance with research highlighting active recovery, combined with passive recovery and water immersion following a bout of rigorous exercise, is more beneficial in terms of aiding the recovery process than passive recovery alone (Dodd et al., 1984; Choi et al., 1994; Wilcock et al., 2006). Each sprint was manually timed using a digital stopwatch. The fastest front crawl sprint of S1 (FC1) and S2 (FC2) and the fastest back crawl sprint of S2 (BS) were selected for further analysis. The testing sessions were conducted in a 25m indoor level deck swimming pool (average pool temp 29.5° ± 0.2). The volume within which the data were sampled was calibrated using a rectangular prism frame 4.5m in length (X direction), 1.5m height (Y direction), and 1.0m width (Z direction) (Psycharakis et al., 2005) for S1, and a frame 6.0m in length (X direction), 2.5m height (Y direction) and 2.0m width (Z direction) for S2. The frames were suspended to cover both above and below water surface so that approximately half was below and half above the water surface with the X axis aligned horizontally in the swimming direction, the Y axis vertical, and the Z axis horizontal and perpendicular to the X axis. The calibration frames were recorded by six gen-locked video cameras (four cameras below and two above the water surface) and digitized to yield separate calibration files for the above and below water views using the Ariel Performance Analysis System (APAS) which incorporates the direct linear transformation (DLT) algorithms of Abdel-Aziz and Karara (1971). The calibration frames used for S1 and S2 differed slightly in dimension, and so the fields of view of the cameras were larger for FC2 and BS than for FC1. The heights of all cameras were adjusted so that the control points on the calibration frame were clearly distinguishable. All six cameras recorded the motion of the swimmer at a sampling frequency of 50 fields per second and an electronic shutter speed of 1/120s enabling sharp images of the moving limbs for digitizing. Figure 3
Data processingOne front crawl stroke cycle (SC), defined as the period between the frame corresponding to the entry of the 3rd metacarpal tip of one hand to the instant of entry of the same hand which was manually digitized using the APAS system. The digitizing protocols differed so that the effect of frame rate and the number of additional points could be assessed. For the front crawl trial (FC1) of S1 the digitized data set included 10 video fields prior to, and beyond, the defined SC. Every video field i.e. 50Hz was digitized. For the front crawl (FC2) and the back crawl trials (BS) of S2, frames rather than fields were digitized, that is the frames had not been split into the two fields comprising the frame. Thus the data set included 5 frames prior to, and beyond, the defined SC. This meant that the real time duration of the recordings was similar and the field of view was consistent across FC1, FC2 and BS despite the difference in the number of frames digitized. In terms of the protocol, all that differed between the trial of S1 (FC1) and the trials of S2 (FC2 and BS) was the number of extrapolation points (10 vs 5) and the sampling frequency (50 Hz vs 25 Hz). Following DLT transformation the 3D coordinates of the above and below water were combined into a single file representing continuous coordinates throughout the SC. This process was repeated five times by the same experienced operator with digitizing conducted on separate days and no repeats of the same camera view on the same day. Separating the digitizing bouts temporally ensured that reliability wasn’t artificially improved by familiarity due to the practice effect of identifying the body landmarks. Each of the five repeat digitizations was input to a customised MATLAB (Mathworks, Inc.) analysis program to calculate all variables. A 4th order Butterworth filter with a 4Hz cut-off frequency was applied after extrapolating the data by reflection to an additional 20 points beyond the start and finish of the SC (30 points of additional data at each end for FC1 and 25 points of additional data at each end for FC2 and BS) as added insurance against distortion of the endpoints of the data set. The rationale for choosing 4 Hz as the cut-off was based on the Fourier spectral analysis indicating that little power (<1%) was contained in frequencies greater than 4Hz. Further, the spectral analysis indicated that the highest frequency with substantial amplitude, the ankles, approximated 3Hz. This corresponded to the six beat kicking motions with each kick completing three kick cycles in approximately one second (the duration of the stroke cycle). Given that stroke cycles are close to or greater than one second for all swimming strokes and distances, 4 Hz as a cut-off can be used generically in swimming as appropriate for retaining the real signal undiminished while eliminating most of the noise. Given that noise is amplified when deriving velocities and accelerations, selection of the smoothing frequency is critical to optimising reliability. The CM was determined by taking moments about the X, Y, Z reference axes of the segment centres of mass obtained using the digitized endpoint coordinates in conjunction with the segment masses and proportional segment centre of mass locations obtained from the e-Zone program. Linear velocity of the X, Y, and Z components of the wrist, ankle, and CM were determined using the first central difference formula. Resultants of the linear velocity components were determined by application of Pythagoras Theorem to indicate the overall magnitude (speed) of wrist and foot motion as these are important with respect to force generation by the hands and feet respectively. Component accelerations of the CM were determined using the second central difference formula. A Fourier transform and inverse transform was then used to interpolate the data to the equivalent of 200 samples per second. Expanding the data set by Fourier interpolation, retaining all Fourier harmonics, maintains the exact shape of the data profiles while increasing the precision of identifying the start and finish of the SC. Events defining phases of the swimming cycle, in particular, the instants of catch and release of the right and left hands could be precisely identified from the wrist X velocity data as the instant of first backward movement of the wrist with respect to the external reference frame (catch) following entry and the instant of last backward movement of the wrist with respect to the external reference frame (release) following the pull. After trimming the data to the period of the SC the data were then converted to 101 points, again by Fourier transform and inverse transform, representing percentiles of the SC. The use of the Fourier series transform is regarded as highly appropriate when analysing periodic data, such as in swimming (Bartlett, 1977).
Statistical analysisFor each variable of interest the standard deviation of the five digitizing trials was obtained for each percentile of the time profile. Average error was obtained as the mean of the 101 standard deviations and expressed as a percentage of the range of the data from minimum to maximum. The repeatability of the time-profile of each variable was obtained as R2 using the methods outlined by Kadaba et al (1989). A correlation coefficient R was also obtained as the square root of R2. Paired two-tailed t-tests, treating each variable as a ‘subject’ were conducted to determine whether there were differences in %error between FC1, FC2, and BS and paired one-tailed t-tests to compare average error between 0-15%, and 0-85%, and between 85-100% and 15-85% of the time normalised SC.
Table 1 displays the average error, range, average error expressed as a percentage of the range (average error%), R2 and R for the variables of interest. These include the CM velocities and accelerations in X, Y, and Z directions, and wrist and ankle speeds. Wrist velocity in the X direction and ankle velocity in the Y direction are presented due to their importance to propulsion given that the hands and feet respectively are the primary propelling surfaces (Toussaint, 2011; Ungerechts and Arellano, 2011). Mean average error% was significantly larger for FC2 than FC1 (5.10 v 3.56; p < 0.001) and for FC2 than BS (5.10 v 3.29; p < 0.001). There was no significant difference between FC1 and BS (3.56 v 3.29; p = 0.26). Table 2 shows the mean average error% for the first (0-15%), middle (15-85%) and last (85-100%) normalised time periods of the stroke cycle for CM velocity and acceleration, hand speed, and foot speed for FC1, FC2 and BS. Errors were larger (p < 0.01) during the first 15% than 15-85% of the SC for CM velocity and acceleration and foot speed.
Although 3D videography with manual digitizing is time consuming, and therefore not commonly practiced, it enables detailed interpretation of the net forces acting on a swimmer and the links to the swimmer’s actions and postures. However the confidence of the interpretations depends on the reliability of the data which, in turn, depends on the effect of random error introduced during the manual digitizing process. Thus, the purpose of this study was to explore the reliability of estimating three-dimensional (3D) linear kinematics and kinetics of a swimmer derived from digitized video and to compare the effect of framing rate and smoothing window size. The pattern of whole body velocity, particularly in the swimming (X) direction, is an aspect of great interest as it indicates the net effect of the propulsive actions and active drag. The average error of the instantaneous CM X velocities (0.014 m·s1) was 4.0% of the range in CM velocity in FC1, 5.23% in FC2, and 4.87% in BS. It is noteworthy that this is similar to the reliability in estimating the range of X velocity (3.67%) reported by Psycharakis et al (2009) using similar 3D data collection and analysis techniques. Thus, the reliability is good enough to compare the gains and losses of velocity between right and left arm actions. For example, in the case of FC1, there is a greater increase in CM X velocity from catch to release of the right hand than the left (Figure 4). It is clear that this swimmer has a smaller minimum CM X velocity prior to the force produced by the right hand than at the equivalent time of the pull of the left hand. The instant at which that minimum occurs coincides with the instant that the force produced by the right hand overcomes the active drag. The subsequent increase in CM X velocity during the pull of the right hand is greater than that during the pull of the left hand. This points to an asymmetry in possible force production of the right and left hands during their respective pull phases and therefore has implications for technique and strength training to develop a more even contribution of right and left pulls. Similarly in BS there was a greater loss in velocity of the CM between the left hand release and right catch than between the right hand release and left catch. Comparing between strokes, the range of CM X velocity was 0.55 m·s1 for BS compared to 0.37 m·s1 for FC1. However, comparison of change in velocity of the backstroke compared to front crawl in the same subject (FC2 v BS) is difficult due to the decreased reliability at the beginning of the data set in FC2. To delve more deeply into the contributions of the actions to propel the body the acceleration patterns of the CM can be very useful. The acceleration profile directly reflects the net force acting on the swimmer and therefore the interplay between propulsive and resistive forces. Because they are the second derivative of the CM displacement data, the accelerations are sensitive to error. However, with the data collection protocols and smoothing at 4 Hz the average error in CM X accelerations across the stroke cycle (0.244 m·s2) was 4.95% of the range of accelerations in FC1. As can be seen in Figure 5 the reliability is sufficiently good to indicate clearly some differences between the right and left sides in the production of force by this swimmer. Figure 5 also reveals that CM X accelerations in FC2 have high levels of reliability except for the beginning of the data set. This corresponds with the finding for CM X velocity. Similarly, the reliability of the backstroke data for both CM X velocity and CM X acceleration is very good except for the first 15% of the stroke cycle. Nevertheless, by comparing the acceleration profiles for the period between left catch and left release, a section of the data with high reliability, it is evident that the pattern of net force (indicated by the net accelerations) is different between the two swimmers performing front crawl sprints (FC1 and FC2) and between strokes of the second swimmer (FC2 and BS). Given the good reliability for FC1 the potential for detecting differences between trials within and between swimmers with confidence is apparent provided that there are close to 10 samples beyond the start and finish of the period of interest. For example, in the case of the trial of the swimmer studies in FC1 it is apparent that there is no difference between the maximum acceleration achieved during the pulls of the right and left hands. This underlines the importance of investigating the force profiles rather than relying only on the CM X velocity profiles. Given that greater velocity was attained during the right pull than the left one could have erroneously concluded that the right hand applies greater force than the left during its pull. However, inspection of the acceleration profiles reveals that the greater gain in velocity during the pull of the right hand compared to the pull of the left was due to differences in the pattern of net force production. In particular, the force was sustained longer near the time of the catch for the right pull than the left and also during the transition between the pull and push phases of the pull. While differences in net force can be revealed by the CM X acceleration profiles, it cannot be assumed that there are differences in the patterns of force applied by right and left hands. This is because there may be differences in the active drag during equivalent instants of the pull of right and left hand. For example, different postures may be adopted during equivalent phases due to asymmetries in body roll. Indeed, asymmetries in body roll, even in non-breathing cycles have been quantified in several studies (McCabe et al., 2011; Payton et al, 1999; Psycharakis and Sanders, 2008; Sanders and Psycharakis, 2009). Similarly, there may be differences in the contributions of the kicking actions. Thus, velocity and acceleration data need to be considered in combination with analysis of other kinematic data to enable valid conclusions to be drawn. The additional data required to supplement the CM velocity and CM acceleration data should include wrist X velocity and foot Y velocity as these can provide an indication of whether differences in force production by the hands and feet are likely. The average errors in wrist and ankle speeds and component velocities (Table 1) were less than 2% for the wrist and less than 6% for the ankles. This enables real differences between right and left wrist and ankle actions to be detected. For example, in the case of FC1, it can be seen that the patterns of wrist X velocity were very similar for right and left wrists in both recovery and during the pull (Figure 6). However, it is evident that the right wrist achieves a faster backward motion than the left during the latter part of the underwater action as the hand is pushing backwards prior to release (compare the speeds at 5 to 15% i.e. the push with the right wrist and 55 to 65% i.e. the push with the left wrist). This coincides with larger net CM X accelerations (Figure 5). Therefore, it is reasonable to conclude that the right hand applies more force due to its faster backward motion than the left during these equivalent periods of the stroke. Similarly, scrutiny of the right and left ankle motions in the Y direction for FC1 (Figure 7) reveals that the kick is more vigorous between the period approaching release of the right hand and catch of the left hand than the period approaching release of the left hand and catch of the right hand. Thus, it would appear that kicking helped to maintain force in the desired direction during the periods when the hands were not generating propulsive force. The average error% for the hand and foot velocity measures for FC2 and BS are also generally good. The worst of the %error scores was for the left foot speed of FC2. This was due to difficulty in seeing the foot marker due to turbulence. Despite the same stroke trials, FC1 showed smaller error than FC2 in the majority of variables. Given that the error was generally larger in FC2 than FC1 in the 15 to 85% period of the stroke cycle, rather than only at the ends of the data set, it is reasonable to conclude that the greater error in FC2 than FC1 was due primarily to the larger field of views in FC2 than in FC1. This made the image to be digitized smaller and the body landmarks harder to distinguish in FC2 than in FC1. This is supported by the fact that the errors in BS were less than in FC2 and comparable to FC1. This can be explained in terms of visibility of the body markers. The swimmer produced less turbulence by kicking in back crawl, and the hand path pattern in back crawl was less complex than that in front crawl, which made the markers more visible for many of the landmarks in back crawl than in front crawl. The comparison of the average error% across the period of the stroke cycle indicated that the start and finish of the data set, particularly the start, were slightly less reliable than the middle of the data set despite the additional points digitized and the reflection techniques employed to extend the data set. There was no obvious difference between FC2 or BS and FC1 due digitizing only five extras points rather than 10. Also, although generally larger than the middle section of the data set, the methods restrained the errors to levels that enable analysis and comparisons of patterns within and between swimmers and strokes. Nevertheless, analysts need to bear in mind that the errors at the endpoints are likely to be larger than in the central portion of the data set, particularly when deriving velocities and accelerations of swimmers with rapidly changing motion of the CM and the feet. Based on this study a number of recommendations emerge:
- Sampling at 25 Hz and filtering at 4Hz with a 4th order Butterworth digital filter can yield reliable data for analysis. There is no need to sample at higher rates. However, we recommend interpolating the data to enable the timing of events to be identified with minimal temporal error. On the basis of this study and those of other studies e.g. Vint and Hinrichs (1996) and Giakis et al (1998) it is recommended to digitize additional points. If the swimmer remains in view, 10 additional points would be preferable to five.
- The recommendation of digitizing 10 rather than five must also be considered in light of the need to maximize image size. The main difference in reliability between FC1 and FC2 in this study was due to the larger field of view of the cameras for FC2. Therefore, there needs to be a trade-off between the number of additional frames digitized and the field of view. However, a minimum of five additional points is recommended.
Insights into the production of forces and the interplay of propulsive and resistive forces can be obtained using an inverse dynamics based on 3D position data digitized from multiple camera views above and below the water surface. However, given the time consuming nature of the approach relative to other analysis methods, researchers must be sure that the time taken yields insights additional to those of other, less time consuming, approaches. The additional insights arise from deriving accurate velocities and accelerations of the actual CM of a swimmer rather than monitoring the motion of a fixed point of the body such as the hip joint. Further, the accuracy of derived velocity and acceleration of the CM can be assured by applying a full body model comprising 13 segments with segment masses and mass centre locations relative to digitized landmarks being personalised using the elliptical zone method (Deffeyes and Sanders, 2005; Jensen, 1978). In this study it was shown that the inverse dynamics process yields CM velocity and acceleration data that are sufficiently reliable to allow additional insights into swimming performance, for example, the existence of bilateral asymmetries in force production of right and left hands. Further, the ability to link the force profiles to swimming actions and technique is enhanced by having additional data such as wrist and foot velocities that can be obtained readily from the digitized data. To achieve good reliability at the ends of the data set when using a 4th Order Butterworth it is desirable to have 10 or more sample points beyond the period of interest. However, the number of additional sample points affects the time required for the swimmer to be in view of all cameras and therefore the field of view of the cameras. Given that the reliability of digitizing is affected by the image size a trade-off between the number of additional points and field of view of the cameras is necessary. When using the methods described in this paper sampling at 25Hz with between five and 10 additional frames prior to and after the period of interest, e.g. a full stroke cycle, is recommended.
ACKNOWLEDGEMENTS |
The authors declare that there is no conflict of interest with regard to this paper for any author. |
|
AUTHOR BIOGRAPHY |
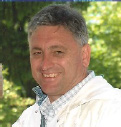 |
Ross H. Sanders |
Employment: Professor and Head of Exercise and Sport Science at The University of Sydney in the Faculty of Health Sciences. |
Degree: PhD |
Research interests: Bridge biomechanics and motor control and learning with specific emphasis on movement asymmetries and rhythms. |
E-mail: ross.sanders@sydney.edu.au |
|
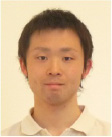 |
Tomohiro Gonjo |
Employment: PhD student at Centre for Aquatics Research and Education (CARE), The University of Edinburgh. |
Degree: MSc |
Research interests: Biophysical research of front crawl and back crawl in swimming. |
E-mail: T.Gonjo@sms.ed.ac.uk |
|
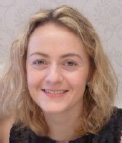 |
Carla B. McCabe |
Employment: Biomechanist at Ulster Sports Academy, University of Ulster. |
Degree: PhD |
Research interests: Biomechanical analysis of swimming and aquatic exercise. |
E-mail: c.mccabe@ulster.ac.uk |
|
|
|
REFERENCES |
 Barbosa T., Lima F., Portela A., Novais D., Machado L., Colaco P., Goncalves P., Fernandes R.J., Keskinen K.L., Vilas-Boas J.P. (2006) Relationships between energy cost, swimming velocity, and speed fluctuation in competitive swimming strokes. Portuguese Journal of Sports Sciences 6, 192-194.
|
 Bartlett R (1977) Introduction to Sports Biomechanics. London. E & FN Spon.
|
 Cappaert J.M., Pease D.L., Troup J.P. (1995) Three-dimensional analysis of the men’s 100-m freestyle during the 1992 Olympic Games. Journal of Applied Biomechanics 11, 103-112.
|
 Cappaert J.M., Pease D.L., Troup J.P., Troup J.P., Hollander A.P., Strasse D., Trappe S.W., Cappaert J.M., Trappe T.A. (1996) Biomechanics and Medicine in Swimming VII, Proceedings of the VII International Symposium on Biomechanics and Medicine in Swimming. Biomechanical highlights of world champion and Olympic swimmers. London. E & FN Spon.
|
 Choi D., Cole K.J., Goodpaster B.H., Fink W.J., Costill D.L. (1994) Effect of passive and active recovery on the resynthesis of muscle glycogen. Medicine and Science in Sports and Exercise 26, 992-996.
|
 Colman V., Persyn U., Ungerechts B.E., Keskinen K., Komi P., Hollander A.P. (1998) Biomechanics and Medicine in Swimming VIII, Proceedings of the VIII International Symposium on Biomechanics and Medicine in Swimming. A mass of water added to the swimmer’s mass to estimate the velocity in dolphin-like swimming below the water surface. Jyväskylä, Finland. University of Jyväskylä Press.
|
 Dodd S., Powers S.K., Callender T., Brooks E. (1984) Blood lactate disappearance at various intensities of recovery exercise. Journal of Applied Physiology 57, 1462-1465.
|
 D’Amico M., Ferrigno G. (1990) Technique for the evaluation of derivatives from noisy biomechanical displacement data using a model-based-bandwidth-selection procedure. Medical and Biological Engineering and Computing 28, 407-415.
|
 Figueiredo P., Vilas Boas J.P., Maia J., Gonçalves P., Fernandes R.J. (2009) Does the hip reflect the centre of mass swimming kinematics. International Journal of Sports Medicine 30, 779-781.
|
 Giakis G., Baltzopoulos V., Bartlett R.M. (1998) Improved extrapolation techniques in recursive digital filtering: A comparison of least squares and prediction. Journal of Biomechanics 31, 87-91.
|
 Hollander A.P., De Groot G., Van Ingen Schenau G.J. (1968) Measurement of active drag during front crawl swimming. Journal of Sport Science 4, 21-30.
|
 Jensen R.K. (1978) Estimation of the biomechanical properties of three body types using a photogrammetric method. Journal of Biomechanics 11, 349-358.
|
 Kadaba M.P., Ramakrishnan M.E., Wootten J., Gainey G., Gorton G., Cochran G.V.B. (1989) Repeatability of kinematic, kinetic, and electromyographic data in normal adult gait. Journal of Orthopaedic Research 7, 849-860.
|
 Kolmogorov S.V., Rumyantseva O.A., Gordon B.J., Cappaert J.M. (1997) Hydrodynamic characteristics of competitive swimmers of different genders and performance levels. Journal of Applied Biomechanics 13, 88-97.
|
 Mason B., Sacilotto G., Menzies T. (2011) Estimation of active drag using an assisted tow of higher than max swim velocity that allows fluctuating velocity and varying tow force. Abstracts of 29th International Society of Biomechanics in Sports, July, 2011, Porto, Portugal. Portuguese Journal of Sports Science 11, 327-330.
|
 McCabe C.B., Psycharakis S., Sanders R. (2011) Kinematic differences between front crawl sprint and distance swimmers at sprint pace. Journal of Sports Sciences 29, 115-123.
|
 Payton C.J., Bartlett R.M., Baltzopolous V., Coombs R. (1999) Upper extremity kinematics and bodyroll during preferred-side breathing and breath-holding front crawl swimming. Journal of Sports Sciences 17, 268-695.
|
 Payton C.J., Wilcox C. (2006) Intra-cyclic speed fluctuations of uni-lateral arm amputee front crawl swimmers. Portuguese Journal of Sport Sciences 6, 73-75.
|
 Psycharakis S., Sanders R.H. (2009) Validity of the use of a fixed point for intracycle velocity calculations in swimming. Journal of Science and Medicine in Sport 12, 262-265.
|
 Psycharakis S.G., Naemi R., Connaboy C, McCabe C., Sanders R.H. (2010) Three-dimensional analysis of intracycle velocity fluctuations in front crawl swimming. Scandinavian Journal of Medicine & Science in Sport 20, 128-135.
|
 Psycharakis S.G., Sanders R.H. (2008) Shoulder and hip roll changes during 200m front crawl swimming. Medicine and Science in Sports and Exercise 40, 2129-2136.
|
 Sanders R.H., Psycharakis S.G. (2009) Rolling rhythms in front crawl swimming with six-beat kick. Journal of Biomechanics 42, 273-279.
|
 Sanders R.H., Wilson B.D., Jensen R.K. (1991) Accuracy of derived ground reaction force curves for a rigid link human-body model. International Journal of Sport Biomechanics 7, 330-343.
|
 Togashi T., Nomura T., Maclaren D. (1992) Biomechanics and Medicine in Swimming – Swimming Science VI. A biomechanical analysis of the novice swimmer using the butterfly stroke. London. E&FN Spon.
|
 Toussaint H.M., Seifert L., Chollet D., Mujika I. (2011) World Book of Swimming. Biomechanics of drag and propulsion in front crawl swimming. New York. Nova Science Publishers Inc..
|
 Ungerechts B., Arellano R., Seifert L., Chollet D., Mujika I. (2011) World Book of Swimming. Hydrodynamics in Swimming. New York. Nova Science Publishers Inc..
|
 Vint P.F., Hinrichs R.N. (1996) Endpoint error in smoothing and differentiation raw kinematic data: An evaluation of four popular methods. Journal of Biomechanics 29, 1637-1642.
|
 Vorontsov A.R., Rumyantsev V.A., Knuttgen H.G., Dirix A., Renstrom P., Tittel K. (2000) Biomechanics in Sport: Encyclopaedia of Sports. Propulsive forces in swimming and Resistive forces in swimming. IOC and International Federation of Sports Medicine. Blackwell Publishing.
|
 Whittlesey S.N., Robertson D.G., Robertson D.G.E (2004) Research Methods in Biomechanics. Two dimensional inverse dynamics. Champaign, IL. Human Kinetics.
|
 Wilcock I.M., Cronin J.B., Hing W.A. (2006) Physiological response to water immersion: a method for sport recovery. Sports Medicine 36, 747-765.
|
 Winter D.A. (2009) Biomechanics and motor control of human movement. University of Waterloo, Ontario, Canada. John Wiley & Sons.
|
|
|
|
|
|
|