|
|
|
ABSTRACT |
Performance capacity in athletes depends on the ability to recover from past exercise. While evidence suggests that athletic performance decreases following (partial) sleep deprivation and increases following sleep extension, it is unclear to which extent natural variation in sleep impacts performance. Sleep quantity and, for the first time, sleep stages were assessed among 98 elite athletes on three non-consecutive nights within a 7-day monitoring period, along with performance tests that were taken on standardized times each following morning. Performance assessment included psychomotor performance (10-minute psychomotor vigilance task) and sport-specific tests of fine (e.g., accuracy) and gross motor skills (e.g., endurance, power). Mixed-effects models were employed to assess the effect of sleep quantity (total sleep time (TST), sleep onset latency (SOL), wake after sleep onset, sleep efficiency) and sleep stage duration (light, deep, REM) on performance. Average TST was 7:30 ± 1:05 hours, with a mean variation of 57 minutes across days. Longer TSTs were associated with faster reaction times (p = 0.04). Analyses indicated small and inconsistent effects of sleep quantity (TST, SOL) and sleep staging (light sleep) on gross motor performance, and no effects on fine motor skill performance.
Results indicate that natural variation in sleep quantity impacts psychomotor vigilance to a greater extent than athletic performance. Small or absent effects can be a consequence of the rather small variation in non-manipulated sleep. It is suggested that one night of compromised sleep may not be immediately problematic, but that more extreme sleep loss or accumulated sleep debt may have more severe consequences. |
Key words:
Athletic performance, sleep quantity, sleep architecture, psychomotor vigilance, elite sports
|
Key
Points
- Psychomotor performance is more strongly affected by sleep than athletic performance
- The effectof sleep on performance appears according to a dose-response relationship
- More extreme sleep loss or accumulated sleep debt over time may prove to be detrimental for performance and recovery.
|
The importance of sleep for athletic performance and recovery is widely acknowledged (Rae et al., 2017). Despite this importance, however, it appears that particularly elite athletes are facing compromised sleep quantity and quality (Lastella et al., 2015b; Leeder et al., 2012). Studies on partial and total sleep deprivation have highlighted the adverse effects of sleep loss on athletic performance (see: Fullagar et al., 2015 for a review of this literature), while other studies show that sleep extension may actually benefit performance (Mah et al., 2011; Schwartz and Simon, 2015). Yet, the minimal magnitude of sleep deprivation or extension required to impact athletic performance is unknown. Routinely, extreme changes in sleep duration (± 4 hours) are fairly rare among elite athletes. Minor variations, however, are more frequently encountered. For example, literature indicates that unfavourable training schedules (Sargent et al., 2014), competition times (Lastella et al., 2015a), and (inter-meridian) travel (Manfredini et al., 1998), may all cause small, but significant reductions in sleep quantity, especially when compared to sleep on rest days (Sargent et al., 2014). Against this background, the current study aimed to assess the extent to which natural variations in sleep are reflected in the performance of elite athletes. Regarding athletic performance, a classical distinction is often made between ‘fine motor skills’ and ‘gross motor skills’ (Davis et al., 2000; Fullagar et al., 2015). Fine motor skills are skills that incorporate intricate, precise movements, which use small muscle groups and involve high levels of hand-eye coordination (e.g., golf putting). In contrast, gross motor skills are skills that incorporate less precise, whole-body movement, which use large muscle groups and involve lower levels of hand-eye coordination (e.g., jumping, running, cycling; Davis et al., 2000). As such, an important aspect that distinguishes fine motor skills and gross motor skills is the extent to which they rely on cognitive functions to accurately coordinate the movement. Given the well-documented effect of sleep on cognitive functioning (e.g., reduced behavioral alertness and more cognitive errors after sleep loss; Jarraya et al., 2013; Van Dongen et al., 2003), it is often proposed that fine motor skills are more strongly affected by sleep loss than gross motor skills (Fullagar et al., 2015). Yet, empirical research is scarce, findings remain equivocal, and the effect of sleep on athletic performance is still poorly understood (Fullagar et al., 2015). To produce more insight in this matter, the current study tested the impact of sleep on elite athletes’ psychomotor performance as well as their performance on sport-specific fine and gross motor skills. Furthermore, while most studies have focused on implications of reduced sleep duration (i.e., sleeping fewer hours), to our knowledge, no studies have investigated how natural between-days variations in sleep staging (e.g., differences in the proportion of light, deep or REM sleep) may affect athletic performance. Still, specific recovery functions associated with different sleep stages make it likely that (natural) variation in the absolute time spent in a certain sleep stage might influence performance. For example, deep sleep (also referred to as Slow Wave Sleep or N3) has been associated with the release of growth hormone and, hence, is believed to contribute specifically to muscle restoration and physiological recovery (Shapiro et al., 1981). With regard to psychomotor performance, studies indicate that sheer reductions in sleep quantity rather than variations in the time spent in a certain sleep stage (e.g., more time spent in deep sleep) are responsible for the observed effects (e.g., Edinger et al., 2000; Tilley and Wilkinson, 1984). With regard to athletic performance, however, such information is currently lacking. Therefore, in assessing the impact of sleep on psychomotor and athletic performance, the current study assessed effects of (changes in) sleep quantity as well as sleep staging (i.e., absolute time spent in light sleep, deep sleep, and REM sleep). In view of the considerations above, the aim of the current study was to investigate the effect of natural between-days variation in sleep quantity and the absolute time spent in different sleep stages on (sport-specific) performance in elite athletes. In particular, effects of sleep were assessed on a general measure of, i) psychomotor performance; and sport-specific measures of, ii) fine motor skill performance, and iii) gross motor skill performance. To provide a robust answer, objective measures of sleep and performance were taken on three non-consecutive occasions within a 7-day monitoring period, on uniform times, among a large cohort of elite athletes (i.e., within-subject, repeated measures design). The overarching hypothesis was that variations in sleep (i.e., increase or decrease in sleep from one day to the next) would impact psychomotor performance more strongly than sport-specific performance and that fine motor skill performance would be more impacted than gross motor skill performance (Fullagar et al., 2015). We had no a priori expectation with regard to which distinct sleep characteristic (i.e., sleep quantity or sleep staging) would impact performance most.
ParticipantsAthletes were recruited via the Netherlands Olympic Committee*Netherlands Sport Federation (NOC*NSF) or via the head coaches of the respective Dutch sport associations. In total 98 elite athletes (56 female) participated. All participants were part of the national (youth) selection in their respective sport and competed at the highest national and international (youth) level. Athletes were aged 18.8 ± 3.0 (range 15-32) years, had an average Body Mass Index of 21.3 ± 1.6 kg/m2, had practiced their sport on average for 10 ± 3.5 years, and spent on average 19.3 ± 5.1 hours per week on training and competition. Athletes competed in different individual and team sports (Table 1). Athletes were screened for overall sleep quality ((PSQI; Buysse et al., 1989; 4.61 ± 2.04, M ± SD)) and subjective sleep complaints (HSDQ; Kerkhof et al., 2013; 1.64 ± 0.35, M ± SD) 1.64 ± 0.35, M ± SD). A detailed description of subjective and objective sleep estimates of the current sample can be found elsewhere (Knufinke et al., 2018a; Knufinke et al., 2018b). No athletes were excluded based on their sleep history. Ethical approval was obtained from the faculty’s ethical committee and all participants or responsible guardians signed informed consent [ECSW2013-1612-170].
Measures and proceduresAs part of a larger project assessing sleep among Dutch elite athletes, sleep was assessed for seven consecutive nights. Within this period, measures of (sport-specific) performance were taken on three occasions, typically scheduled 48 hours apart (i.e., on day 1, 4, and 7). In specific cases, adjustments needed to be made in scheduling the performance tests to avoid interference with existing training schedules: female road cyclists (n = 9): performance tests on day 1, 6, 7; soccer players (n = 17): performance tests on day 1, 3, 6; volleyball players (n = 30): performance tests on day 1, 5, 8). Before starting the initial study protocol, athletes underwent three nights of habituation to sleep-wake assessment and one performance test practice session, to become familiar with the performance tests and to get used to the sleep monitoring. All athletes slept at home or in a (training) environment that was highly familiar to them and sleep-wake schedules were habitual (self-chosen). In all cases, the monitoring period was free from competition, with the exception of exhibition matches. Handball and volleyball players, triathletes and mountain bikers were monitored during a training period at their home-base. Road cyclists and soccer players were monitored during one of their annual training camps abroad. The female cyclists (n = 9) crossed six time-zones in a west-ward inter-meridian travel. For those athletes, data collection started after 6 days to allow for circadian adaptation to the new time-zone. Sleep quantity and sleep stages were assessed by means of wrist-actigraphy and one-channel EEG sensors, respectively. In addition, a sleep diary (Expanded Consensus Sleep Diary; Carney et al., 2012) was kept to facilitate analysis of the actigraphy data and to monitor background variables related to the athletes’ sleep hygiene (data reported elsewhere: Knufinke et al., 2018a). Performance assessment included tests of (1) psychomotor performance, (2) fine motor performance and (3) gross motor performance. Psychomotor performance was assessed by means of a standardized 10-minute psychomotor vigilance task (PVT; Dinges and Powell, 1985). In consultation with the athletes’ coaches, fine and gross motor skill performance were assessed sport-specifically (Table 1). To allow for comparison across sports, test outcomes for fine and gross motor skill performance were transformed into norm scores (see ‘Data Processing’). In case of multiple performance tests, the test sequence was standardized. All performance measures were taken in the morning on uniform time points, following a standardized (sport-specific) warm-up. Performance tests were conducted between 6 AM and 10 AM, depending on sport and team, but at standardized times within individuals.
Sleep quantitySleep quantity was assessed by means of an actigraph that was continuously worn around the non-dominant wrist and only detached during training or when being in contact with water (Actiwatch 2, Philips Respironics, Murrysville, USA). The Actiwatch has been validated against polysomnography (e.g., Weiss et al., 2010). Motion was sampled at 32Hz, averaged and stored in 60 second bins. Parameters of interest were total sleep time (TST; h:min), sleep onset latency (SOL; min), wake after sleep onset (WASO; min), and sleep efficiency (SE; %).
Sleep stagesSleep stages were recorded by means of a wireless, self-logging headband sensor (Wireless System, WS; Zeo Inc., Newton, USA). The Wireless System was validated for sleep registration in healthy adults and performs automatic classification of sleep stages based on recordings of a single bi-polar channel, which was located at the forehead (EEG position approximately at Fp1-Fp2 with a ground at Fpz) and integrated into an elastic headband (Shambroom et al., 2012). Based on analysis of 30-second epochs the WS distinguishes between episodes of wakefulness, light sleep (comparable to N1/N2), deep sleep (comparable to N3) and Rapid Eye Movement (REM) sleep. As reported by Shambroom et al. (2012), agreement between WS and Polysomnography (PSG) is 98.5% for scoring sleep / wakefulness and 83.6% for sleep stage classification. The Wireless System was attached just before lights-off and removed following lights-on. Parameters of interest were absolute time (h:min) spent in light sleep, deep sleep, and REM sleep.
Performance assessmentApart from assessing effects of sleep on psychomotor performance, the aim of the current study was to arrive at a broad but yet representative indication of the effects of sleep on fine and gross motor skill performance across different sports. For this reason, performance outcomes on sport-specific performance tests were standardized and pooled to reflect separate scores for fine and gross motor skill performance (see ‘Data Processing’).
Psychomotor performancePsychomotor performance was assessed by means of a custom made, 10-minute PVT (Dinges and Powell, 1985). Using a standardized computer setup, participants were instructed to press a button as fast as possible upon appearance of a red target stimulus on an otherwise black screen. Stimulus appearance was randomized with inter-stimulus intervals ranging between 2 and 10 seconds. Reaction time delay in milliseconds (ms) was indicated by a scrolling counter and served as immediate feedback upon response. Reaction times below the anticipation criterion of ≤ 100 ms were excluded from further analysis. Reactions without a stimulus were considered as false alarms (errors of commission) and a lapse was operationalized as a reaction time ≥ 500 ms. The following outcome metrics were included: (a) mean reaction time, and (b) error rate (sum from the number of false alarms and the number of lapses) (Van Dongen et al., 2012). The PVT was usually assessed before the physical performance tests, except for handball- and volleyball players, who executed multiple performance tests. To arrive at a time efficient assessment protocol, those individuals received a predefined testing sequence that was standardized within individuals, but varied between individuals.
Fine motor skill performanceFine motor skills were operationalized as skills that incorporate intricate, precise movements, which use small muscle groups and generally involve high levels of hand-eye coordination (Davis et al., 2000). Assessment of fine motor skills was sport-specific and, depending on the sport, comprised shooting accuracy, dribbling, or a technical skills-track. Handball players and volleyball players performed a shooting accuracy test (see Mah et al., 2011; Reyner and Horne, 2013 for a similar approach in testing effects of sleep on shot accuracy in basketball and tennis players, respectively), soccer players performed a dribble test (e.g., Reilly et al., 2007), and mountain bikers performed a standardized technical skills-track, (e.g., Gregory et al., 2007). Given the nature of their sport (and in consultation with the coaches), road cyclists (N = 26) and triathletes (N = 8) did not perform a test of fine motor skill performance. A detailed description of all fine motor skill performance tests is included below. Shooting accuracy. Handball- and Volleyball players performed a shooting accuracy test. Handball players (n = 13) performed jump shots in the direction of a target that was located in the top left and right corner of the goal. The target was a metal frame (50 x 50 cm), with a 5cm wide framework and an opening of 40 x 40 cm. Shots were taken from the 9 meter line. Players took a regular 3-step run-up before executing the jump shot. To mimic actual performance situations, two air-bodies were placed in the center of the 7 meter line. Scores ranged from 1-3, depending on whether the ball was thrown through the target opening (3 points), hit its framework (2 points), or missed the target (1 point). The average score over 10 trials (5 aims towards the right side of the goal and 5 aims towards the left side of the goal) was taken as outcome measure. Volleyball players (n = 30) performed smashes in the direction of a target area that was located in the right and left outside corners of the opponents back zone, in one meter distance from the attack-line (3-meter line). The target area (180 x 180 cm) was divided into three rectangular parts of the same size (180 cm long x 60 cm wide). Smashes were taken from the position of the ‘middle blocker’, just behind the net. Standardized set-ups were given by the coach who also threw the ball manually. Scores ranged from 1-4, depending on whether the volleyball hit the target closest to the side line (4 points), hit the central-part of the target (3 points), hit the most inside part of the target (2 points), or missed the target (1 point). The average score over 10 trials (5 aims towards the right side of the court and 5 aims towards the left side of the court) was taken as outcome measure. Dribbling. Soccer players (n = 17) performed a dribble test in which they had to dribble the ball as rapidly as possible through a slalom course that consisted of six cones set 1.5 m apart for a total of 10.5m from start to finish (e.g., Reilly et al., 2007). Start and end of the slalom course were indicated by timing gates (Smart Speed, Fusion Sport, Queensland, Australia). Each trial was started from a position 50 cm in front of the start gate. The average time (in seconds) over two trials was taken as outcome measure. Technical skills-track. Mountain bikers (n = 4) performed a standardized indoor technical skills-track, which they had to complete as fast as possible (e.g., Gregory et al., 2007). The track entailed a pumptrack (2.5 rounds), ramps, sharp turns, jumps, trunk overpasses and balancing. The task was timed using timing gates (Smart Speed, Fusion Sport, Queensland, Australia). The average time (in seconds) over three trials was taken as outcome measure.
Gross motor skill performanceGross motor skills were operationalized as skills that involve large muscle movements, which are not very precise and include many fundamental movement skills (e.g., jumping, running, cycling; Davis et al., 2000). Assessment of gross motor skills was sport-specific and, depending on the sport, comprised vertical jumps, maximal sprints, or a constant power test. Handball- and volleyball players performed a vertical jump test (Lucas et al., 2009) and – for the handball players only – a maximal 20-meter sprint test (e.g., Skein et al., 2011), and road cyclists, mountain bikers and triathletes performed a constant power test (e.g., Mougin et al., 1988). In consultation with the coaches, soccer players (n = 17) did not perform a test of gross motor skill performance. A detailed description of all gross motor skill performance tests is included below. Vertical jumps. Handball players (n = 13) and volleyball players (n = 30) performed a vertical jump test in which they executed three trials of the counter movement jump (CMJ) and spike jump (SJ) (Lucas et al., 2009). The highest relative jump height (i.e., jump height minus standing reach height) was used as outcome measure. Jump height and standing reach height were measured using Vertec (Yardstick, Swift Performance Equipment, Lismore, Australia). Maximal sprints. Handball players (n = 13) performed a maximal 20-meter sprint test. Start and end of the sprint were indicated by timing gates (Smart Speed, Fusion Sport, Queensland, Australia). Each trial was started from a position 50 cm in front of the start gate. The average time (in seconds) over two trials was taken as outcome measure (e.g., Skein et al., 2011). Constant power tests. Road cyclists (n = 26), mountain bikers (n = 4) and triathletes (n = 8) performed a constant power test (Mougin et al., 1988). Road cyclists and mountain bikers performed the constant power test by cycling for 10 minutes at a fixed (individualized) power of 4 watt/kg. After a standardized warm-up, the 10-minutes started as soon as athletes arrived at their pre-defined power output. The athletes were instructed (and followed by car) to cycle the same route on an individually set power, and at a self-preferred cadence. The average cadence at which participants cycled was 83 rpm, with a within-subject variation of 3.86 rpm (i.e., 3.24 %), indicating that across tests participants adopted a constant pace. Average heart rate during the constant power test was obtained with the Garmin (or other) chest-worn heartrate sensors. Triathletes performed the constant power test by swimming over a 200-meter extent at constant (individually set) velocity. Velocity was monitored every 25m and communicated by the coach, a method the athletes were familiar with. Heart rate was assessed using waterproof chest-sensors (version T31) by Polar (version v800) that had a transmitter integrated in a back pocket of a small vest. The transmitter send the heart rate data to a laptop located next to the pool were the test intervals were manually indicated by event markers (Hosand GT aqua system).
Data processingSleep and performance data were processed as described below. Sleep quantity. Actigraphy data was analysed using Respironics Actiware 5 (Philips Respironics, Murrysville, USA), following the guidelines by the Society of Behavioural Sleep Medicine (SBSM) as delineated by Ancoli-Israel et al. (2015). Data was visually inspected and excluded when activity counts and light values indicated detachment of the sensor. In all other cases, rest intervals were manually set when (i) event markers identified bed- and rise time, or – in case of missing event markers – when (ii) light and activity was absent. If light and activity values were ambiguous, (iii) WS data and diary entries were used to set rest intervals. The default setting (10-minutes immobility parameter) was used to identify sleep onset and sleep offset. Following Sargent et al. (2016), episodes of sleep / wakefulness were identified using a high sleep-wake threshold (i.e., AW>80; epochs are scored as wake if activity counts were above 80). TST was derived from the interval type “rest”. SOL, WASO and SE were derived from the interval type “sleep”. Sleep stages. Sleep stages were automatically classified by the WS. Consecutively, data was processed as follows: First, to determine lights-off and lights-on times, actigraphy and diary entries were taken as a reference. Second, in order to match time in bed (TIB) values with actigraphy and diary reports, missing epochs were manually added at the beginning and end of a night and classified as “undefined” when necessary. Third, nights with more than 15% “undefined-” epochs were excluded from further analysis, which was the case for 29.9% of the recordings (e.g., losing the headband, equipment mal-functioning). Consequently, from all 294 recordings / nights, 206 nights (70.1%) were maintained for statistical analysis. Little’s MCAR test revealed that data was missing at random (χ2(9) = 16.666, p = 0.054), indicating that available data (i.e., 206 of all 294 possible recordings) may be taken as representative. Fourth, sleep onset was operationalized as the first of three consecutive epochs of “light sleep” (Moser et al., 2009). Compared to polysomnography, the WS overestimates REM at the cost of “wake” (Shambroom et al., 2012). Therefore, all “undefined” or “REM” epochs that preceded sleep onset were manually scored as “wake”. Finally, based on the normative range of REM sleep onset (49.5-278.5 min) provided by Mitterling et al. (2014), “REM” epochs within the first 30 minutes of the night and without succeeding “deep sleep” were also manually scored as “wake”. Performance. Psychomotor performance (alertness) was assessed in standardized fashion across sports, using the PVT. Outcome measures were reaction time and error rate. Fine and gross motor skill-performance were assessed sport-specifically. To allow comparison across sports, performance outcomes were standardized by means of group-mean-centering, according to the following formula: =(X - µteam )/(σteam) . Standardized scores were then rescaled if needed such that high scores always reflected better performance. Whenever the assessment of fine or gross motor skill-performance consisted of more than one test (i.e., handball and volleyball players only), scores of different tests were averaged so as to express a single outcome measure for each type of performance.
Statistical analysisDescriptive statistics were performed for sleep quantity, sleep stages and performance outcomes. To investigate how natural variation in sleep affects subsequent performance, linear mixed-effects models were employed. Linear mixed-effects models are an extension to linear regression that take the nested structure of the data into account (i.e., repeated measurements within individuals). We used the lmer function of the lme4 package (version 1.1.-1) in R (R Core Team, 2015). All performance categories were analysed in separate models (psychomotor performance [mean reaction time and error rate]; fine motor and gross motor skill performance). To optimize power, sleep quantity and sleep stage effects were tested in separate models, resulting in a total of 8 linear mixed-effects models. Each model included a fixed intercept and fixed effects for gender (with contrast set as 1 for male and -1 for female), age, time awake (time between awakening and performance assessment), and day. On a side note, one might argue that, apart from these variables, sleep and performance analyses may also be controlled for alcohol intake, caffeine intake and the use of sleep aids. For reasons of model convergence, this was not done. During the study, substance use was monitored on a daily basis (data reported elsewhere; Knufinke et al., 2018a), which generally indicated extremely low occurrence and no or minimal correlations with sleep. Hardly any alcohol was consumed in the evening (average occurrence: 2% of nights), standardized testing procedures prevented variation in caffeine intake in the morning, and only 1 out of 98 participants consistently took sleep medication (i.e., a low dose of melatonin, consistently taken throughout the measurement period). Based on these numbers, and considering that all analyses considered within-person effects, it is assumed that substance use had no impact on our results. In the “sleep stage model” minutes spent in light sleep, deep sleep and REM sleep (all from the WS) were respectively included in the same model. In the “sleep quantity model”, TST, SOL, WASO and SE (all from the Actiwatch) were included in the same model. SOL was log10 transformed to correct for the non-normal distribution of SOLs. All variables were person-mean-centered, which means that for each variable we first took the mean value of all 3 measurements within each individual and then subtracted it from that individual’s respective day-values, resulting in 3 new person-mean-centered scores. The average of each individual’s person-mean centered scores equals 0. A maximal random-effects structure was used by including a per-participant random intercept as well as per-participant random slopes for time awake and the respective model’s corresponding sleep parameters. For convergence reasons, all possible random correlation terms among the random effects were excluded. P-values were determined using the function “mixed” from the package afex using type 3 tests and the parametric bootstrap method (with 10000 simulations), which in turn calls the function PBmodcomp from the package pbkrtest (version 0.4.6). Confidence intervals were calculated using parametric bootstrapping as implemented in lme4's bootMer function, with 10000 simulations and by deriving 95% confidence intervals using the function boot.ci of the package boot (version 1.3.17).
Means and standard deviations for all sleep parameters and performance outcomes based on the three nights / days are displayed in Table 1 and Table 2. A more detailed 7-day sleep profile including sleep hygiene aspects of all participating athletes was previously published in (Knufinke et al., 2018a; 2018b). The three-day average total sleep time was 7:30 ± 1:05 hours. Across the three measurement days, within-individual variation in total sleep time ranged from 4 minutes for the most consistent athlete to 149 minutes for the most variable athlete (variance between days). Across all athletes, the average between-days variation in total sleep time was 57.14 ± 38.48 minutes. 36 out of 98 athletes had a variation in total sleep time of maximal ≥ 60 minutes across all three nights.
Effect of sleep quantity and sleep stages on psychomotor performanceReaction time. With regard to the effect of sleep quantity on mean reaction time, linear mixed-effects models revealed a significant effect of total sleep time (B = -0.070, se = 0.04, 95% CI [-0.142, 0.001], p = 0.04), indicating an improvement in reaction time following nights in which an athlete slept longer than his/her own average (TST = 0, Figure 1). To indicate the extent to which very slow and/or very fast reaction times influenced the observed effect of TST on reaction time, a post-hoc analysis was performed using Basner and Dinges (2011) measure of ‘response speed’ (i.e., response speed = 1000/RT). As outlined by Basner and Dinges (2011), transforming reaction times to response speed reduces the influence of extreme values. As appeared from the analysis, TST did not significantly affect response speed (with, B = 0.001, se = 0.001, 95% CI [-0.001, 0.002], p = 0.43), thereby indicating that the observed effect of TST on reaction time was at least partly dependent on large variability in reaction times with increasing or decreasing TST. The remaining sleep quantity parameters did not reach significance, with sleep onset latency (B = -3.393, se = 3.19, 95% CI [-9.758, 2.977], p = 0.29), wake after sleep onset (B =0.100, se = 0.13, 95% CI [-0.151, 0.356], p = 0.42), and sleep efficiency (B = 0.224, se = 0.42, 95% CI [-0.610, 1.060], p = 0.60). Concerning the effect of sleep stages on reaction time, no significant associations were observed. That is, reaction time was not significantly affected by the time spent in light sleep (B = -0.068, se = 0.05, 95% CI [-0.166, 0.030], p = 0.15), deep sleep (B = -0.03, se = 0.10, 95% CI [-0.244, 0.182], p = 0.77), or REM sleep (B = -0.090, se = 0.07, 95% CI [-0.235, 0.055], p = 0.21). Error rate. With regard to the effect of sleep quantity on error rate, linear mixed-effects models revealed no significant association, with total sleep time (B = -0.005, se = 0.01, 95% CI [-0.020, 0.009], p = 0.44), sleep onset latency (B = -0.461, se = 0.60, 95% CI [-1.666, 0.728], p = 0.45), wake after sleep onset (B = -0.011, se = 0.03, 95% CI [-0.061, 0.039], p = 0.67), and sleep efficiency (B = 0.041, se = 0.07, 95% CI [-0.104, 0.184], p = 0.59). Similar to sleep quantity, no significant associations could be established between sleep stages and error rate. That is, error rate was not significantly affected by variation in light sleep (B = -0.000, se = 0.01, 95% CI [-0.019, 0.018], p = 0.96), deep sleep (B = 0.25, se = 0.02, 95% CI [-0.010, 0.060], p = 0.12), or REM sleep (B = -0.019, se = 0.01, 95% CI [-0.041, 0.004], p = 0.09).
Effect of sleep quantity and sleep stages on fine motor skill performanceRegarding the effect of sleep quantity on fine motor skill performance, linear mixed-effects models revealed no significant associations with total sleep time (B = 0.005, se = 0.00, 95% CI [-0.001, 0.010], p = 0.10), sleep onset latency (B = 0.185, se = 0.00, 95% CI [-0.225, 0.593], p = 0.38), wake after sleep onset (B = -0.001, se = 0.01, 95% CI [-0.022, 0.020], p = 0.91), or sleep efficiency (B = -0.021 (se = 0.03), 95% CI [-0.089, 0.047], p = 0.52). Similar to sleep quantity, no significant associations could be established between sleep stages and fine motor skill performance. That is, fine motor skills were not significantly affected by variation in light sleep (B = 0.001, se = 0.00, 95% CI [-0.008, 0.010], p = 0.74), deep sleep (B = .014, se = 0.01, 95% CI [-0.005, 0.033], p = 0.18), or REM sleep (B = 0.001, se = 0.01, 95% CI [-0.011, 0.013], p = 0.86).
Effect of sleep quantity and sleep stages on gross motor skill performanceRegarding the effect of sleep quantity on gross motor skill performance linear mixed-effects models revealed a significant effect of sleep onset latency on gross motor skill performance (B = -0.343, se = 0.16, 95% CI [-0.670, -0.011], p = 0.03), suggesting shorter sleep onset latencies to be associated with improved gross motor skill performance. In addition, a small but significant effect of total sleep time on gross motor skill performance (B = -0.005, se = 0.02, 95% CI [-0.009, -0.001], p = 0.008) indicated that shorter total sleep time was associated with improved gross motor skill performance. Wake after sleep onset (B = -0.009, se = 0.01, 95% CI [-0.024, 0.005], p = 0.18) and sleep efficiency (B = -0.030, se = 0.02, 95% CI [-0.071, 0.012], p = 0.14) did not show significant associations with gross motor skill performance. Concerning the effect of sleep stages on gross motor skills, a small but significant effect of light sleep on gross motor skill performance (B = -0.007, se = 0.00, 95% CI [-0.012, 0.001], p = 0.01) indicated that obtaining fewer minutes of light sleep was associated with improved gross motor performance. Deep sleep (B = -0.005, se = 0.01, 95% CI [-0.019, 0.008], p = 0.41), and REM sleep (B = 0.005, se = 0.00, 95% CI [-0.004, 0.014], p = 0.28) did not show significant associations with gross motor skill performance.
The current study investigated to which extent natural between-days variation in sleep is reflected in the psychomotor and sport-specific performance of elite athletes. Objective measures of sleep quantity, sleep stages and subsequent (sport-specific) performances were taken on three non-consecutive occasions within a 7-day monitoring period, on uniform times, among a large cohort of elite athletes. Our results show that small changes in sleep quantity (total sleep time) were reflected in small but significant changes in psychomotor performance (reaction time). In addition, small but inconsistent effects of sleep quantity and sleep staging on gross motor skill performance were observed. Fine motor skill performance remained irresponsive to changes in sleep. Overall, athletes showed adequate though slightly fragmented sleep, with approximately 8 hours of total sleep time and a healthy distribution of sleep stages across the measurement period of 7-days (see: Knufinke et al., 2018b, for a more detailed report of sleep characteristics in the same population). With regard to psychomotor performance, we found a significant negative association between total sleep time and reaction time, with longer sleep duration being related to faster responses on the psychomotor vigilance test. As appears from Figure 1, athletes’ mean reaction time increased or decreased with approximately 5ms when they slept about 60 minutes shorter or longer than their individual average, respectively. The fact that this effect is relatively small is in line with the literature (Jewett et al., 1999) and may be explained by the observation that within-subject variation in total sleep time across the three measurement occasions was also small (i.e., mean ∆TST = 57.14 minutes). Still, in elite sports, small effects can be critical. Moreover, and in line with the dose-relationship between sleep duration and psychomotor vigilance, the current data suggests that when incidental sleep loss is more extreme (e.g., as might occur when approaching important competitions (Lastella et al., 2015a)), or continues over a number of days, effects may be expected to be substantially larger (Van Dongen et al., 2003). In this sense, the current study underlines that sleep loss negatively affects psychomotor vigilance but at the same time, highlights the potential benefits of sleep extension (Mah et al., 2011). With regard to our measures of sport-specific fine and gross motor skill, results indicated very few significant effects. While in part this confirms the idea that sleep has a stronger effect on psychomotor performance than on athletic performance, we had expected to substantiate this perspective by showing that performance on fine motor skills would be more strongly affected by sleep than performance on gross motor skills (Fullagar et al., 2015). However, neither sleep quantity nor sleep staging appeared to have any effect on our measure of fine motor skill. Again, a likely explanation for the absence of significant effects is that within-subject variation in sleep across the three measurement occasions was simply too small to have a measureable impact on performance. At the same time, however, we did find a significant negative association between sleep onset latency and performance on gross motor skills (i.e., worse performance with longer sleep onset latencies), as well as improved gross motor skill performance as a function of less total sleep time and less light sleep. It should be noted, however, that the observed effects were generally small. Considering this, the general pattern of results appears to indicate that minor variations in sleep quantity and sleep staging, as in the current study, are unlikely to have a strong influence on athletic performance. Future research, involving more extreme variations in sleep quantity and sleep staging is required to substantiate this finding and dissociate between effects on fine and gross motor skills. Apart from the naturally minor between-days variations in sleep, there are a number of potential limitations that need to be taken into account when interpreting the current findings. The current field setting entailed that, to conveniently measure athletes’ sleep, we had to rely on wristwatch actigraphy (sleep quantity) and wireless one-channel EEG (sleep stages; see Methods). While both systems are often used in field research (Knufinke et al., 2018b; Leeder et al., 2012) and show adequate agreement with polysomnography (which is considered the gold standard) (Shambroom et al., 2012; van de Water et al., 2011), absolute values may deviate and, for example, precise measurement of actigraphy-based sleep onset latency is prone to a systematic error (Paquet et al., 2007). The observed effect of sleep onset latency on gross motor skill performance should therefore be interpreted with caution. Also, in attempting to make a representative (sport-specific) distinction between effects of sleep on fine and gross motor skill performance across different sports, we chose to standardize and pool performance outcomes on different tests. While this was done according to a strictly formalized procedure, standardizing and pooling data across tests might have introduced noise and, hence, reduced measurement sensitivity. Similarly, arguments may be raised to suggest that the current performance categories may be overly broad and that pooling across more specific performance categories may be more useful. Future studies are therefore advised to incorporate more standardized tests of fine and gross motor skill performance, potentially also in a more homogeneous group of athletes. Moreover, it should be noted that in the current study, performance tests were always taken on standardized times in the morning. While this procedure effectively excluded potential time-of-day effects, literature suggests that effects of sleep loss on athletic performance may be slightly more pronounced when performance tests are conducted in the evening (i.e., with sustained wakefulness; Meijman et al., 1990). Finally, sampling from a limited pool of elite athletes, age ranges may vary more than what is desired. In the current study, assessing within-subject effects and including age as a covariate in the analyses, strongly reduced the potential influence of age on sleep and performance. To fully exclude age effects, also in case of non-linear effects, future studies are advised to recruit athletes from a smaller age-range.
ConclusionsThe current study showed that even minor (between-days) variations in sleep can significantly affect elite athletes’ psychomotor performance; with reduced total sleep time being associated with reduced psychomotor vigilance on the following morning. At the same time, fine motor skill performance and to a large extent also gross motor skill performance appeared largely irresponsive to minor variations in sleep. Taken together, results indicate that psychomotor performance is more strongly affected by sleep than athletic performance (Fullagar et al., 2015) and highlight a dose-response relationship (Jewett et al., 1999). It is thereby suggested that one night of minor sleep loss (i.e., < 1 hour) may not be immediately problematic, but that more extreme sleep loss or accumulated sleep debt over time may prove to be detrimental for performance and recovery.
ACKNOWLEDGEMENTS |
No authors declare conflicts of interest. The study complied with the laws of the country of the authors’ affiliation. |
|
AUTHOR BIOGRAPHY |
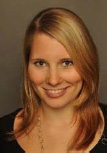 |
Melanie Knufinke |
Employment: A PhD-candidate at the Behavioural Science Institute, Radboud University in the Netherlands. |
Degree: MSc |
Research interests: How sleep optimization can be used to improve health and performance in elite athletes. |
E-mail: M.Knufinke@psych.ru.nl |
|
 |
Arne Nieuwenhuys |
Employment: Lecturer in Sport and Exercise Psychology at the University of Auckland in New Zealand. |
Degree: PhD |
Research interests: How psychophysiological states such as sleep, fatigue and anxiety affect human motor control and performance. |
E-mail: |
|
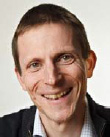 |
Kamiel Maase |
Employment: As performance manager research & innovation for TeamNL |
Degree: MSc |
Research interests: A former distance runner holding several national records and has competed in the 2000, 2004 and 2008 Olympics. |
E-mail: |
|
 |
Maarten H. Moen |
Employment: The Dutch NOC*NSF, the Dutch Olympic movement. |
Degree: MD, PhD |
Research interests: Sleep in elite athletes. |
E-mail: |
|
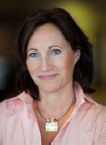 |
Sabine A.E. Geurts |
Employment: A full professor of work- and organizational psychology at the Behavioural Science Institute at the Radboud University, Nijmegen (Netherlands). |
Degree: PhD |
Research interests: Work stress, recovery, sleep, working time arrangements, health behaviour and physical activity. |
E-mail: |
|
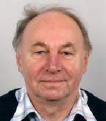 |
Anton M.L. Coenen |
Employment: Emeritus professor at the Radboud |
Degree: PhD |
Research interests: Sleep sensory stimuli and brain. |
E-mail: |
|
 |
Michiel A.J. Kompier |
Employment: Professor of Work and Organizational Psychology at Radboud University. |
Degree: PhD |
Research interests: Stress, motivation and performance in work and in sports. |
E-mail: |
|
|
|
REFERENCES |
 Ancoli-Israel S., Martin J.L., Blackwell T., Buenaver L., Liu L., Meltzer L.J., Sadeh A., Spira A.P., Taylor D.J. (2015) The SBSM guide to actigraphy monitoring: clinical and research applications. Behavioral Sleep Medicine 13, S4-S38.
|
 Basner M., Dinges D.F. (2011) Maximizing sensitivity of the psychomotor vigilance test (PVT) to sleep loss. Sleep 34, 581-591.
|
 Buysse D.J., Reynolds C.F., Monk T.H., Berman S.R., Kupfer D.J. (1989) The Pittsburgh Sleep Quality Index: a new instrument for psychiatric practice and research. Psychiatry Research 28, 193-213.
|
 Carney C.E., Buysse D.J., Ancoli-Israel S., Edinger J.D., Krystal A.D., Lichstein K.L., Morin C.M. (2012) The Consensus Sleep Diary: standardizing prospective sleep self-monitoring. Sleep 35, 287-302.
|
 Davis, R., Philips, R., Roscoe, J. and Roscoe, D. (2000) The nature and
classification of skill. In: Physical education and study of sports.
4th edition. London UK: Harcourt Publishers Ltd. 284-285.
|
 Dinges D.F., Powell J.W. (1985) Microcomputer analyses of performance on a portable, simple visual RT task during sustained operations. Behavior Research Methods Instruments & Computers 17, 652-655.
|
 Edinger J.D., Glenn D.M., Bastian L.A., Marsh G.R. (2000) Slow-wave sleep and waking cognitive performance II: Findings among middle-aged adults with and without insomnia complaints. Physiology & Behavior 70, 127-134.
|
 Fullagar H.H.K., Skorski S., Duffield R., Hammes D., Coutts A.J., Meyer T. (2015) Sleep and athletic performance: the effects of sleep loss on exercise performance, and physiological and cognitive responses to exercise. Sports Medicine 45, 161-186.
|
 Gregory J., Johns D.P., Walls J.T. (2007) Relative vs. absolute physiological measures as predictors of mountain bike cross-country race performance. The Journal of Strength & Conditioning Research 21, 17-22.
|
 Jarraya M., Jarraya S., Chtourou H., Souissi N., Chamari K. (2013) The effect of partial sleep deprivation on the reaction time and the attentional capacities of the handball goalkeeper. Biological Rhythm Research 44, 503-510.
|
 Jewett M.E., Dijk D.-J., Kronauer R.E., Dinges D.F. (1999) Dose-response relationship between sleep duration and human psychomotor vigilance and subjective alertness. Sleep 22, 171-179.
|
 Kerkhof G.H., Geuke M.E.H., Brouwer A., Rijsman R.M., Schimsheimer R.J., Van Kasteel V. (2013) Holland Sleep Disorders Questionnaire: a new sleep disorders questionnaire based on the International Classification of Sleep Disorders-2. Journal of Sleep Research 22, 104-107.
|
 Knufinke M., Nieuwenhuys A., Geurts S.A.E., Coenen A.M.L., Kompier M.A.J. (2018a) Self-reported sleep quantity, quality and sleep hygiene in elite athletes. Journal of Sleep Research 27, 78-85.
|
 Knufinke M., Nieuwenhuys A., Geurts S.A.E., Møst E.I.S., Maase K., Moen M.H., Coenen A.M.L., Kompier M.A.J. (2018b) Train hard, sleep well? Perceived training load, sleep quantity and sleep stage distribution in elite level athletes. Journal of Science and Medicine in Sport 21, 427-432.
|
 Lastella M., Roach G.D., Halson S.L., Martin D.T., West N.P., Sargent C. (2015a) Sleep/wake behaviour of endurance cyclists before and during competition. Journal of Sports Sciences 33, 293-299.
|
 Lastella M., Roach G.D., Halson S.L., Sargent C. (2015b) Sleep/wake behaviours of elite athletes from individual and team sports. European Journal of Sport Science 15, 94-100.
|
 Leeder J., Glaister M., Pizzoferro K., Dawson J., Pedlar C. (2012) Sleep duration and quality in elite athletes measured using wristwatch actigraphy. Journal of Sports Sciences 30, 541-545.
|
 Lucas S.J., Anson J.G., Palmer C.D., Hellemans I.J., Cotter J.D. (2009) The impact of 100 hours of exercise and sleep deprivation on cognitive function and physical capacities. Journal of Sports Sciences 27, 719-728.
|
 Mah C.D., Mah K.E., Kezirian E.J., Dement W.C. (2011) The effects of sleep extension on the athletic performance of collegiate basketball players. Sleep 34, 943-950.
|
 Manfredini R., Manfredini F., Fersini C., Conconi F. (1998) Circadian rhythms, athletic performance, and jet lag. British Journal of Sports Medicine 32, 101-106.
|
 Meijman T.F., Thunnissen M.J., de Vries-Griever A.G.H. (1990) The after-effects of a prolonged period of day-sleep on subjective sleep quality. Work and Stress 4, 65-70.
|
 Mitterling T., Högl B., Schoenwald S.V., Hackner H., Gabelia D., Biermayr M., Frauscher B. (2014) Sleep and respiration in 100 healthy caucasian sleepers - a polysomnographic study according to American Academy of Sleep Medicine standards. Sleep 38, 867-875.
|
 Moser D., Anderer P., Gruber G., Parapatics S., Loretz E., Boeck M., Kloesch G., Heller E., Schmidt A., Danker-Hopfe H., Saletu B., Zeitlhofer J., Dorffner G. (2009) Sleep classification according to AASM and Rechtschaffen & Kales: effects on sleep scoring parameters. Sleep 32, 139-149.
|
 Mougin F., Davenne D., Simon-Rigaud M., Renaud A., Garnier A., Magnin P. (1988) Disturbance of sports performance after partial sleep deprivation. Comptes Rendus des Séances de la Société de Biologie et de ses Filiales 183, 461-466.
|
 Paquet J., Kawinska A., Carrier J. (2007) Wake detection capacity of actigraphy during sleep. Sleep 30, 1362-1369.
|
 Rae D.E., Chin T., Dikgomo K., Hill L., McKune A.J., Kohn T.A., Roden L.C. (2017) One night of partial sleep deprivation impairs recovery from a single exercise training session. European Journal of Applied Physiology 117, 699-712.
|
 Reilly T., Atkinson G., Edwards B., Waterhouse J., Farrelly K., Fairhurst E. (2007) Diurnal variation in temperature, mental and physical performance, and tasks specifically related to football (soccer). Chronobiology international 24, 507-519.
|
 Reyner L.A., Horne J.A. (2013) Sleep restriction and serving accuracy in performance tennis players, and effects of caffeine. Physiology & Behavior 120, 93-96.
|
 Sargent C., Halson S., Roach G.D. (2014) Sleep or swim? Early-morning training severely restricts the amount of sleep obtained by elite swimmers. European Journal of Sport Science 14, S310-S315.
|
 Sargent C., Lastella M., Halson S.L., Roach G.D. (2016) The validity of activity monitors for measuring sleep in elite athletes. Journal of Science and Medicine in Sport 19, 848-853.
|
 Schwartz J., Simon R.D. (2015) Sleep extension improves serving accuracy: A study with college varsity tennis players. Physiology & Behavior 151, 541-544.
|
 Shambroom J.R., Fabregas S.E., Johnstone J. (2012) Validation of an automated wireless system to monitor sleep in healthy adults. Journal of Sleep Research 21, 221-230.
|
 Shapiro C.M., Bortz R., Mitchell D., Bartel P., Jooste P. (1981) Slow-wave sleep: a recovery period after exercise. Science 214, 1253-1254.
|
 Skein M., Duffield R., Edge J., Short M.J., Mundel T. (2011) Intermittent-sprint performance and muscle glycogen after 30 h of sleep deprivation. Medicine and Science in Sports and Exercise 43, 1301-1311.
|
 Tilley A.J., Wilkinson R.T. (1984) The effects of a restricted sleep regime on the composition of sleep and on performance. Psychophysiology 21, 406-412.
|
 van de Water A., Holmes A., Hurley D.A. (2011) Objective measurements of sleep for non-laboratory settings as alternatives to polysomnography – a systematic review. Journal of Sleep Research 20, 183-200.
|
 Van Dongen E.V., Takashima A., Barth M., Zapp J., Schad L.R., Paller K.A., Fernández G. (2012) Memory stabilization with targeted reactivation during human slow-wave sleep. Proceedings of the National Academy of Sciences 109, 10575-10580.
|
 Van Dongen H.P., Maislin G., Mullington J.M., Dinges D.F. (2003) The cumulative cost of additional wakefulness: dose-response effects on neurobehavioral functions and sleep physiology from chronic sleep restriction and total sleep deprivation. Sleep 26, 117-126.
|
 Weiss A.R., Johnson N.L., Berger N.A., Redline S. (2010) Validity of activity-based devices to estimate sleep. Journal of Clinical Sleep Medicine 6, 336-342.
|
|
|
|
|
|
|