|
|
|
ABSTRACT |
The Test of Gross Motor Development 2 (TGMD-2) is currently the standard approach for assessing fundamental movement skills (FMS), including locomotor and object control skills. However, its extensive application is restricted by its low efficiency and requirement of expert training for large-scale evaluations. This study evaluated the accuracy of a newly-developed video-based classification system (VCS) with a marker-less sensor to assess children’s locomotor skills. A total of 203 typically-developing children aged three to eight years executed six locomotor skills, following the TGMD-2 guidelines. A Kinect v2 sensor was used to capture their activities, and videos were recorded for further evaluation by a trained rater. A series of computational-kinematic-based algorithms was developed for instant performance rating. The VCS exhibited moderate-to-very good levels of agreement with the rater, ranging from 66.1% to 87.5%, for each skill, and 72.4% for descriptive ratings. Paired t-test revealed that there were no significant differences, but significant positive correlation, between the standard scores determined by the two approaches. Tukey mean difference plot suggested there was no bias, with a mean difference (SD) of -0.16 (1.8) and respective 95% confidence interval of 3.5. The kappa agreement for the descriptive ratings between the two approaches was found to be moderate (k = 0.54, p < 0.01). Overall, the results suggest the VCS could potentially be an alternative to the conventional TGMD-2 assessment approach for assessing children’s locomotor skills without the necessity of the presence of an experienced rater for the administration. |
Key words:
Children, Fundamental movement skills, Kinect v2 sensor, Marker-less device, TGMD-2, Video-based system
|
Key
Points
- Criterion-specific thresholds were identified for children aged from 3 – 8 years which can serve as a basis for the establishment of referencing norm in local region.
- The percentage agreement (72.4%) and kappa agreement (54.0%) between the VCS and the rater in terms of descriptive ratings were found to be good and moderate, respectively
- With the use of VCS, objective, comprehensive and instant assessment of FMS locomotor skills for a large population without the necessity of the presence of an experienced rater, thereby substantially reducing human intervention during administration compared with the conventional TGMD-2 assessment approach.
- New and validated marker-less, kinematic-computational-based approach in assessing children’s locomotor skills is proved to be feasible.
|
Fundamental movement skills (FMS) comprise basic movement competencies for coordinating different body parts. FMS typically consist of three components, namely locomotor, object control and stability skills (Haywood and Getchell, 2008), which are considered the building blocks of more advanced movements required to engage in physical activities, such as those involved in sports and games (Clark and Metcalfe, 2002). Children aged three to eight years are at a crucial stage of the development of FMS (Gallahue et al., 2012; Loprinzi et al., 2015). For older children, well-developed gross movement skills are beneficial for effortless body movements, object manipulation and balance (Cools et al., 2009). Delayed acquisition of FMS may discourage physical activity (Lubans et al., 2010; Stodden et al., 2007) and cause performance deficiencies (Lucas et al., 2016; Vameghi et al., 2013) as well as various social problems for children as they grow (Bailey, 2005; Lucas et al., 2016; Roberts et al., 2011). Therefore, children’s acquisition of gross movement skills – such as body movement, stabilisation, and control as well as object manipulation skills – at an early age is critical for them to explore their surroundings. The Test of Gross Motor Development 2 (TGMD-2) is a standardised test that is widely used to screen for deficiencies in FMS and evaluate children’s development (Burton and Miller, 1998; Houwen et al., 2010; Parvinpour et al., 2019; Ulrich, 2000). Specifically, the test is employed to assess each child’s performance based on the presence or absence of 24 performance criteria for six locomotor and six object control skills (Ulrich, 2000). The rating for each performance criterion is conducted in real-time by an experienced and well-trained rater. Alternatively, a video recording is used during children’s execution of the locomotor skills, after which a trained rater reviews the captured video clips for assessment (Barnett et al., 2014). However, the TGMD-2 framework has two drawbacks. First, the raters must undergo systematic training processes before the delivery of accurate ratings (Zuvela et al., 2011). Second, video reviews constitute a time-consuming process because they involve repeatedly assessing slow-motion clips for detailed observations, in particular among inexperienced raters. These drawbacks prevent the test from being applied extensively and render it inefficient for screening large populations (Bisi et al., 2017). Establishing a monitoring system to detect even minor delays in the development of FMS is a critical task. A human gait capture and analysis system that can be used to evaluate the motor development of children is necessary (Logan et al., 2017; Williams and Monsma, 2007). The use of inertial measurement units (IMUs) to assess the locomotor subtest of TGMD-2 was demonstrated to constitute a valid approach with high usability, reliability, and objectivity (Bisi et al., 2017). With the help of such IMUs, video recording, or well-trained and experienced raters, were not required throughout the assessment, thus simplifying the entire process. However, two limitations may hinder the applicability of this approach. First, five IMU sensors must be placed on particular positions on a participant’s body (Bisi et al., 2017), an approach that would be inefficient and time-consuming when administered to a large population (Zuvela et al., 2011). Second, this approach could not provide a complete evaluation of running abilities due to the exclusion of performance criterion 3 of TGMD-2 (Bisi et al., 2017). Accordingly, a time-saving evaluation system for a complete real-time assessment process is crucial for the efficient application of TGMD-2. Microsoft Kinect v2 is a cost-effective and marker-less alternative tool for movement analysis, which can be used to overcome the aforementioned limitations of the IMU-based approach when used to assess the locomotor subtest of TGMD-2. In conjunction with Microsoft’s in-house Kinect SDK software, a body tracking model with 25 joints is established for capturing 3D joint trajectory data (Figure 1). Besides, studies have documented that the Kinect v2 sensor is a potentially effective clinical assessment tool for collecting spatiotemporal gait data, such as body joint locations and displacement, as well as kinematic data, such as the sagittal plane kinematics of the knee and hip joint during a gait cycle (Eltoukhy et al., 2017; Mentiplay et al., 2015; Motiian et al., 2015; Springer and Seligmann, 2016). According to these studies, the Kinect v2 sensor can adequately identify a set of kinematic parameters in various activities. The aim of the present study was to develop a video-based classification system (VCS) and evaluate its accuracy in assessing schoolchildren’s locomotor skills under the TGMD-2 framework. The study tested the hypothesis that implementing a VCS incorporated with a marker-less Kinect v2 sensor was potentially an alternative for assessing children’s locomotor skills instantly, without the presence of an experienced rater and yielding comparable agreement with the conventional TGMD-2 rating approach.
ParticipantsTotally, 272 children from four schools were approached and 213 children consented, through their parents, to participate in the study. Data from 10 children were excluded from the study due to data loss, resulting in a convenience sample of 203 children with typical development from Hong Kong, who were aged three to eight years (5.4 ± 1.6 years). Of the participating children, 74 were from one primary school and 129 were from three kindergartens. Table 1 presents the demographic characteristics of the sample. None of the children had any reported development delays according to their parents and teachers. Informed consent (approved by the Human Research Ethics Committee of the Education University of Hong Kong) was obtained from the participants’ parents prior to data collection.
Experimental setupMicrosoft Kinect v2 (Microsoft Corp., Redmond, WA) is a marker-less motion capture system with one single camera that has increasingly been employed in kinematic data analysis (Han et al., 2013). In this study, a 3.71m-long runway was set with pre-specified points at 1.85 m, 1.98 m and 3.19 m (Figure 2). A single Kinect v2 sensor was positioned to alternate between two predetermined points extending from 3.19 m and 3.71 m of the runway – one representing setting 1 and the other representing setting 2. These were distanced 2.99 m and 3.50 m away from the two predetermined points, respectively. To record the executions of all six locomotor activities, the Kinect v2 sensor was placed at either setting 1 or 2 pointing at 1.98 m or 1.85 m of the runway by using a static tripod at a height of 0.75 m, constituting an inclination angle. The inclination angle between the runway and setting 1 or 2 was adjusted slightly, depending on the activity being tested. Empirically, the capture of the six locomotor skills was found to be the most optimal when the inclination angle was set to 60.0° for the assessment of run, gallop, and horizontal jump skills in setting 1 and to 69.0° for the assessment of leap, hop, and slide skills in setting 2 (Figure 2).
Procedure and FMS assessmentTGMD-2 assesses the gross motor development of children aged three to 10 years. For the locomotor subtest, it is used to evaluate the performance of a child’s locomotor skills based on the following six activities: run, gallop, hop, leap, horizontal jump, and slide (Ulrich, 2000). This study followed the standard administration procedures for the locomotor subtest of TGMD-2. According to the TGMD-2 guidelines (Ulrich, 2000), specific instructions for each activity were provided to each child, before the child was allowed to perform two warm-up trials to ensure that they fully understood the correct techniques to complete each move. The children began each activity in a standing position and subsequently performed each skill along the runway for two trials. The activities were also recorded from the side view using a 1080p digital camera placed just behind setting 2. The corresponding video clips were used for post hoc analyses. Based on the TGMD-2 guidelines (Ulrich, 2000), 24 criteria were used for the locomotor subtest, including four performance criteria for each skill of run, gallop, horizontal jump, and slide, as well as five performance criteria for hop and three performance criteria for leap. Each criterion was given a score of 1 or 0 denoting “pass” or “fail,” respectively. Scores for a particular activity (two trials) were summed up as a skill score for this activity. Subsequently, all six skill scores were summed up to acquire subtest raw scores (0 – 48 points). Moreover, the subtest raw scores were converted into standard scores (1 – 20 points) by considering the child’s age. Each child was subsequently assigned a descriptive rating (i.e. “very poor”, “poor”, “below average”, “average”, “above average”, “superior” and “very superior”) corresponding to their standard scores and age according to the TGMD-2 guidelines.
Development of VCSTo score each performance criterion, 24 algorithms (each with its own initial threshold) were developed in Microsoft Visual C++ (Microsoft Corp., USA) on the basis of a series of body frames captured using the Kinect v2 system. Table 2 and Table 3 show the 24 algorithms along with their respective brief descriptions and terminologies with illustrations. Each body frame denoted the positions of a set of joints (Figure 1) relative to the Kinect v2 camera position; i.e. the sensor position was the origin (0, 0, 0) of the world coordinate system. All calculations regarding any specific performance criterion were based on the joint locations of one or more body frames. To optimise the system for new data obtained from non-assessed children, the optimal threshold for each performance criterion must be determined. In this study, algorithms in Python 3.5 software were developed for threshold tuning. Accordingly, 42 children (20% of the sample) were randomly selected according to the age distribution. For each performance criterion, the scores of these 20% of children rated by the VCS and a trained rater were compared individually, with the highest agreement set as the threshold for that particular performance criterion in the cohort. By repeating the above threshold tuning procedures 1000 times (Parks et al., 2016), the mean, mode and median of the threshold for each performance criterion that achieved the highest agreement of the scores between the VCS and the rater were then calculated respectively. The mean thresholds were assigned as the optimal thresholds for all 24-performance criteria.
Data analysisPrior to the administration of the study, inter-rater reliability between a trained rater and an experienced physical education teacher was conducted. Moreover, intra-rater reliability of the trained rater was also evaluated in a pilot study with 101 elementary school students aged six to nine years old (7.62 ± 0.93 years). The inter- and intra-rater reliability were found to be good with intra-class correlation coefficients ranging from 0.82 to 0.91. To evaluate the system’s predictability, this study calculated the percentage agreement between the VCS and the rater’s scores in terms of the performance criterion for each skill component, and the standard scores.
- A series of optimal thresholds of the performance criterion for
each skill component was applied to the system; subsequently, raw scores assigned
to each performance criterion of each skill for all 203 children were compared.
- After the conversion of the raw scores to the standard scores,
the corresponding descriptive ratings for all 203 children were compared.
Statistical analysisPaired t-test was used to compare the standard scores obtained by the VCS and the trained rater. In addition, Tukey mean difference plot was used to evaluate differences in the standard scores between the VCS and the rater. Percentage agreement and kappa agreement were calculated to assess agreement of standard scores between the VCS and the rater for validation. The associated interpretive scales for percentage agreement and Kappa agreement were used in accordance with Sasyniuk et al. (2007) and Cohen (1960), respectively, as shown in Table 4a and Table 4b. Statistical significance was set at p < 0.05.
Considering the time spent on downloading clips from the camera and organising a collection of video clips, the mean time required for the study rater to evaluate all 24 performance criteria for each participant and to offer a descriptive rating was about 20 minutes. By contrast, an experienced and trained rater would be able to assess each skill performance in real time without any recording and slow motion reviewing of videos. Similarly, the VCS also analysed the data captured by the Kinect v2 sensor in real time while each child executed a specified move. It then directly generated an overall performance criterion score immediately after the child completed the move. In terms of threshold tuning, the mean, mode and median values of the thresholds for each performance criterion obtained were found to be identical (Table 5). Overall for every skill, a comparable agreement was achieved between the VCS’ scores and the rater’s scores. Based on data obtained from children aged three to eight years (203 children in total), the mean percentage agreement between the VCS and the rater for each skill ranged from 66.1% to 87.5%. Notably, only the mean average accuracy rates for hop did not exceed 75%, while those for run, gallop and leap were higher than 80%. For the percentage agreement between the VCS and the rater for each performance criterion, the percentage agreement levels for all performance criteria ranged from 43.6% to 92.9% (Table 6). The percentage agreement values for criterion 2 (43.6%) and criterion 5 (55.7%) under hop were moderate in agreement (Sasyniuk et al., 2007). A paired t-test showed that the ratings of the VCS and the rater were not significantly different, with p=0.20, while the correlation between the two approaches was 0.762 (p < 0.01). A Tukey mean difference plot for all standard scores indicated a mean difference (standard deviation) of -0.16 (1.8) and the corresponding 95% confidence interval was 3.5 (Figure 3). The mean (standard deviation) standard scores for the VCS and the rater were 8.4 (2.8) and 8.2 (2.4), respectively (Table 7). As for descriptive ratings, assessment of the data obtained from children aged three to eight revealed the accuracy to be good (Sasyniuk et al., 2007). The percentage agreement between the VCS and the rater in terms of descriptive ratings was 72.4%. Taking into account the possibilities of chance agreement, the kappa agreement for the descriptive ratings between the two approaches was 0.54 with p < 0.01. Comparison of the two rating approaches showed that they differed in their descriptive ratings for 56 children: the VCS underestimated the ratings for 30 children and overestimated those for 26 children when compared with the trained rater. Ratings provided by the VCS and the trained rater both ranged from “very poor” to “above average”. On the other hand, there were no ratings from the two approaches falling into the descriptive categories of “very superior” and “superior”. Furthermore, most of the children were rated “average” by both the VCS and the trained rater, with the corresponding figures being 57.2% and 58.6%, respectively. The rater considered 25.1% of the children to be “below average”, whereas the VCS considered 17.7% of them to be “below average”. The VCS rated a higher percentage of children as “poor” and “very poor” compared with the trained rater (Table 8).
This study examined the accuracy of a newly developed video-based kinematics computation system, namely VCS, in instantly providing objective and comprehensive assessments of young children’s FMS under the TGMD-2 framework. Unlike the conventional TGMD-2 rating approach, which depends solely on humans’ visual analysis, the key parameters for determining assessment scores in the proposed VCS are based on data captured by the Kinect v2 sensor and the VCS. Through computing a series of specific parameters, such as joint angles and duration off the ground, the system can quantify the score for each performance criterion according to the TGMD-2 locomotor subtest guidelines. By incorporating the marker-less Kinect v2 sensor, the VCS can be an assistive tool for an inexperienced rater to evaluate children’s locomotor skills in an instant, without the necessity for the presence of an experienced rater. At the same time, it yielded comparable accuracy with the conventional TGMD-2 assessment approach. In terms of efficiency, the VCS can provide accurate and objective assessments in a short time. According to the TGMD-2 guidelines, an experienced and well-trained rater is required to assess each locomotor skill in real-time. However, it is formidable for a rater with less experience to evaluate each skill simultaneously as they are required to observe and identify whether the child has achieved the performance criteria for that skill or not. Alternatively, videos can be recorded for the inexperienced rater to confirm the ratings, which can be particularly useful for ambiguous cases when skill performance can be reviewed repeatedly, in slow motion. However, this is a process that involves substantial time and human intervention. A study also concluded that even though the raters may be well-trained and experienced, the approach is confined in terms of the ability to identify all performance criteria during real-time assessment (Ward, 2019). The results of this study showed that the VCS was a potential alternative to rating children’s FMS performance, particularly in contexts where there are no experienced raters available, for example in kindergarten settings. This system can thus serve as an alternative to the conventional approach, especially for inexperienced raters, since it facilitates kinematic data processing and sufficiently reduces the necessity of human intervention in larger-scale assessments of FMS. In general, the research yielded identical results for the mean, mode and median of the threshold of each performance criterion (Table 5) after 1000 times’ random sampling and comparison, suggesting uniform distributions of the thresholds. This evidences that the thresholds for each performance criterion obtained would be the optimal results. Favourable agreement levels were discovered between the VCS and the rater who conducted visual analysis, thus revealing that the VCS can achieve accurate and comprehensive assessments with low latency. The mean percentage agreements between the VCS and the rater in terms of the scores for each skill ranged from 66.1% to 87.5%. This finding suggests that the VCS can be used to identify most distinctive features and even minor details associated with all six skill motions, similar to the human rater’s performance. However, some performance criteria were associated with percentage agreement values of less than 60%; for example, performance criterion 2 and 5 under hop were associated with percentage agreement values of 43.6% and 55.7%, respectively. The decrease in agreement may be ascribed to the field of view of the Kinect v2 sensor in a single side of the runway (Webster and Celik, 2014). Consequently, the view of children’s execution of the skills was restricted to the limited capture range of the sensor and the joint movements on the other side were occasionally blocked from occlusion and unable to be captured. Besides, the use of a non-preferred leg would hinder children from successfully hopping. Since hopping requires tremendous muscular strengths and balancing skills (Krasnow and Wilmerding-Pett, 2015), the hopping techniques were still improving for children aged above five (Krasnow and Wilmerding-Pett, 2015). Hence, hopping with non-dominant leg would be difficult for early aged children. The paired t-test showed that there was no significant difference but a significant correlation between the standard scores rated by the VCS and the trained rater. From Tukey mean difference plot analysis, the two rating approaches did not differ significantly in terms of the standard score at the group level. The mean difference of the two approaches was close to zero difference, suggesting there was no bias. However, the 95% confidence interval limit of agreement indicated considerable variations at the individual level that the VCS approach may be 3.71 scores inferior or 3.38 superior to the rater approach. These results reveal that the VCS had certain levels of agreement with the traditional rating approach at the group level, whereas the agreement between two approaches at the individual level would require further improvement. Regarding descriptive ratings, the difference between the VCS and the rater’s mean scores was not significant, demonstrating that the VCS could be used for objectively and comprehensively assessing children’s FMS. The percentage agreement and kappa agreement between the VCS and the rater in terms of their descriptive ratings were found to be good and moderate, respectively (Sasyniuk et al., 2007; Cohen, 1960). However, the VCS was more likely to assign lower standard scores than the human rater, thus explaining the observation that the VCS rated a higher percentage of children as “poor” and “very poor” compared with the human rater. In addition, it was noted that there were no participants rated “very superior” or “superior” by either the rater or the VCS, which was possibly an indication of general inferiority of locomotor skills in the samples. This was one of the limitations pertained to the insufficient sample age range on account of convenience sampling. In this study, children aged nine and 10 years were not included, despite them being within the proper age range based on the TGMD-2 guidelines. Therefore, future evidence is necessary to ascertain whether the VCS can classify children with good FMS ability within the categories of “superior” or “very superior”. By developing the VCS, this study contributes to the current understanding of approaches for designing algorithms to instantly assess children’s FMS without the presence of an experienced rater. Similarly, a previous study presented an approach that involved the use of wearable sensors to assess 23 of the 24 criteria under the TGMD-2 locomotor subtest (Bisi et al., 2017). This approach was validated as having reliably and objectively evaluated FMS (Bisi et al., 2017). However, the approach could not provide a rapid or reliable assessment of the performance criterion 3 pertaining to running. The approach not only involved a time-consuming process of placing several sensors on each participant’s body but also required two minutes to compute the results for each participant. Accordingly, this approach does not constitute a fully automated system in that it lacks time efficiency because of its relatively long running time and its requirement of manually evaluating performance criterion 3. In contrast, for performance criteria and descriptive ratings, the VCS proved to be objective, accurate, and easy to use due to the incorporation of marker-less devices. Furthermore, the accuracy of the VCS achieved in the current study (72.4%) was comparable to that obtained by using wearable sensors (73%) (Bisi et al., 2017). Therefore, moving to a marker-less system is warranted in that the VCS obviates the recording of video clips, extensive rater training, and additional system running time. It substantially simplifies the assessment procedure and saves considerable time. Apart from the insufficient sample range, another limitation that might arise was evident when the Kinect v2 sensor was used in the VCS. Considering the study findings, the accuracy between the VCS and the rater would be adversely affected by the sophisticated threshold determination processes and limited field of view of the Kinect v2 sensor. The Kinect v2 sensor was unable to track and distinguish different body joints when overlapping of joints occurred, thereby leading to a decrease in accuracy. A study has documented the possibility of the use of two Kinect sensors on either side to collect FMS data by converting the joint positions of one sensor into the world coordinate system of another (Rosenberg et al., 2016). To address the limitation of the Kinect v2 sensor, future studies should consider using more than one sensor to capture movements in order to collect kinematic data from both sides of the runway. This has been a preliminary study developing and evaluating the accuracy of the VCS in assessing young children without disability or other health issues. However, existing empirical studies have validated TGMD-2 for children with health problems (Houwen et al., 2010; MacDonald et al., 2013; Pan et al., 2009). Hence, children with wider range of ability, for example, diagnosed with developmental delays, could be included in experimental assessments in future clinical research in order to improve and validate the general applicability of the VCS. A further validation study should be conducted to see whether the system is sensitive to differentiate children with developmental delay from typically developed children. For the sake of early screening for developmental delay, more subject recruitment in different ages would be required to establish a local reference norm. Moreover, the proposed system was verified to be feasible for performing video-based skill assessments by using the object control subtest of TGMD-2 as well as of the new version, TGMD-3 (Allen et al., 2017). Details of the spatiotemporal and kinematic parameters used in this study can be accessed for further analysis of the FMS of the participants in this study. The present results may serve as a valuable resource for intervention and rehabilitation studies. Future studies on the automation of VCS under the TGMD-2 standard should also investigate the possibility of using portable devices (e.g., smartphones or tablets) other than Kinect v2 sensors to further enhance the applicability and convenience of systems for motor control monitoring. Direct and more informative feedback could be provided with the help of a user-friendly app. For achieving accurate and time-efficient predictions based on kinematic computations, the VCS could be improved to identify key parameters related to additional activities under other physical evaluation frameworks, such as the Movement Assessment Battery for Children, Second Edition (Henderson et al., 2007), and the Bruininks–Oseretsky Test of Motor Proficiency, Second Edition (Bruininks and Bruininks, 2005). Doing so can enable comprehensive, efficient, and objective evaluation of the full scope of children’s development (Logan et al., 2017).
A video-based kinematic computation system, namely VCS, combined with a kinematic-data-capturing sensor, was developed. Its accuracy was evaluated and found to be good with the conventional TGMD-2 assessment approach in terms of percentage agreement. The VCS was found to be a potential alternative to assessing children’s locomotor skills instantly without the necessity of the presence of an experienced rater, thereby minimising human intervention. The study revealed the feasibility of the system in assessing other movement capabilities, such as object control, fine motor skills, stability, and even balance. These assessments can serve as a reference for monitoring children’s growth and screening for developmental delays, thereby facilitating early clinical interventions.
ACKNOWLEDGEMENTS |
This research was funded by the Departmental Research Fund, grant number 04182. The experiments comply with the current laws of the country in which they were performed. The authors have no conflict of interest to declare. |
|
AUTHOR BIOGRAPHY |
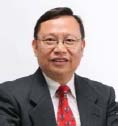 |
Daniel H. K. Chow |
Employment: Chair Professor of Health and Sports Science in the Department of Health and Physical Education at the Education University of Hong Kong. |
Degree: PhD |
Research interests: Human movement analysis, biomechanics, ergonomics and sports health. |
E-mail: danielchow@eduhk.hk |
|
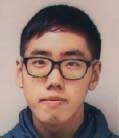 |
Wilson H. W. Cheng |
Employment: Research Assistant in the Department of Health and Physical Education at the Education University of Hong Kong. |
Degree: Bachelor |
Research interests: Human movement analysis, biomechanics and sports health. |
E-mail: hwcheng@eduhk.hk |
|
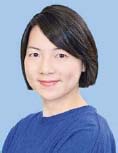 |
Simone S. M. Tam |
Employment: Lecturer in the Department of Health and Physical Education at the Education University of Hong Kong. |
Degree: MSc |
Research interests: Fundamental movement and motor performance of young children. |
E-mail: ssmtam@eduhk.hk |
|
|
|
REFERENCES |
 Allen K.A., Bredero B., Van-Damme T., Ulrich D.A., Simon J. (2017) Test of Gross Motor Development – 3 (TGMD-3) with the Use of Visual Supports for children with Autism Spectrum Disorder: Validity and Reliability. Journal of Autism and Development Disorders 47, 813-833.
|
 Bailey R. (2005) Evaluating the relationship between physical education, sport and social inclusion. Educational Review 57, 71-90.
|
 Barnett L.M., Minto C., Lander N., Hardy L.L. (2014) Interrater reliability assessment using the Test of Gross Motor Development-2. Journal of Science and Medicine in Sport 17, 667-670.
|
 Bisi M.C., Panebianco G.P., Polman R., Stagni R. (2017) Objective assessment of movement competence in children using wearable sensors: An instrumented version of the TGMD-2 locomotor subtest. Gait & Posture 56, 42-48.
|
 Bruininks, R.H. and Bruininks, B.D. (2005) Bruininks-Osretsky Test of
Motor Proficiency. 2nd Edition. Pearson, Minneapolis, MN.
|
 Burton, A.W. and Miller, D.E. (1998) Movement Skill Assessment.
Human Kinetics, Champaign, IL.
|
 Clark, J.E. and Metcalfe, J.S, (2002) The mountain of motor development:
a metaphor. In: Motor development: Research and reviews. Ed:
Clark, J.E. and Humphrey, J.H. National Association for Sport
and Physical Education, Reston, VA, 62-95.
|
 Cohen J. (1960) A coefficient of agreement for nominal scales. Educ Psychol Meas. 20, 37-46.
|
 Cools W., Martelaer K.D., Samaey C., Andries C. (2009) Movement skill assessment of typically developing preschool children: A review of seven movement skill assessment tools. Journal of Sports Science & Medicine 8, 154-168.
|
 Eltoukhy M., Oh J., Kuenze C., Signorile J. (2017) Improved kinect-based spatiotemporal and kinematic treadmill gait assessment. Gait & Posture 5, 77-83.
|
 Gallahue D.L., Ozmun, J.C. and Goodway, J. D. (2012) Understanding
Motor Development: Infants, Children, Adolescents, Adults. 7th
Edition. McGraw-Hill Education, New York, NY.
|
 Han J., Shao L., Xu D., Shotton J. (2013) Enhanced Computer Vision with Microsoft Kinect Sensor: A review. IEEE Transactions on Cybernetics 43, 1318-1334.
|
 Haywood, K.M. and Getchell, N. (2008) Lifespan motor development. 5th
edition. Human Kinetics, Champaign, IL.
|
 Henderson, S.E., Sugden, D.A. and Barnett, A.L. (2007) Movement
Assessment Battery for children - 2 Examiner's Manual.
London: Harcourt Assessment, Pearson.
|
 Houwen S., Hartman E., Jonker L., Visscher C. (2010) Reliability and validity of the TGMD-2 in primary-school-age children with visual impairments. Adapted Physical Activity Quarterly 27, 143-159.
|
 Krasnow, D.H. and Wilmerding-Pett, M.V. (2015) Development in
locomotor skills. In: Motor Learning and Control for Dance
Principles and Practices for Performers and Teachers.
Australia: Human Kinetics. 43-60.
|
 Logan S.W., Barnett L.M., Goodway J.D., Stodden D.F. (2017) Comparison of performance on process-and product-oriented assessments of fundamental motor skills across childhood. Journal Sports Science 35, 634-641.
|
 Loprinzi P.D., Davis R.E., Fu Y.C. (2015) Early motor skill competence as a mediator of child and adult physical activity. Preventive Medicine Reports 2, 833-838.
|
 Lubans D.R., Morgan P.J., Cliff D.P., Barnett L.M., Okely A.D. (2010) Fundamental movement skills in children and adolescents: review of associated health benefits. Sports Medicine 40, 1019-1035.
|
 Lucas B.R., Elliott E.J., Coggan S., Pinto R.Z., Jirikowic T., McCoy S.W., Latimer J. (2016) Interventions to improve gross motor performance in children with neurodevelopmental disorders: a meta-analysis. BMC Pediatrics 16, 193.
|
 MacDonald M., Lord C., Ulrich D.A. (2013) The relationship of motor skills and social communicative skills in school-aged children with autism spectrum disorder. Adapted Physical Activity Quarterly 30, 271-282.
|
 Mentiplay B.F., Perraton L.G., Bower K.J., Pua Y.H., McGaw R., Heywood S., Clark R.A. (2015) Gait assessment using the Microsoft Xbox One Kinect: Concurrent validity and inter-day reliability of spatiotemporal and kinematic variables. Journal of Biomechanics 48, 2166-2170.
|
 Motiian S., Pergami P., Guffey K., Mancinelli C.A., Doretto G. (2015) Automated extraction and validation of children's gait parameters with the Kinect. BioMedical Engineering OnLine 14, 112.
|
 Pan C.Y., Tsai C.L., Chu C.H. (2009) Fundamental movement skills in children diagnosed with autism spectrum disorders and attention deficit hyperactivity disorder. Journal of Autism and Developmental Disorders 39, 1694-1705.
|
 Parks N.A., Gannon M.A., Long S.M., Young M.E. (2016) Bootstrap Signal-to-Noise Confidence Intervals: An Objective Method for Subject Exclusion and Quality Control in ERP Studies. Frontiers on Human Neuroscience 10, 50.
|
 Parvinpour S., Shafozadeh M., Balali M., Abbasi A., Wheat J., Davids K. (2019) Effects of Developmental Task Constraints on Kinematic Synergies during Catching in Children with Developmental Delays. Journal of Motor Behavior 7, 1-17.
|
 Roberts G., Anderson P.J., Davis N., De-Luca C., Cheong J., Doyle L.W. (2011) Victorian Infant Collaborative Study Group. Developmental coordination disorder in geographic cohorts of 8-year-old children born extremely preterm or extremely low birthweight in the 1990s. Developmental Medicine & Child Neurology 53, 55-60.
|
 Rosenberg M., Thronton A.L., Lay B.S., Ward B., Nathan D., Braham R. (2016) Development of a Kinect Software Tool to Classify Movements during Active Video Gaming. PloS one 11, 7.
|
 Sasyniuk T.M., Mohtadi N.G., Hollinshead R.M., Russell M.L., Fick G.H. (2007) The inter-rater reliability of shoulder arthroscopy. Arthroscopy 23, 971-977.
|
 Springer S., Seligmann G.Y. (2016) Validity of the Kinect for Gait Assessment: A Focused Review. Sensors 16, 194.
|
 Stodden D., Goodway J. D. (2007) The Dynamic Acssociation Between Motor Skill Development and Physical Activity. Journal of Physical Education, Recreation & Dance 78, 33-49.
|
 Ulrich, D.A. (2000) TGMD-2: Test of Gross Motor Development, 2nd
edition. Pro-Ed, Austin, TX
|
 Vameghi R., Shams A., Shamsipour-Dehkordi P. (2013) The effect of age, sex and obesity on fundamental motor skills among 4 to 6 years-old children. Pakistan Journal of Medical Sciences 29, 586-589.
|
 Ward, B. (2019) Techological approaches to fundamental movement skill
assessment in children. Doctoral thesis, The University of
Western Australia, Australia.
|
 Webster D., Celik O. (2014) Systematic review of Kinect applications in elderly care and stroke rehabilitation. Journal of NeuroEngineering and Rehabilitation 11, 108.
|
 Williams, H.G. and Monsma, E.V. (2007) Assessment of Gross Motor
Development. In: Psychoeducational Assessessment of
Preschool Children, Ed: Bracken, B.A. and Nagle, R.J.,
Mahwah, 4th edition. Mahwah, NJ: Lawrence Erlbaum
Associates. 397-433
|
 Zuvela F., Bozanic A., Miletic D. (2011) POLYGON – A New Fundamental and Movement Skills Test for 8 Year Old Children: Construction and Validation. Journal of Sports Science and Medicine 10, 157-163.
|
|
|
|
|
|
|