|
|
|
ABSTRACT |
There is only very limited data examining cardiovascular responses in real-world endurance training/competition. The present study examines the influence of a marathon race on non-linear dynamics of heart rate (HR) variability (HRV). Eleven male recreational runners performed a self-paced marathon road race on an almost flat profile. During the race, heart rate and beat-to-beat (RR) intervals were recorded continuously. Besides HRV time-domain measurements, fractal correlation properties using short-term scaling exponent alpha1 of Detrended Fluctuation Analysis (DFA-alpha1) were calculated. The mean finishing time was 3:10:22 ± 0:17:56 h:min:s with a blood lactate concentration of 4.04 ± 1.12 mmol/L at the end of the race. Comparing the beginning to the end segment of the marathon race (Begin vs. End) significant increases could be found for km split time (p < .001, d = .934) and for HR (p = .010, d = .804). Significant decreases could be found for meanRR (p = .013, d = .798) and DFA-alpha1 (p = .003, d = 1.132). DFA-alpha1 showed an appropriate dynamic range throughout the race consisting of both uncorrelated and anti-correlated values. Lactate was consistent with sustained high intensity exercise when measured at the end of the event. Despite the runners slowing after halfway, DFA-alpha1 continued to fall to values seen in the highest intensity domain during incremental exercise testing in agreement with lactate assessment. Therefore, the discrepancy between the reduced running pace with that of the decline of DFA-alpha1, demonstrate the benefit of using this dimensionless HRV index as a biomarker of internal load during exercise over the course of a marathon race. |
Key words:
Autonomic nervous system, Detrended Fluctuation Analysis, non-linear dynamics, endurance exercise
|
Key
Points
- DFA-alpha1 is defined as an indicator of relative internal load and proxy of organismic demands
- DFA-alpha1 showed a wide dynamic range throughout the marathon race consisting of both uncorrelated and anti-correlated values supporting its ability to finely differentiate internal physiologic status and indicating dynamic modulation processes
- Lactate as a measure of internal metabolic load was consistent with sustained high intensity exercise when measured at the end of the event
- The discrepancy between the reduced running pace with that of the decline of DFA-alpha1 and high lactate measures may demonstrate the utility of DFA-alpha1 as a biomarker of internal load over the course of a marathon race showing complex modulatory dynamics with increasing fatigue
|
Marathon running has been extensively studied in terms of underlying cardiovascular physiology, substrate metabolism, biomechanical efficiency, and even motivational factors (Zinner and Sperlich, 2016). However, although there are data describing behavior of heart rate (HR) variability (HRV) during the actual event (Franco et al., 2014), the specific indices studied have been less than optimal for assessment of real-time exercise intensity because of a lack of appreciable changes during exercise. Although time- and frequency-domain related linear HRV measures such as the standard deviation of all normal beat-to-beat (RR) intervals SDNN, the root mean square of successive differences (RMSSD) and high-frequency (HF) power have been shown to change with exercise intensity, they generally follow a trajectory showing a loss of dynamic range around the first ventilatory threshold (VT1) (Tulppo et al., 1996; Cottin et al., 2007; Karapetian et al., 2008). In this context, non-linear HRV analysis is a promising approach for internal load monitoring and physiologic threshold determination (Gronwald et al., 2020a). Detrended Fluctuation Analysis (DFA; Peng et al., 1995) with its dimensionless short-term scaling exponent alpha1 (DFA-alpha1) reveals fractal correlation properties of HR time series within a range of 0 and 2 and shows clear dependency on changes to organismic demands related to external load markers such as intensity, cadence and duration, leading to insights in complex regulation during endurance exercise (for review see Gronwald and Hoos, 2020; for application see Gronwald et al., 2020a). A recent investigation has shown that this non-linear index based on fractal correlation properties of HRV changes with exercise intensity with the midpoint of its range being near the VT1, thereby demonstrating potential as a physiological marker for a wide range of workloads (Gronwald et al., 2020a; Rogers et al., 2021). Therefore, this approach shows great potential regarding the investigation of Network Physiology of Exercise (NPE) recently introduced by Balagué et al. (2020). However, despite the growing body of literature from laboratory studies in this field, HRV data from real-world endurance training/competition is rarely available for linear HRV indices (Sztajzel et al., 2006; Cottin et al., 2007; Franco et al., 2014). For non-linear DFA-alpha1, Gronwald et al. (2020b) conducted a pilot study during prolonged severe to maximal endurance exercise during a 10km road race and found a clear reduction in correlation properties in RR fluctuations that might be attributed to increased organismic demands. Furthermore, they showed that a higher performance outcome in terms of a faster total running time implies the necessity of an earlier loss of correlation properties during the beginning of the exercise regimen, indicating an accelerated alteration of cardiac autonomic regulation and cardio-pulmonary onset-kinetics at a higher performance standard. The fact that a self-paced competitive endurance race performance provides more insights into the complex organismic regulation during se-vere to maximum intensity exercise, elucidates the need for further research in this field. In addition, since much of the current information related to exercise and DFA-alpha1 has been obtained during short-term incremental ramp protocols (Gronwald and Hoos, 2020), further insights may be gleaned by observing the behavior over the course of longer exercise sessions such as a marathon road race. The purpose of this report is to gain more general insight into non-linear HRV dynamics during prolonged endurance exercise competition and to evaluate the potential of DFA-alpha1 as a marker for internal load and complex regulation during long duration exercise. Most of the exercise intensity during a marathon race is performed near aerobic threshold (Zinner and Sperlich, 2016) which is not considered a strenuous work rate (Jamnick et al., 2020), albeit for a very prolonged period of time. However, the measurement of the running speed at the aerobic threshold is generally done via short duration constant power or incremental ramp testing (Coen et al., 2001; Faude et al., 2009). Few studies have examined real time blood lactate measurements during a long road race, but evidence indicates values between 2 to 3 mmol/L (Santos et al., 2006), mostly well above typical threshold values for the aerobic threshold (Faude et al., 2009). Thus, there appears to be a possible discrepancy between a measure of internal load intensity (e.g. blood lactate concentration) with that of external load intensity (e.g. running speed at aerobic threshold). Insights into the issue of internal vs. external load monitoring are possible given the dynamic range of DFA-alpha1 throughout the spectrum of exercise intensities. Since direct sampling of blood lactate is not practical during a competitive long-distance event, only immediate post-race values will be obtained. Therefore, non-linear DFA-alpha1, along with standard linear time-domain measures of HRV will be examined for potential usefulness as internal load monitors. We assume that DFA-alpha1 add complementary information about complex cardiovascular regulation during marathon racing in addition to the rather low but steady increases in HR due to cardiovascular drift.
ParticipantsFifteen participants were recruited by newspaper advertisement or personal approach two hours before the marathon race in the warm-up area and voluntarily participated in the study. All runners were free from any medical issues. After being informed extensively about the procedures and objectives of the study, they provided informed consent in accordance to the institutional review board and the guidelines of the Helsinki World Medical Association Declaration. After exclusion of RR-interval artefacts eleven male runners (age: 36.6 ± 9.6 years; height: 1.77 ± 0.07 m; body mass: 70.9 ± 9.8 kg) with a weekly training volume of 50 to 100km, and a personal best marathon time of 2:45 to 3:30 h:min were included in the study analysis.
Study DesignThe data were collected during an official marathon race on a certified asphalt road course (German Road Races Association) with an almost flat profile (overall elevation between start and finish line: 80m; temperature: 22 °C, altitude: 282 m above sea level). During exercise, heart rate (HR) and RR-intervals were recorded continuously during the race (beat-to-beat-modus) using a HR-monitor with a time resolution of 1ms (Polar S810, Polar Electro GmbH, Germany; Kingsley et al., 2005; Nunan et al., 2009). Additionally, blood lactate (La) and blood glucose (Glu) concentration were assessed from each runner immediately (within the first minute) after crossing the finish line and were analyzed using an enzymatic-amperometric method (Super GL ambulance, Dr. Mueller Geraetebau GmbH, Germany; Faude and Meyer, 2008).
HRV AnalysisCollected raw data were transferred to a PC via an infrared interface. The NN intervals were stored as ASCII files for further data analysis. Using Kubios HRV software (Premium Version 3.4.1, Biosignal Analysis and Medical Imaging Group, Kuopio, Finland; Tarvainen et al., 2014), the HRV analysis was conducted on data collected from 5 min every 10% (10-100%) of the race for each runner. Raw data were visually inspected and artefacts were corrected with the Kubios proprietary automatic artefact correction method. Only participants whose data contained artefact rates of <3% were included for analysis. Besides standard HRV indices obtained from time-domain analysis including the mean of normal RR-interval length (meanRR in ms), the total variability as standard deviation of all normal RR-intervals (SDNN in ms) and the root mean square of successive differences (RMSSD in ms; Ciccone et al., 2017), the scaling behavior for assessing correlation properties was calculated using the non-linear short-term scaling exponent DFA-alpha1 (window width: 4 ≤ n ≤ 16 beats). DFA-alpha1 values indicate time series correlation properties, i.e. type of noise: approx. 1.5 for strongly correlated Brownian noise and ≤ 0.5 for uncorrelated white noise with random signals. Approx. 1 signifies a mix of uncorrelated and maximally correlated signal components with 1/f noise (represents a balance between the complete unpredictability (randomness) of white noise and the predictability (strong correlations) of Brownian noise) (Platisa and Gal, 2008).
Statistical analysisThe statistical analysis was performed with SPSS 23.0 (IBM Statistics, USA) for Windows (Microsoft, USA). The Shapiro-Wilk test was applied to verify the Gaussian distribution of the data. The degree of variance homogeneity was verified by the Levene test. Subsequently, to analyze the effects of the exercise bout on dependent variables (HR, HRV parameters) and 1 km split times between measuring intervals 10% (Begin) and 100% (End) paired sample T-tests were applied. For all tests, statistical significance was accepted for p ≤ .05. Cohen’s d was used to denote effect sizes in comparison of the measurement intervals (small effect = 0.2, medium effect = 0.5, large effect = 0.8; Cohen, 1988).
The mean finishing time was 3:10:22 ± 0:17:56 h:min:s (minimum values: 2:43:18 h:min:s; maximum values: 3:33:14 h:min:s) with a blood lactate concentration of 4.04 ± 1.12 mmol/L and a blood glucose concentration of 6.15 ± 1.21 mmol/L at the end of the race. Comparing the beginning to the end segment of the marathon race (Begin vs. End) significant increases with large effect size were found for km split time (p < .001, d = .934) and for HR (p = .010, d = .804), showing higher HR with slower km split times. Significant decreases with large effect size were found for meanRR (p = .013, d = .798) and DFA-alpha1 (p = .003, d = 1.132). There were no significant changes in SDNN (p = .802, d = .072) and RMSSD (p = .188, d = .379). Descriptive values (mean ± standard deviation) of all analyzed parameters during the race (10-100%) are provided in Table 1. In addition, Figure 1 and Figure 2 show the km split times, HR and DFA-alpha1 over time. Figure 3 provides an additional view (km split time, HR, DFA-alpha1, RMSSD and SDNN) of one subject with the time-varying analysis function of Kubios HRV Software (Version 3.4.1).
The aim of the present study was to gain more insight into non-linear HRV dynamics during prolonged endurance exercise competition and to evaluate the potential of the non-linear index DFA-alpha1 as a marker for internal load and complex regulation along with standard linear time-domain measures of HRV. This study provides unique insights in the behavior of both time-domain measures (SDNN and RMSSD) and DFA-alpha1 of HRV over the course of a marathon race. As expected, given the considerable exercise intensity of this event, changes in all three markers were noted. However, SDNN and RMSSD were very low (<5ms) during the whole race and did not show substantial variation during the time course of the race, which is in agreement with previous work that showed the lack of discriminative power of linear HRV indices beyond moderate exercise intensities (Tulppo et al., 1996; Karapetian et al., 2008). This is also in line with studies linking both SDNN and RMSSD as threshold markers signifying the VT1. To determine a corresponding threshold intensity, they required an incremental ramp to display a discernible nadir at the VT1, making them ill-suited to denote internal load intensity in random, untested subjects. In this context, DFA-alpha1 as a dimensionless index of fractal correlation properties and complex regulation has shown the ability to indicate current intensity loads without prior performance level information (Gronwald and Hoos, 2020). Based on previous observations, the VT1 occurs near its midpoint of range therefore making it suitable for a wide range of exercise intensities (Gronwald et al., 2020a). Interestingly, DFA-alpha1 displayed a rather large dynamic range over the course of the marathon, denoting possible fluctuations in internal load during the long distance road race. In regard to the question pertaining to the agreement of external vs. internal load monitoring several observations are of note. In accordance with recent findings (Santos et al., 2006; Myrkos et al., 2020), most of the runners in this study ran a slower second half of the race as displayed in their reduced running speed (positive pacing profile). Despite a slower pace in the second part of the race, DFA-alpha1 declined to values well into those associated with severe intensity domains (Gronwald et al., 2020b). Fluctuation between values of 0.75 and 0.25 seem to depend upon a narrow band of heart rates in a given individual (see Figure 3). In other words, as heart rate fluctuated around a subject’s particular “threshold” level, DFA-alpha1 exhibited changes in the range of uncorrelated and anti-correlated (random) patterns. Although heart rate is a well-established marker of cardiac strain and internal loading, without a predefined knowledge of each individual’s physiologic reference values (HR at lactate thresholds, HR to VO2 relation), defining a particular load level is problematic. Even though it is well known that marathon exercise intensity, measured by an external non-biological load parameter (speed), is performed near the aerobic threshold, it appears that a measure of internal biological load (DFA-alpha1) indicates intensity near maximal physiologic bounds. Speculation as to the cause of these disparate measurements of load (speed vs. HRV derived DFA-alpha1) may be better understood by reviewing behavior of another biologic marker such as blood lactate concentration. Prior studies of lactate measurements taken every 6km in elite marathon runners during a 30km all-out race have shown values from 2.39 mmol/L at 6km to 3.15 mmol/L at 30 km throughout the race (Santos et al., 2006). Therefore, even though a marathon may be performed at or below the conventionally defined intensity of lactate accumulation measured through ramp or stage testing, these “threshold” intensity levels may not hold for multi hour exercise. The present findings seem to support a moderate steady state elevation of lactate but well below the point of what may be considered a maximal steady state (Perrey et al., 2003). In a study in cyclists (Gronwald et al., 2019), DFA-alpha1 measured during a prolonged incremental ramp averaged 0.5 at lactate values near 2mmol/l. In addition, despite lactate not being measured during the actual marathon run in this report, values of blood lactate concentration immediately after the race averaged 4mmol/l, consistent with near maximal steady state values and severe intensity levels. Although further studies are needed to support the possibility of using the wide dynamic range of DFA-alpha1 as a biomarker for submaximal metabolic steady state, both the current study and the previously done incremental ramp testing have indicated this possibility.
LimitationsAlthough the data presented here have been slightly affected by artefacts, very few studies have been conducted on the influence of artefact presence and correction on DFA-alpha1 during exercise so far. Further investigations are needed to determine appropriate cutoffs for artefact and any resultant bias to the DFA-alpha1 index. In addition, only male runners were included in this study, diminishing the generalization to female athletes or participants of various age and ethnic groups. Ideally, lactate could have been measured on a regular basis throughout the marathon but was only obtained immediately post finish. However, doing so would have disrupted the normal procedure of the race and is not practical in a field race situation. The average value of 4mmol/l seen here agrees with that of Santos et al. (2006), and is consistent with severe intensity exercise domains just below the maximal steady state (Faude et al., 2009; Beneke et al., 2011), avoiding exponential rise in blood lactate concentration (Farrell et al., 1979). Finally, the use of a chest belt recording device is often employed in field studies. However, agreement with medical grade ECG recording equipment has not yet been proven for DFA-alpha1 determination during exercise conditions.
A comparison of non-linear vs. linear time-domain HRV indices changes over the course of a marathon race highlight substantial fluctuations in DFA-alpha1 as an indicator of relative internal exercise load and proxy of organismic demands. DFA-alpha1 showed a wide dynamic range throughout the race consisting of both uncorrelated and anti-correlated values indicating dynamic modulation processes during the course of the race. Despite that, both SDNN and RMSSD showed minimal fluctuations and thus were poorly suitable to discriminate the development of fatigue during prolonged high intensity exercise in the field. Lactate as a measure of internal metabolic load was consistent with sustained high intensity exercise when measured at the end of the event. Heart rate was elevated throughout the marathon and increased over time, but without knowing an individual’s baseline VO2 kinetics (or maximum value of HR), it does not provide sufficient information on relative intensity. Evaluation of an external load indicator, running speed showed a gradual slowing especially past the midpoint (positive pacing profile). Despite a reduction of this external load indicator, the DFA-alpha1 continued to fall to values seen in the highest intensity domain during incremental exercise testing in agreement with lactate assessment. Therefore, the discrepancy between the reduced running pace with that of the decline of DFA-alpha1, may demonstrate that there is a complex modulatory dynamics present during the course of the race that may not be detected by conventional linear HRV measurements (Molkkari et al., 2020). Therefore, the dimensionless biomarker DFA-alpha1 may demarcate the complex dynamics of internal load development over the course of a marathon race as a descriptor of NPE (Balagué et al., 2020). Applying these findings to other endurance events and pacing strategies may provide further information on complex modulation during exercise training and intensity distribution for performance enhancement and mitigation against overtraining from inappropriate internal stress.
ACKNOWLEDGEMENTS |
The authors wish to thank the athletes for their participation in the present study. The experiments comply with the current laws of the country in which they were performed. The authors have no conflict of interest to declare. The datasets generated during and/or analyzed during the current study are not publicly available, but are available from the corresponding author who was an organizer of the study. |
|
AUTHOR BIOGRAPHY |
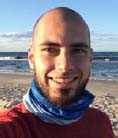 |
Thomas Gronwald |
Employment: Professor for Sports and Exercise Science, Dept. Performance, Neuroscience, Therapy and Health, MSH Medical School Hamburg |
Degree: PhD, MBA |
Research interests: Exercise physiology, load monitoring, diagnostics, injury prevention, HRV |
E-mail: thomas.gronwald@medicalschool-hamburg.de |
|
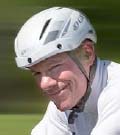 |
Bruce Rogers |
Employment: Department of Medicine, University of Central Florida |
Degree: MD |
Research interests: Exercise physiology, load monitoring, consumer wearables |
E-mail: bjrmd@knights.ucf.edu |
|
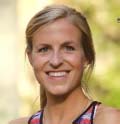 |
Laura Hottenrott |
Employment: Institute of performance diagnostics and health promotion, Martin-Luther-University Halle-Wittenberg |
Degree: Master of Science |
Research interests: Training adaptations, aging,sex-differences, endurance sports, cardiac autonomic control |
E-mail: hottenrott.laura@gmail.com |
|
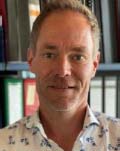 |
Olaf Hoos |
Employment: Professor for Sports and Exercise Science, Director of the Center for Sports and Physical Education of the Julius-Maximilians-University Wuerzburg |
Degree: PhD |
Research interests: Exercise physiology, training monitoring, performance diagnostics, adapted physical activity, heart rate variability |
E-mail: olaf.hoos@uni-wuerzburg.de |
|
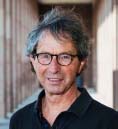 |
Kuno Hottenrott |
Employment: Professor for Sports Science, Martin-Luther-University Halle-Wittenberg |
Degree: PhD |
Research interests: Training adaptation, cardiac autonomic control, performance diagnostic, exercise sciene, sports nutrion |
E-mail: kuno.hottenrott@sport.uni-halle.de |
|
|
|
REFERENCES |
 Balagué N., Hristovski R., Almarcha M.D.C., Garcia-Retortillo S., Ivanov P.C. (2020) Network Physiology of Exercise: Vision and Perspectives. Frontiers in Physiology 11, 611550. Crossref
|
 Beneke R., Leithäuser R.M., Ochentel O. (2011) Blood lactate diagnostics in exercise testing and training. International Journal of Sports Physiology and Performance 6, 8-24. Crossref
|
 Ciccone A.B., Siedlik J.A., Wecht J.M., Deckert J.A., Nguyen N.D., Weir J.P. (2017) Reminder: RMSSD and SD1 are identical heart rate variability metrics. Muscle Nerve 56, 674-678. Crossref
|
 Coen B., Urhausen A., Kindermann W. (2001) Individual anaerobic threshold: methodological aspects of its assessment in running. International Journal of Sports Medicine 22, 8-16. Crossref
|
 Cohen, J.W. (1988) Statistical power analysis for the behavioral sciences.
Hillsdale, NJ: Erlbaum.
|
 Cottin F., Slawinski J., Lopes P., de V., Louw A., Billat V. (2007) Effect of a 24-h continuous walking race on cardiac autonomic control. European Journal of Applied Physiology 99, 245-250. Crossref
|
 Farrell P.A., Wilmore J.H., Coyle E.F., Billing J.E., Costill D.L. (1979) Plasma lactate accumulation and distance running performance. Medicine and Science in Sports 11, 338-344. Crossref
|
 Faude O., Kindermann W., Meyer T. (2009) Lactate threshold concepts. Sports Medicine 39, 469-490. Crossref
|
 Faude O., Meyer T. (2008) Methodische Aspekte der Laktatbestimmung Methodological aspects of lactate determination. Deutsche Zeitschrift für Sportmedizin 59, 305-309.
|
 Franco V., Callaway C., Salcido D., McEntire S., Roth R., Hostler D. (2014) Characterization of electrocardiogram changes throughout a marathon. European Journal of Applied Physiology 114, 1725-1735. Crossref
|
 Gronwald T., Hoos O. (2020) Correlation properties of heart rate variability during endurance exercise: a systematic review. Annals of Noninvasive Electrocardiology 25, e12697. Crossref
|
 Gronwald T., Hoos O., Hottenrott K. (2020b) Influence of performance level of male run-ners on non-linear dynamics of heart rate variability during a 10km race. International Journal of Performance Analysis in Sport 20, 569-583. Crossref
|
 Gronwald T., Hoos O., Ludyga S., Hottenrott K. (2019) Non-linear dynamics of heart rate variability during incremental cycling exercise. Research in Sports Medicine 27, 88-98. Crossref
|
 Gronwald T., Rogers B., Hoos O. (2020a) Fractal correlation properties of heart rate variability: A new biomarker for intensity distribution in endurance exercise and training prescription?. Frontiers in Physiology 11, 550572. Crossref
|
 Jamnick N.A., Pettitt R.W., Granata C., Pyne D.B., Bishop D.J. (2020) An Examination and Critique of Current Methods to Determine Exercise Intensity. Sports Medicine 50, 1729-1756. Crossref
|
 Karapetian G.K., Engels H.J., Gretebeck R.J. (2008) Use of heart rate variability to estimate LT and VT. International Journal of Sports Medicine 29, 652-657. Crossref
|
 Kingsley M., Lewis M.J., Marson R.E. (2005) Comparison of polar 810 s and an ambulatory ECG system for RR interval measurement during progressive exercise. International Journal of Sports Medicine 26, 39-44. Crossref
|
 Molkkari M., Angelotti G., Emig T., Räsänen E. (2020) Dynamical heart beat correlations during running. Scientific Reports 10, 1-9. Crossref
|
 Myrkos A., Smilios I., Kokkinou E.M., Rousopoulos E., Douda H. (2020) Physiological and Race Pace Characteristics of Medium and Low-Level Athens Marathon Runners. Sports 8, 116. Crossref
|
 Nunan D., Donovan G.A.Y., Jakovljevic D.G., Hodges L.D., Sandercock G.R., Brodie D.A. (2009) Validity and reliability of short-term heart-rate variability from the Polar S810. Medicine and Science in Sports and Exercise 41, 243-250. Crossref
|
 Peng C.K., Havlin S., Stanley H.E., Goldberger A.L. (1995) Quantification of scaling exponents and crossover phenomena in nonstationary heartbeat time series. Chaos 5, 82-87. Crossref
|
 Perrey S., Grappe F., Girard A., Bringard A., Groslambert A., Bertucci W., Rouillon J.D. (2003) Physiological and metabolic responses of triathletes to a simulated 30-min time-trial in cycling at self-selected intensity. International Journal of Sports Medicine 24, 138-143. Crossref
|
 Platisa M.M., Gal V. (2008) Correlation properties of heartbeat dynamics. European Biophysics Journal 37, 1247-1252. Crossref
|
 Rogers B., Giles D., Draper N., Hoos O., Gronwald T. (2021) A new detection method defining the aerobic threshold for endurance exercise and training prescription based on fractal correlation properties of heart rate variability. Frontiers in Physiology 11, 596567. Crossref
|
 Santos R.V.T., Almeida A.L.R., Caperuto E.C., Martins E., Rosa L.C. (2006) Effects of a 30-km race upon salivary lactate correlation with blood lactate. Comparative Biochemistry and Physiology 145, 114-117. Crossref
|
 Sztajzel J., Atchou G., Adamec R., de Luna A.B. (2006) Effects of extreme endurance running on cardiac autonomic nervous modulation in healthy trained subjects. The American Journal of Cardiology 97, 276-278. Crossref
|
 Tarvainen M.P., Niskanen J.P., Lipponen J.A., Ranta-Aho P.O., Karjalainen P.A. (2014) Kubios HRV - heart rate variability analysis software. Computer Methods and Programs in Biomedicine 113, 210-220. Crossref
|
 Tulppo M.P., Makikallio T.H., Takala T.E., Seppanen T., Huikuri H. V. (1996) Quantitative beat-to-beat analysis of heart rate dynamics during exercise. American Journal of Physiology 271, H244-H252. Crossref
|
 Zinner, C. and Sperlich, B. (2016) Marathon Running: Physiology, Psychology, Nutrition and Training Aspects. Basel: Springer International Publishing Crossref
|
|
|
|
|
|
|