|
|
|
ABSTRACT |
This study analysed critical movement demands of tennis match-play to better inform contemporary approaches to athlete preparation and training. HawkEye data from matches during the 2021 and 2022 Australian Open were utilised. Distance was aggregated for movement cycles, points, games, sets, and matches, alongside total shots played. Data was collated for males (best-of-five sets) and females (best-of-three sets) allowing comparisons both within and between sexes. Overall, no differences within sexes were evident for total distance, however males traversed further per match than females (MDE = 809 ± 139m, ES = 0.86). Female players travelled further in their deciding (third) sets compared to set 1 (ES = 0.28) and while this effect wasn’t as discernible for males, the deciding (fifth) set showed some evidence of elevated distance requirements and variability. Between sexes, only female set 3 was different to male set 3 (ES = 0.29). Female and male tiebreak games (i.e. game 13) required players travel further distance compared to other games (ES = ~1.45). Between sex differences were observed for tiebreak games compared to games 1 to 12 (female ES = 1.36 and male ES = 1.53). Players from both sexes generally covered similar distances during points and movement cycles, with between-shot distances of 4.2m-4.5m, notably longer than previous reports. Further, total shots and total match distance (r > 0.97; p < 0.01) shared similar linear relationships. These results highlight that the between shot or movement cycle demands of professional hard court tennis are substantially higher than described in the literature (Roetert et al., 2003). The findings also reveal competitiveness as a key influence on set level distance demands during professional tennis match-play, a consideration in player preparation programs. |
Key words:
Professional tennis, movement analysis, movement cycle
|
Key
Points
- Between sex differences are evident for match distance in hard court grand slam tennis, largely owing to the difference in format (i.e. best-of-three sets vs. best-of-five sets).
- No sex differences were present when distance is normalised for number of shots played, supporting differences in total match distance resulting from more shots played by males.
- Deciding sets (third for females and fifth for males) generally feature elevated distance demands, as do tiebreak games.
- The average movement cycle distance on hard courts is 4.5m for males and 4.2m for females, where 80% of movement cycles are ~6m or less.
|
World class professional tennis requires speed, power and agility, coupled with a unique endurance capacity to sustain high intensity efforts whilst making skilful shots over prolonged periods of time (Giles et al., 2018; Kovacs, 2006). Historically, the physical aspects of tennis performance have been critiqued through an assessment of physiology, movement demands, or a combination of both. To date, the majority of literature exploring the movement demands of tennis utilise summary statistics, which details high-level metrics such as distance covered, velocity, and acceleration (Galé-Ansodi et al., 2018;2017a; 2017b; Hoppe et al., 2014; 2016; Kilit and Arslan, 2017; Kovalchik and Reid, 2017; Pereira et al., 2016; 2015; 2017; 2018; Whiteside and Reid, 2017). However, these summary statistics are generally aggregated to represent an entire match or set, leaving a clear gap in the understanding of more specific or technical aspects of movement in match-play (i.e., point or shot movement requirements). Movement in tennis encompasses a coupling of the distance a player is required to travel and the time they have available to cover this distance. A recent interview with expert coaches outlined higher-order themes typically associated with elite movement in tennis (Giles et al., 2018). This perspective suggests that, when posed with similar movement scenarios, players may have different movement solutions that prove equally effective. Furthermore, the physical ability of players is likely intertwined with their playing style, where faster and more enduring players often adopt counterpunching approaches to playing the game (Roetert and Kovacs, 2011). Paradoxically though, much of the sport’s research focus has been on match tactics rather than the movement that allows for those tactics to be implemented. From a training point of view, interest has grown in quantifying the higher order kinematics of player movement, often with load management in mind, but lower order kinematics like distance remain the foundational starting point of designing on-court movement training interventions, such as the star drill or box drill (Reid et al., 2008). Roetert et al. (2003) reported that 2.5m represented 80% of between-shot distance in tennis, but this information is now two decades old and may not be representative of the modern game. This means that training design is regularly based on the observational analysis of experts and/or generalised and dated information describing the distance demands of point play. Therefore, it is critical that the distance requirements of tennis match play are contemporised. Understanding the distance requirements of an entire tennis match is important to guide training and prepare athletes for the demands of tournament match play. Accordingly, Whiteside and Reid (Whiteside and Reid, 2017) have previously reported the cumulative court coverage demands of male and female players over the first week of Grand Slam tennis (Whiteside and Reid, 2017). They revealed that males covered 9647m over four matches (~2400m per match) and females 5656m (~1400m per match) (Whiteside and Reid, 2017). This information is valuable as it informs the expected workload during the first week of a major tournament. Coaches can use these figures to guide pre-season preparation workloads or to cross reference against the data of their own players. However, these aggregated match demands can mask how distance accumulates over sets, games, points, and shots, therein limiting the precision with which coaches can plan training. While practitioners may be able to piece together these demands from different published sources, they are plagued by methodological differences (i.e., performance level, sex, court surface etc.), and therefore, remain incomplete (Pluim et al., 2023). A more forensic examination of distance requirements (i.e., shot, point or game) remains a gap in the literature (Galé-Ansodi et al., 2017a; Pereira et al., 2016; Pluim et al., 2023; Roetert et al., 2003; Whiteside and Reid, 2017), which could guide the design of more representative training sessions and drills. For instance, the most practically referenced work investigating distance travelled in tennis to date, examined between-shot movement of elite male players during the French Open Grand Slam (Roetert et al., 2003). Findings from this work illustrated ~80% of between-shot movement required athletes to travel <2.5m, ~10% of movement between 2.5-4.5m, and ~10% of movement >4.5m, which to the authors’ knowledge, is the only investigation that has examined the sport’s between-shot court coverage or movement cycle requirements, which ironically represent the most rehearsed movement patterns in tennis. However, for all of its potential practical value, the work was limited by the hand notation of distance and a small sample size of male players, which misses 50% of the world's professional playing body and limits the generalisability of the findings. Furthermore, this research explored the distance demands of decades old match-play on clay courts (Roetert et al., 2003), further limiting its utility given the changes in the physicality of the modern game and prevalence of hard court tournament play (Fernandez et al., 2006; Galé-Ansodi et al., 2017a; Pereira et al., 2016; Tech, 2010). Accordingly, a more detailed and contemporary review of these demands is needed, which was the focus of the current study. More specifically, match, set, game, point, and movement cycle distances were compared both within and between sexes during a grand slam on hard courts.
All athletes entering the 2021 or 2022 Australian Open Grand Slam main draw tournament were considered for inclusion in this study. Each year, 128 males and 128 females enter the Australian Open main draw tournament, with 169 and 172 unique males and females respectively, featured in the 2021 and 2022 Australian Open, who were all considered for participation in this study. Of the 169 male and 172 female players, 155 male and 168 female athletes’ data met the inclusion criteria for this study (detailed below). All participants provided consent for their tournament data to be collected, analysed, and publicly documented at the time of entry into the tournament. Ethics approval to conduct this study was provided by an institutional research ethics committee (2022/ET000216). Player tracking data were recorded by Hawk-Eye (Hawk-Eye Innovations Ltd, Basingstoke, UK), which uses a 10-camera system to identify on-court player and ball positioning during tennis matches with a mean error of 2.6mm (for ball tracking), operating at a 25Hz and more recently 50Hz sampling frequencies (Innovations). The Hawk-Eye player tracking accuracy is unpublished, however ball tracking precision alongside single camera analysis systems error of 55mm (Dunn et al., 2014) suggest an expected error comparable to the ball tracking. Hawk-Eye staff collect and process the video camera footage of each match in the Australian Open and provide a raw sampled output of the centre of mass X Y positional coordinates of players during live match play. The resultant data is owned by the tournament owner. For this study, the raw player tracking data, describing the centre of mass X Y positional coordinates for each player, for each match were down sampled to 25Hz for inter-event consistency and smoothed using a Butterworth low pass filter. Once processed, the data were checked for spurious values or errors consistent with tracking drop out. This included identification of data loss during a rally or interpolated data where signal drop-out occurred. Where any errors were identified in a point, the entire point was removed from the match file. The entire match file was included for analysis if >90% of the points were available after checking for errors. Of the 504 male and 506 female match files available for analysis, 371 male and 483 female match files met the inclusion criteria. The movement cycle definition by Margi (Margi, 2020) was used to assess the distance travelled during each movement cycle for each player per rally. However, as HawkEye player tracking does not detect the split-step action, opposition racket-ball impact time was utilised as a surrogate for the player split-step action due to the close timing of the split-step and opposition hitting time (Mecheri et al., 2019). As the movement cycle consists of both travelling to the ball and recovering to a position of leverage for the next shot, the following considerations were made regarding the player tracking data for the movement cycle analysis. To account for variation in distance travelled from the serve, which is typically shorter than general rally plays, all data from the serve was excluded for the movement cycle analysis. Further, as a rally may have no clear end time (i.e. distinct moment when the rally is over), the player tracking data from the last shot of a rally was also excluded from the movement cycle analysis. This meant that data for the movement cycle was captured from the return shot of the serve until the moment of the last shot in a rally. Therefore, if a rally was three shots or less, no movement cycle data was included for that rally. This distance was summarized for each movement cycle, meaning several data points may have existed for each player in the same rally. The total distance a player moved was calculated for each aspect of tennis match-play; this included match, set, game, point, and movement cycle distances for each player during each match. All movement data during live match play were included for the summation of match, set, game and point distances, whereas the movement cycle excluded the serve and final shot data of the rally to ensure a full movement cycle was captured. For match analysis, players’ data were aggregated at each round and all matches from the quarterfinals, semi-finals and grand final were aggregated as “Finals matches”. Further, the total number of shots played was also calculated for each match. Due to data tracking drop out (common when players are deep off the baseline and do not come into Hawkeye video frame) observations of more than four standard deviations (SD) from the mean were removed for each aspect of tennis match-play to account for those observations that may not have included a full data set based on visual inspection of outliers. A series of linear models were used to compare the phases of each aspect of match play within and between sexes (i.e., males Game 1 vs. males Game 2 etc., and males Game 1 vs. females Game 1 etc.). In the first model, distance travelled was the outcome variable, aspect number (i.e. Game 1, Game 2, etc.) was the fixed effect, and player was a random effect. The second model was reflective of the first model, with the addition of sex as a fixed effect. Analysis of variance (ANOVA) between the first two models informed a likelihood ratio p-value to assess the influence of sex on predicted distance for each aspect of match-play. This comparison was conducted for match, set, game, and point distance. The final linear model analysed the movement cycle, with distance travelled as the outcome variable, sex as a fixed effect, and player as a random effect. Moreover, a linear regression model was utilised to investigate total match distance predicted by total shots played, allowing for a comparison of sexes. Visual inspection of model residuals led to a log transformation of the data in each case. Cooks distance was used to further remove any influential outliers, where the top 1% of observations were removed (Cook, 1979). To compare within and between sex differences, 95% confidence intervals (CI) were calculated. True differences were acknowledged where confidence intervals did not overlap between comparisons. To interpret practicality, Cohen’s d effect sizes (ES) were calculated only for those comparisons with true differences, interpreted as small (0.2), medium (0.5), and large (0.8) (Cohen, 1988). For generalised comparisons, a mean difference between estimates (MDE) was calculated and reported as a mean±SD and a mean ES for those comparisons with true differences only. Summary statistics were calculated for full movement cycles for each sex, dissected in ten-percentile increments. All statistical computation was conducted using the R programming language (R version 4.1.3 (2022-03-10)).
Likelihood ratio p-values from the ANOVA model comparisons indicated a significant effect of sex in each aggregated aspect of match-play (shots per point, point, game, set, and match p < 0.01). The estimate (±95% CI) for each aspect of match-play are presented in Figure 1. The average estimate for each aspect of match-play and each sex is presented in Table 1. At the match level, no differences were observed within female matches across the tournament (MDE = 132 ± 157m) or within males’ matches (MDE = 126 ± 150m) with variance ranging between 6-11%. True differences were observed between sexes (MDE = 809 ± 139m, mean ES = 0.86), highlighting males covered ~70% more distance than females in a match. Match estimates are presented in Figure 1A. When investigating sets, a true difference was observed for females’ Set 1 compared to Set 3 (ES = 0.28), where distance was 16% further (81m) in Set 3. For males, no differences were observed between sets (MDE = 34m ± 39m), with a typical variance of 6%. Between sexes, females covered 15% more distance than males in Set 3 (ES = 0.29). Besides Set 3, no further differences were observed between sexes (MDE = 36 ± 63m), with a typical variance of 10-12% evident. Set estimates are presented in Figure 1C. At the Game level, female Game 13 was different to Games 1 to 12 (MDE 67 ± 3m, mean ES = 1.45), where Game 13 distance was 56% greater than the other games. When Game 13 was removed (which represents a tie-break and is a-typical of a normal tennis game), a typical variance of 8% is observed (MDE = 3 ± 4m). For males, Game 13 was also different to Games 1 to 12 (MDE = 67 ± 3m, mean ES = 1.44), where 58% greater distance was evident in Game 13. When Game 13 was removed, a typical variance of 7% was evident (MDE = 3 ± 4m). Several differences were observed between sexes, with females’ Game 13 being higher than males’ Games 1 to 12 by ~55% (MDE = 63 ± 3m, mean ES = 1.36); further, males’ Game 13 being higher than female Games 1 to 12 by~57% (MDE = 71 ± 3m, mean ES = 1.53). When removing Game 13, the variation between games was <10% (MDE = 4 ± 4m). Model estimates for games are presented in Figure 1D. At the point level, no differences were observed within female (MDE = 1 ± 2m) or male (MDE = 3 ± 3m) points, with variance ranging between 14-31%. Point distance between sexes were not different (MDE = 2 ± 3m), despite males traveling ~16% further than females per point. Point estimates are presented in Figure 1E. A linear relationship between distance and shots played was evident (see Figure 1F). The addition of one shot per point results in 2 ± 1m (7%) and 2 ± 2m (7%) more distance for female and male players, respectively. When shots played per point were matched (i.e. males play 5 shots and females play 5 shots in a point), no differences between sexes are observed (MDE = 1 ± 1m), with a typical variance of 3% evident. Regression analysis of total match distance by total shots played are reported in Figure 1B. No meaningful differences were observed between sexes. The relationship between shots played and total match distance was near perfect for both sexes (male r = 0.98; p < 0.01; female: r = 0.97; p < 0.01). Regression equations are presented in Figure 1B, which are almost identical between sexes. No true differences between sexes were evident in the movement cycle, as overlap between sex confidence intervals existed (see Table 1). Males travelled 0.3m further than females, equating to an average of 7% further per cycle. A distribution plot of the movement cycle is presented in Figure 2.
The purpose of this investigation was to compare the distance demands of movement cycles, points, games, sets, and matches during elite professional hard court match-play both within and between sexes. The evaluation of these demands with a cohort in near identical conditions directly addresses the disconnected view of court coverage previously presented in the literature (Galé-Ansodi et al., 2018; 2017a; 2017b; Hoppe et al., 2014; 2016; Kilit and Arslan, 2017; Kovalchik and Reid, 2017; Pereira et al., 2016; 2015; 2017; Whiteside and Reid, 2017). Male professional players have been reported to cover more distance than their female counterparts in Grand Slam hard court match-play (Whiteside and Reid, 2017). A similar trend was observed in the current study where males travelled ~2.4km and females ~1.4km per match, in line with past research (Pereira et al., 2017; Whiteside and Reid, 2017). This contrast in movement demands between sexes would, to some extent, appear to support the perception that men’s tennis is more physically demanding (Kovalchik and Reid, 2017), and when tallied across an entire (7 match) Grand Slam event, necessitates that male players travel 60% more or ~5.5km longer distances than their female counterparts. This difference is largely explained by the five rather than three set format of men’s matches. These statistics provide rationale for longer endurance type training sessions (McGowan, 2022). However, coaches also regularly prescribe tennis-specific drills (Murphy et al., 2014), where further evaluation of the movement cycle can enhance drill specificity. Beyond format, another factor that has been purported to influence the court covered in a match is competitiveness or the closeness of the contest (Whiteside and Reid, 2017). Interestingly, the current study observed more court coverage in the final sets of both male and female matches that “go the distance” (i.e., five and three setters respectively). This might reaffirm the relationship between competitiveness and physicality of contests, or alternatively, could indicate a change in playing behaviour where players adopt tactics that increase rally length, which is highly correlated with distance travelled (Figure 1B). Tie-break games also feature elevated distance demands, further underlining the link between competitive matches and distance travelled. Both deciding sets and tie-breaks are critical phases of match play, where match outcomes are decided. The practical applications from understanding the physical characteristics of these critical high-pressure moments can allow coaches to program similar scenarios in training, heightening the physical representativeness of training design even if the mental characteristics of match play are hard to recreate (Fleming et al., 2023). As grand slam tournaments progress, higher ranked players are more likely to meet head-to-head. Variance in total match distance also increases as the tournament progresses, most notably in round 4 and finals matches (Figure 1A). This variance may be influenced by other factors such as playing style, specific player match ups, or opposition familiarisation. As an example, two players who regularly compete against each other (e.g., Djokovic and Nadal, who have played 59 times (ATP, 2022)) are often very familiar with each other’s strengths and weaknesses, which may lead to more competitive contests. In contrast, a player can have a breakout tournament in a Grand Slam, where a proven champion could end their campaign in a less competitive affair. These two scenarios may present in the finals of a Grand Slam and explain the variance in total match distance observed in finals matches. Another novel aspect of this investigation was the analysis of between shot movement, or movement cycles, which were observed to last ~4.5m on average. Notation of Grand Slam matches at Roland Garros from two decades ago suggested that ~80% of between-shot movement was <2.5m, ~10% was between 2.5m and 4.5m, and ~10% of movement saw players cover >4.5m between shots (Roetert et al., 2003). This seminal work is the only scientific reference to between-shot movement, and the breakdown of distance demands continues to inform practice today, where coaches consider movement in three ‘ranges’ often termed inner, mid, and end range. The current data present a more contemporary view of the game, especially on hard courts and for both males and females. Less than 10% (~5% for male and ~7% for female) of movement cycles were observed to span <2.5m, which is proportionally reflective of one eighth of that previously observed (Roetert et al., 2003). The remaining 2.5-4.5m (~44% for male and ~49% for female) and >4.5m (51% for male and 44% for female) distance bands were also proportionally 4-5 times higher in the current results (Roetert et al., 2003). Additionally, 80% of movement cycles were <2.5m in the male game historically, that figure now stands at <6m for both sexes. These disparate profiles are likely the result of the evolving physical, technical, and tactical nature of the game and its athletes (Roetert et al., 2003; Tech, 2010). Practically, the current findings are critical in allowing coaches to recalibrate their training design so that drills are more representative of the modern game’s demands (Edwards, 2010). With immediate effect, coaches should update any training logic based on the previous guide that 80% of movement cycles cover <2.5m. This research clearly shows that for hard court tennis, players travel ≤6m between 80% of shots. Furthermore, traditional movement tests to profile the athleticism of tennis players (i.e., 20m sprints, 5-0-5 tests) might be adjusted to better reflect the sport’s movement demands (i.e., 3-0-3 or 10-0-10). Indeed, these findings provide cause to consider whether the inner, mid, and end range categories of movement are still relevant or whether the movement cycles of the contemporary hard-court game are better and more simply served described as ≤4.5m and >4.5m (i.e. inner and extended range). Alternatively, given the complex and unpredictable nature of tennis play, the sport’s movement demands may be better considered as a continuum. In any event, this baseline understanding might pave the way for more individualised approaches to movement analysis for players or future work to consider the intensity of movement. In these contexts, the action capabilities of players as well as the situational demands of point play can ultimately take centre stage.
This study has illustrated professional players court coverage in contemporary Grand Slam tennis. Notable differences, largely attributable to match format, emerged in the movement demands of male and female players. Competitiveness of contests also elevate distance demands, with deciding sets observed to enhance distance coverage. Significantly, the typical movement cycle of players on hard courts was reported as at least two times longer than previously contemplated, which challenges current conventions and presents opportunities for training design.
ACKNOWLEDGEMENTS |
The data that support the findings of this study are available from the corresponding author [CA] upon reasonable request. The authors have no conflicts of interest. The present study complies with the current laws of the country in which it was performed. |
|
AUTHOR BIOGRAPHY |
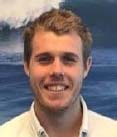 |
Cameron Armstrong |
Employment: PhD Student, School of Human Sciences (Exercise and Sport Science) The University of Western Australia, Perth, Australia |
Degree: MSc |
Research interests: Player tracking and wearable technology in sport, biomechanics, and physiology in sports performance |
E-mail: Cameron.armstrong@uwa.edu.au |
|
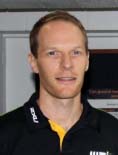 |
Peter Peeling |
Employment: School of Human Sciences at the University of Western Australia, Director of Research at the Western Australian Institute of Sport |
Degree: PhD |
Research interests: Sports performance, sports nutrition, environmental stress |
E-mail: peter.peeling@uwa.edu.au |
|
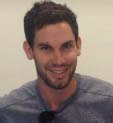 |
Alistair Murphy |
Employment: Youth Development and Applied Science Manager at Tennis Australia |
Degree: PhD |
Research interests: Training load management in youth development tennis players |
E-mail: AMurphy@tennis.com.au |
|
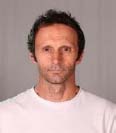 |
Machar Reid |
Employment: Head of Innovation at Tennis Australia |
Degree: PhD |
Research interests: Biomechanics and technology applications in sport |
E-mail: mreid@tennis.com.au |
|
|
|
REFERENCES |
 ATP. (2022) Novak Djokovic vs Rafael Nadal ATP Head to Head, Vol. 2023.
Available from URL: http://www.atptour.com/en/players/atphead-2-head/novak-djokovic-vs-rafael-nadal/D643/N409
|
 Cohen, J. (1988) Statistical Power Analysis for the Behavioral Sciences. 2nd Edition edition. New York: Routledge.
|
 Cook R.D. (1979) Influential Observations in Linear Regression. Journal of the American Statistical Association 74, 169-174. Crossref
|
 Dunn M., Haake S., Wheat J., Goodwill S. (2014) Validation of a Single Camera, Spatio-temporal Gait Analysis System. Procedia Engineering 72, 243-248. Crossref
|
 Edwards, W.H. (2010) Motor Control and Learning. Wadsworth, Cengage Learning: Yolanda Cossio.
|
 Fernandez J., Mendez-Villanueva A., Pluim B. (2006) Intensity of Tennis Match Play. British Journal of Sports Medicine 40, 387-391. Crossref
|
 Fleming J., Field A., Lui S., Naughton R.J., Harper L.D. (2023) The Demands of Training and Match-Play on Elite and Highly Trained Junior Tennis Players: A Systematic Review. Internation Journal of Sports Science and Coaching 18, 1365-1376. Crossref
|
 Galé-Ansodi C., Castellanlo J., Usabiaga O. (2018) Differences Between Running Activity in Tennis Training and Match-Play. International Journal of Performance Analysis in Sport 18. Crossref
|
 Galé-Ansodi C., Castellano J., Usabiaga O. (2017a) Effects of Different Surfaces in Time-Motion Characteristics in Youth Elite Tennis Players. International Journal of Performance Analysis in Sport 16, 860-870. Crossref
|
 Galé-Ansodi C., Castellano J., Usabiaga O. (2017b) More Acceleration and Less Speed to Assess Physical Demands in Female Young Tennis Players. International Journal of Performance Analysis in Sport 17, 872-884. Crossref
|
 Giles B., Peeling P., Dawson B., Reid M. (2018) How do Professional Tennis Players Move? The Perceptions of Coaches and Strength and Conditioning Experts. Journal of Sports Sciences 37, 726-734. Crossref
|
 Hoppe M., Baumgart C., Bornefeld J. (2014) Running Activity Profile of Adolescent Tennis Players During Match Play. Pediatric Exercise Science 26, 281-290. Crossref
|
 Hoppe M., Baumgart C., Freiwald J. (2016) Do Running Activities of Adolescent and Adult Tennis Players Differ During Play?. International Journal of Sports Physiology and Performance 11, 793-801. Crossref
|
 Innovations, H. (N.D.) Electronic Line Calling Technology - How It
Works. Available from URL:
http://resources.platform.pulselive.com/HawkEye/document/20
16/08/15/1e6cdaa4-2b70-4975-b722-
7dca82b8e546/ELC_How_it_Works.pdf
|
 Kilit B., Arslan E. (2017) Physiological Responses and Time-Motion Characteristics of Young Tennis Players: Comparison of Serve vs. Return Games and Winners vs. Losers Matches. International Journal of Performance Analysis in Sport 17, 684-694. Crossref
|
 Kovacs M. (2006) Applied Physiology of Tennis Performance. British Journal of Sports Medicine 40, 381-386. Crossref
|
 Kovalchik S., Reid M. (2017) Comparing Matchplay Characteristics and Physical Demands of Junior and Professional Tennis Athletes in the Era of Big Data. Journal of Sports Science and Medicine 16, 489-497. Crossref
|
 Margi, G. (2020) Movement Cycle. In: Global Agent Academy, Vol.
2022. Available from URL:
https://www.globalagenttennis.com/en/movement-cycle/
|
 McGowan, M. (2022). Australian Open 2022: Davis Cup Assistance
Coach Jaymon Crabb Pinpoints When Alex de Minaur's Form
Revival Started, Vol. 2022, The Australian. Available from
URL: http://www.theaustralian.com.au/breakingnews/australian-open-2022-davis-cup-assistant-coach-jaymoncrabb-pinpoints-when-alex-de-minaurs-form-revivalstarted/news-story/2938f5f18c5af3081dea3fdae9012590
|
 Mecheri S., Laffaye G., Triolet C., Leroy D., Dicks M., Choukou M., Benguigui N. (2019) Relationship Between Split-Step Timing and Leg Stiffness in World-Class Tennis Players When Returning Fast Serves. Journal of Sports Sciences 37, 1962-1971. Crossref
|
 Murphy A.P., Duffield R., Kellett A., Reid M. (2014) A Descriptive Analysis of Internal and External Loads for Elite-Level Tennis Drills. International Journal of Sports Physiology and Performance 9, 863-870. Crossref
|
 Pereira L., Freitas V., Moura F., Aoki M., Loturco I., Nakamura F. (2016) The Activity Profile of Young Tennis Athletes Playing on Clay and Hard Courts: Preliminary Data. Journal of Human Kinetics 50, 211-218. Crossref
|
 Pereira L., Freitas V., Moura F., Urso R., Loturco I., Nakamura F. (2015) Match Analysis and Physical Performance of High-Level Young Tennis Players in Simulated Matches: A Pilot Study. Journal of Athletic Enhancement 4. Crossref
|
 Pereira T., Nakamura F., Jesus M., Vieira C., Misuta M., de Barros R., Moura F. (2017) Analysis of the Distances Covered and Technical Actions Performed by Professional Tennis Players During Official Matches. Journal of Sports Sciences 35, 361-368. Crossref
|
 Perri T., Norton K., Bellenger C., Murphy A. (2018) Training Loads in Typical Junior-Elite Tennis Training and Competition: Implications for Transition Periods in a High-Performance Pathway. International Journal of Performance Analysis in Sport. Crossref
|
 Pluim B., Jansen M., Williamson S., Berry C., Camporesi S., Fagher K., Heron N., Janse Van Rensburg C., Moreno-Perez V., Murray A., O'Connor S., de Oliveira F., Reid M., van Reijen M., Saueressig T., Schoonmade L., Thornton J., Webborn N., Arden C. (2023) Physical Demands of Tennis Across the Different Court Surfaces, Performance Levels and Sexes: A Systematic Review with Meta-Analysis. Sports Medicine. Crossref
|
 Reid M., Duffield R., Dawson B., Baker J., Crespo M. (2008) Quantification of the Physiological and Performance Characteristics of On-Court Tennis Drills. British Journal of Sports Medicine 42, 146-151. Crossref
|
 Roetert, P. and Kovacs, M. (2011) Tennis Anatomy. Human Kinetics, Inc.
|
 Roetert, P., Ellenbecker, T. and Chu, D. (2003) Movement in Tennis. In:
ITF Strength and Conditioning for Tennis. Eds: Reid, M., Quinn,
A. and Crespo, M. London: ITF Ltd. London. 167-175.
|
 Tech, T. (2010) How Tennis Has Evolved Over the Last Two Decades
Part 2, Vol. 2021, Bleacher Report. Available from URL:
http://bleacherreport.com/articles/542410-how-tennis-hasevolved-over-the-last-20-years-part-2
|
 Whiteside D., Reid M. (2017) External Match Workloads During the First Week of Australian Open Tennis Competition. International Journal of Sports Physiology and Performance 12, 756-763. Crossref
|
|
|
|
|
|
|