|
|
|
ABSTRACT |
OpenPose-based motion analysis (OpenPose-MA), utilizing deep learning methods, has emerged as a compelling technique for estimating human motion. It addresses the drawbacks associated with conventional three-dimensional motion analysis (3D-MA) and human visual detection-based motion analysis (Human-MA), including costly equipment, time-consuming analysis, and restricted experimental settings. This study aims to assess the precision of OpenPose-MA in comparison to Human-MA, using 3D-MA as the reference standard. The study involved a cohort of 21 young and healthy adults. OpenPose-MA employed the OpenPose algorithm, a deep learning-based open-source two-dimensional (2D) pose estimation method. Human-MA was conducted by a skilled physiotherapist. The knee valgus angle during a drop vertical jump task was computed by OpenPose-MA and Human-MA using the same frontal-plane video image, with 3D-MA serving as the reference standard. Various metrics were utilized to assess the reproducibility, accuracy and similarity of the knee valgus angle between the different methods, including the intraclass correlation coefficient (ICC) (1, 3), mean absolute error (MAE), coefficient of multiple correlation (CMC) for waveform pattern similarity, and Pearson’s correlation coefficients (OpenPose-MA vs. 3D-MA, Human-MA vs. 3D-MA). Unpaired t-tests were conducted to compare MAEs and CMCs between OpenPose-MA and Human-MA. The ICCs (1,3) for OpenPose-MA, Human-MA, and 3D-MA demonstrated excellent reproducibility in the DVJ trial. No significant difference between OpenPose-MA and Human-MA was observed in terms of the MAEs (OpenPose: 2.4° [95%CI: 1.9-3.0°], Human: 3.2° [95%CI: 2.1-4.4°]) or CMCs (OpenPose: 0.83 [range: 0.99-0.53], Human: 0.87 [range: 0.24-0.98]) of knee valgus angles. The Pearson’s correlation coefficients of OpenPose-MA and Human-MA relative to that of 3D-MA were 0.97 and 0.98, respectively. This study demonstrated that OpenPose-MA achieved satisfactory reproducibility, accuracy and exhibited waveform similarity comparable to 3D-MA, similar to Human-MA. Both OpenPose-MA and Human-MA showed a strong correlation with 3D-MA in terms of knee valgus angle excursion. |
Key words:
Artificial intelligence, Human pose estimation, landing, marker-less, human detection, 3D motion analysis
|
Key
Points
- This study evaluated the accuracy of artificial intelligence-based motion analysis employed the OpenPose algorithm (OpenPose-MA) compared with human visual detection-based motion analysis (Human-MA) with reference to three-dimensional motion analysis (3D-MA).
- No significant difference between OpenPose-MA and Human-MA was observed in terms of the mean absolute error (AI: 2.4° [95%CI: 1.9-3.0°], Human: 3.2° [95%CI: 2.1-4.4°]) of the knee valgus angles.
- The Pearson’s correlation coefficients of OpenPose-MA and Human-MA relative to that of 3D-MA were 0.97 and 0.98, respectively.
- This study revealed that OpenPose-MA exhibited satisfactory accuracy compared with 3D-MA, similar to the conventional Human-MA.
- Compared with conventional motion analysis, OpenPose-MA affords great advantages in terms of time-consumption and cost-saving.
|
Motion analysis serves as a valuable clinical assessment tool, playing a crucial role in functional evaluation, treatment assessment, and sports injury risk screening (Bonnette et al., 2020; Harato et al., 2022; Akbari et al., 2023). It finds application in various scenarios, such as gait analysis in clinical settings (Chester et al., 2005; Baker, 2006) and drop vertical jump (DVJ) tests in sports (Ford et al., 2007; Harato et al., 2022). The DVJ test, in particular, has proven effective in predicting anterior cruciate ligament (ACL) injuries in the knee (Hewett et al., 2005), which are significant traumatic sports injuries, particularly among female athletes (Noyes et al., 2005; Peebles et al., 2020). The DVJ test is extensively employed as a screening tool for sports injuries and for evaluating the efficacy of preventive exercises across various sports domains (Noyes et al., 2005; Hewett et al., 2010; Bonnette et al., 2020). Motion analysis has recently gained prominence as an indispensable assessment tool for musculoskeletal disorder treatment and sports injury prevention. To date, optical camera-based three-dimensional (3D) motion analysis systems have been considered the most accurate and reliable methods (Everaert et al., 1999; Chester et al., 2005). However, they come with certain limitations. Firstly, they necessitate expensive equipment and software, making them financially burdensome. Secondly, the experimental and measurement space is often restricted, which poses constraints on the range of motion that can be analyzed. Moreover, technical expertise is required to operate and analyze data from these systems, making them time-consuming. Another drawback of 3D motion analysis is the manual placement of markers on the subject’s skin to serve as anatomical landmarks. Even slight deviations in marker placement can lead to significant errors in joint angle calculations, as it affects the setting of joint coordinate systems (Piazza and Cavanagh, 2000; Cappozzo et al., 2005). The accuracy of the assessment relies heavily on the examiner’s skill, necessitating well-trained individuals to perform these measurements. Consequently, despite its usefulness, the widespread adoption of 3D motion analysis in clinical and sports settings is challenging. As an alternative to three-dimensional (3D) motion analysis, two-dimensional (2D) motion analysis is frequently utilized. This method involves analyzing joint angles projected onto a single plane, typically the sagittal and/or frontal plane, using general video images. Due to the convenience of capturing videos using digital cameras or smartphones, 2D motion analysis is widely employed in clinical (Ishida et al., 2023) and sports settings (Rabin et al., 2018). However, extracting kinematic data from video images in 2D motion analysis is a time-consuming process prone to human error. Each frame of video footage requires manual identification of joint center points through human detection, which involves substantial time and repetitive work. For instance, analyzing three trials of a typical 10-second video (at a 60 Hz sampling frequency) would entail manually identifying human joint points for 1800 video frames. This process alone would take at least 300 minutes, assuming one frame is processed every 10 seconds. Moreover, human detection procedures may introduce errors, variabilities, and biases (Hensley et al., 2022). Consequently, while 2D motion data can be easily captured on video, it presents limitations in clinical applications due to time-consuming data processing and potential errors in human detection. In recent years, artificial intelligence (AI) has made remarkable advancements, particularly in deep learning using machine learning technology, significantly enhancing the accuracy of feature detection. OpenPose, an open-source framework, enables real-time 2D multi-person keypoint detection and posture estimation (Cao et al., 2019). It is widely recognized as one of the leading AI-based motion analysis methods (Li et al., 2021; Yamamoto et al., 2021; Fan et al., 2022; Ino et al., 2023; Ishida et al., 2024). OpenPose has demonstrated excellent reliability (ICCs = 0.92-0.96) and significant correlations compared to 3D motion analysis systems (R2 = 0.26-0.83) in assessing lower limb joint angles and trunk angle during a double-leg squat (Ota et al., 2020). Hence, AI-based automated motion analysis holds potential in addressing the limitations of traditional 3D and 2D motion analysis methods concerning cost, time, efficiency, and accuracy. However, the specific error range associated with high-velocity movements such as the drop vertical jump (DVJ) has yet to be reported in the literature (D’Antonio et al., 2021; Ota et al., 2021; Ota et al., 2020). Furthermore, it remains unclear whether OpenPose-based motion analysis (OpenPose-MA) is more accurate than conventional human-based motion analysis (Human-MA). If OpenPose-MA proves to be as accurate as or more accurate than Human-MA, it can provide a significant solution for enhancing the data processing efficiency of conventional 2D motion analysis. Therefore, the objective of this study was to compare the accuracy of OpenPose-MA with that of Human-MA and establish the validity of OpenPose-MA using three-dimensional motion analysis (3D-MA) as a reference. We hypothesize that OpenPose-MA and Human-MA will exhibit comparable levels of accuracy within an acceptable error range.
ParticipantsA total of 21 healthy young participants were enrolled in this study, consisting of 10 men and 11 women. The participants had an average age of 20.7 ± 1.0 years, height of 165.2 ± 10.6 cm, mass of 59.6 ± 12.1 kg, and body mass index of 21.6 ± 2.6 kg/m2. None of the participants had any medical conditions affecting physical activity or trunk/lower-extremity disorders/injuries within the 12 months preceding the study. In a pilot study involving seven participants, the effect size was calculated from the mean error and standard deviation (SD) of both OpenPose-MA and Human-MA, yielding a value of 0.65. Based on this effect size (dz), an alpha error level of 0.05, and a statistical power of 0.80, the sample size required to detect a significant difference using a paired t-test was determined to be 21 (calculated using G*Power software ver. 3.1.9.2) (Faul et al., 2007; Faul et al., 2009). Hence, a total of 21 participants were recruited for the study. The Institutional Review Board of Faculty of Health Sciences of Hokkaido University approved this study (IRB protocol number: 19-70-1). Written informed consent was obtained from each participant prior to their involvement in the study.
TaskThe bilateral drop vertical jump (DVJ) task was selected as the task for this study due to its use as a screening test for anterior cruciate ligament (ACL) injury risk (see Figure 1) (Hewett et al., 2005). During the DVJ task, participants began on top of a 30 cm box with their feet positioned shoulder-width apart. They were instructed to drop off the box and execute a maximal vertical jump with both arms raised, simulating a basketball rebound. A successful DVJ was defined as a drop-off without jumping, a bilateral landing, an immediate subsequent jump, and maintaining vertical balance throughout the trial. The DVJ task has been widely employed to assess lower-extremity kinematics and kinetics, demonstrating high within-session reliability with a mean intraclass correlation coefficient of 0.90 and 95% confidence intervals (CI) ranging from 0.86 to 0.95 (Ford et al., 2007). Each participant performed three successful trials of the DVJ task after completing a self-selected warm-up and several practice trials. The trials were recorded for subsequent analysis.
Measurement system and equipmentFor both OpenPose-MA and Human-MA, video image data were acquired using a high-speed digital video camera (Bonita Video 720C, Vicon Motion Systems Ltd., Oxford, England) with a sampling frequency of 120 Hz. The camera was positioned perpendicular to the frontal plane of the knee joint, 30 cm above the floor, at the same height as the surface of the 30-cm box, and 300 cm away from the landing point. It was securely mounted on a separate floor plate to minimize any vibrations resulting from the impact of landing. In all trials, a 30 cm box (40 cm × 80 cm) and two force plates (40 cm × 60 cm each) were arranged side by side. The landing zone for the DVJ was defined as within 40 cm forward of the box edge (Figure 1). During the recording, the camera captured anterior views of the participants' foreheads to ensure accurate visualization of the motion. To ensure the validity of the measurements, simultaneous 3D motion analysis (3D-MA) was conducted alongside the video measurements using the Vicon Nexus 2.10 system (Vicon Motion Systems Ltd., Oxford, England). The Vicon motion system consisted of 14 optical cameras (Eight MX T10-S, Six Vero v2.2; Vicon Motion Systems Ltd., Oxford, England) with a sampling frequency of 120 Hz and two force plates (OR6, Advanced Mechanical Technology Inc., Watertown, NY, USA) with a sampling frequency of 1200 Hz, one for each foot during landing. Following the Vicon Plug-in-Gait marker placement protocol, a total of 34 reflective markers were attached to specific anatomical landmarks on each participant. These markers were placed on the 7th cervical vertebra, 10th thoracic vertebra, clavicle, sternum, right scapula, and bilaterally on the front head, back head, shoulder, elbow, thumb-side wrist, pinkie side wrist, head of the second metacarpal, anterior superior iliac spine, posterior superior iliac spine, lateral thigh, lateral and medial femoral epicondyle, lateral shank, lateral and medial malleolus, second metatarsal head, and calcaneus. Before the motion analysis trials, a static trial was conducted with each participant assuming a neutral standing position. This trial helped align the participants with the global laboratory coordinate system and establish their local joint coordinates. Aligning the local joint coordinates with the standing position of each participant allowed for better control of inter-participant variation in anatomical alignment, particularly the zero-position valgus alignment, during the subsequent motion analysis trials.
OpenPoseOpenPose is a framework for real-time multi-person 2D pose estimation, utilizing a deep learning approach (Cao et al., 2019). This system detects and estimates the posture of individuals in images and movies, identifying major human body joints in two-dimensional space. OpenPose employs an architecture based on Convolutional Neural Networks (CNNs). The model learns the spatial relationships between different parts of the human body based on the concept of "Part Affinity Fields" (PAF). PAFs function as vector fields indicating the direction of body parts, used for accurately linking different human body parts. The model consists of a multi-stage CNN that simultaneously performs detection of body key points and the association between the key points. The training process of OpenPose is designed using a large volume of labeled image data, enabling the model to learn the key points of the human body and their interrelations. Training initially focuses on key point detection, followed by learning the PAFs to capture the spatial relations between these key points. Training data utilized publicly available large-scale human pose datasets, such as the MPII human multi-person dataset (Andriluka et al., 2014) and the COCO key point challenge dataset (Lin et al., 2014). These datasets include images from various scenarios containing real-world challenges like crowds, scale variation, occlusions, and contacts.
Data processingThe OpenPose-MA tool, specifically OpenPose version 1.7.0 (Cao et al., 2017; Hidalgo et al., 2019), was utilized to estimate the positions of joint centers in each frame of the video. In the case of Human-MA, a skilled analyst visually determined the positions of the hip, knee, and ankle joint centers using Frame-Dias V software (DKH Inc., Tokyo, Japan). The OpenPose results were blinded to the analyst responsible for this Human-MA. Both OpenPose-MA and Human-MA relied on the same video images captured from a single plane. To eliminate the impact of lens distortion resulting from the video camera, video distortion was corrected using spatial calibration information obtained from the 3D-MA system (Figure 2). Lens distortion correction was conducted by calibrating the optical motion analysis cameras using a T-shaped wand with optical markers. A high-speed camera synchronized to the motion analysis system was calibrated simultaneously. Each calibration was used to capture 2,000 and 1,000 frames of the wand, respectively. Finally, using the spatial information obtained during calibration, any distortion in the video footage was corrected using the Vicon Nexus 2.10 system (Vicon Motion Systems Ltd., Oxford, England), and then exported as a video file. This correction enabled the extraction of identification errors exclusively for both OpenPose-MA and Human-MA in this study. The trajectories of joint positions were subjected to filtering using a fourth-order Butterworth low-pass filter with zero-lag and a cutoff frequency of 6 Hz. On the two-dimensional plane obtained, coordinates for the hip, knee, and ankle joint centers were estimated, and subsequently, the knee valgus angle was calculated using equation (1). In this equation, θ represents the knee valgus angle, while (x1, y1) and (x2, y2) denote the coordinates of the hip joint and ankle joint, respectively, with the knee joint serving as the origin (Figure 3). The neutral position of the knee valgus-varus angle was defined as zero, with the negative direction indicating a valgus angle.
 |
(1) |
In the reference assessment using 3D-MA, the coordinates of the hip, knee, and ankle joint centers were determined based on the marker coordinates obtained from the markers placed on the participants' body (Plug-in Gait Reference Guide, 2016). The method for calculating the knee valgus angle using these joint center coordinates was the same as the one employed in the video analysis. The trajectories of the markers were also filtered using a zero-lag, fourth-order Butterworth low-pass filter with a cutoff frequency of 6 Hz, similar to the filtering applied in the video analysis. This ensured consistency in the processing of the marker data and facilitated a reliable comparison between the video analysis and the reference 3D-MA. We also estimated the center of gravity (COG) using the Plug-in Gait method (Plug-in Gait Reference Guide, 2016). These data were processed using the Vicon Nexus 2.10 software (Vicon Motion Systems Ltd., Oxford, England).
Data analysis and statisticsThe analysis interval for knee valgus angles spanned from the moment of initial contact to leaving the ground after landing on the DVJ. The landing phase was identified based on the vertical component of the ground reaction force, which reached or exceeded 20N. Within this interval, the following parameters were calculated for knee valgus angles: 1) knee valgus angle at initial contact, 2) knee valgus angle at the lowest COG after landing, and 3) excursion of the knee valgus angle from initial contact to the lowest COG. The method used to calculate the excursion was to subtract the valgus angle at initial contact from the knee valgus angle at the lowest COG. These parameters were calculated separately for males and females, considering the reported sex-based differences in DVJ landing patterns (Peebles et al., 2020; Kawaguchi et al., 2021). To verify the reproducibility of knee valgus angle across the DVJ trials, the intraclass correlation coefficient (ICC) (1, 3) and its 95% confidence intervals (95%CI) were calculated for OpenPose-MA, Human-MA and 3D-MA. The ICC is interpreted as follows (Fleiss, 1986): ICC ≥ 0.75 (excellent), 0.4 ≤ ICC < 0.75 (fair to good), ICC < 0.4 (poor). To assess the accuracy of OpenPose-MA and Human-MA compared to 3D-MA, Pearson’s correlation coefficient was calculated between the knee valgus angles obtained from OpenPose-MA/Human-MA and those obtained from 3D-MA. Additionally, the mean absolute error (MAE) with a 95% CI was calculated for both OpenPose-MA and Human-MA using the 3D-MA results as a reference. The interpretation of absolute error (AE) was categorized into different ranges: AE ≤ 2° (good accuracy), 2° < AE ≤ 5° (acceptable accuracy), 5° < AE ≤ 10° (tolerable accuracy), and AE > 10° (unacceptable accuracy) (McGinley et al., 2009; Bessone et al., 2022), based on previous studies. Furthermore, the coefficient of multiple correlation (CMC) was analyzed to compare the waveform pattern similarity (Ferrari et al., 2010a) between OpenPose-MA/Human-MA and 3D-MA. CMC values were interpreted as follows: 0.65-0.75 (moderate), 0.75-0.85 (good), 0.85-0.95 (very good), and 0.95-1 (excellent) (Ferrari et al., 2010b). A two-way analysis of variance (ANOVA) was performed to evaluate the effects of measurement method, sex, and their interaction on the knee valgus angle. Unpaired t-tests were conducted to compare MAEs, Pearson's correlation coefficients, and CMCs between OpenPose-MA and Human-MA. The statistical significance level was set at p < 0.05, and IBM SPSS Statistics version 22.0 (IBM Corporation, Armonk, NY, USA) was used for the statistical analyses.
AccuracyThe ICCs (1,3) and their 95%CIs for each knee valgus angles during DVJ are shown in Table 1. The ICCs (1,3) for OpenPose-MA were 0.86 to 1.00 (p < 0.001), Human-MA were 0.88 to 1.00 (p < 0.001) and 3D-MA were 0.91 to 1.00 (p < 0.001), indicating "excellent" re-producibility of the DVJ trial (Table 1). The analysis revealed no significant differences in knee valgus angles during landing among OpenPose-MA, Human-MA, and 3D-MA. No interaction effect was detected between the measurement method and sex. However, a significant gender difference was observed, with female participants exhibiting significantly larger knee valgus angles at initial contact, at the lowest COG, and excursions than male participants (Table 2). Strong and significant correlations were found between OpenPose-MA/Human-MA and 3D-MA for each knee valgus angle during landing, as indicated by high Pearson’s r correlation coefficients (Human-MA: 0.883-0.978, OpenPose-MA: 0.846-0.966) and no significant differences were found at landing phase or knee valgus excursion (Figure 4). Regarding the mean absolute errors (MAEs) of knee valgus angles, no significant difference was found between OpenPose-MA and Human-MA (Table 3). The MAE (95% CI) for OpenPose-MA throughout the landing phase was 2.4° (1.9° to 3.0°), while for Human-MA, it was 3.2° (2.1° to 4.4°). These results indicate that both OpenPose-MA and Human-MA exhibit comparable levels of accuracy in estimating knee valgus angles during the DVJ landing phase. The MAEs for both methods fell within the range of good accuracy, suggesting that they provide reliable estimations of knee valgus angles.
Pattern of kinematicsThe mean CMCs (SD) between Human-MA and 3D-MA, as well as OpenPose-MA and 3D-MA, were calculated separately for males and females (Table 4). No significant difference was found between OpenPose-MA and Human-MA in terms of CMCs. Qualitatively, the average waveform patterns obtained from both OpenPose-MA and Human-MA were consistent with 3D-MA (Figure 5). However, it is worth noting that the waveform patterns during landing differed between males and females, showing an inverted phase relationship. Figure 6 also demonstrates the average waveform patterns and their standard deviations for males and females in both OpenPose-MA and Human-MA.
The results of the study indicate that there were no significant differences in the knee valgus angles in the frontal plane during landing among OpenPose-MA, Human-MA, and 3D-MA. This suggests that OpenPose-MA can provide accurate measurements similar to those obtained through conventional Human-MA. The mean absolute errors (MAEs) of the knee valgus angles were not significantly different between OpenPose-MA and Human-MA, further supporting the comparable accuracy of the two methods. In terms of waveform pattern evaluation, OpenPose-MA exhibited a good correlation with 3D-MA. This indicates that OpenPose-MA can be a valuable tool for the qualitative assessment of movement patterns. Figure 7 visually demonstrates the usefulness and value of OpenPose-MA in evaluating movement patterns. Overall, the findings suggest that OpenPose-MA is a reliable and accurate measurement tool for assessing knee valgus angles in the frontal plane during landing. It provides comparable results to conventional Human-MA and offers the additional advantage of automated analysis and efficient data processing. The MAE of 2.4° (95% CI: 1.9° to 3.0°) observed in OpenPose-MA compared to the gold standard 3D-MA indicates an acceptable level of accuracy for clinical evaluation. Previous studies have suggested that absolute errors within this range are considered acceptable in clinical assessments (McGinley et al., 2009; Bessone et al., 2022). Moreover, it is worth noting that individuals with sports-related ACL injuries have been found to exhibit significantly greater dynamic maximum knee valgus angles during the DVJ task compared to those without ACL injuries, with a difference of approximately 7.6° (Hewett et al., 2005). Given this information, the accuracy provided by OpenPose-MA can be valuable in detecting subtle angle differences that may increase the risk of sports-related injuries, such as ACL injuries, as reported in previous studies (Hewett et al., 2005). Therefore, OpenPose-MA not only offers an acceptable level of accuracy but also has the potential to contribute to injury risk assessment and prevention by detecting differences in knee valgus angles associated with increased injury risk. The analysis of ICCs (1,3) and their 95% confidence intervals demonstrated that the DVJ were highly stable and reproducible (Table 1). Furthermore, the OpenPose-MA had sufficient reproducibility. Therefore, the DVJ using OpenPose-MA is considered practical and useful for sports-related functional assessment and screening. In this study, the MAEs for the knee valgus angles in the frontal plane during the DVJ landing phase was 2.4° (95% CI: 1.9° to 3.0°). In contrast, a previous study using smartphone cameras reported the MAEs of 5.82° (Viswakumar et al., 2021), and a report using digital video cameras synchronized with three-dimensional motion analysis showed 5.6° (Stenum et al., 2021). Therefore, the results of this study indicate a better estimation accuracy of OpenPose compared to previous studies. This improvement can be attributed to the matching of the video setup (measurement frequency, resolution, camera distance) with the reference three-dimensional motion analysis and the correction of lens distortion in the video footage. The results suggest that environmental factors may affect the results of OpenPose estimation. OpenPose-MA holds significant potential for revolutionizing motion analysis and offers numerous benefits to biomechanical research. The key advantages of OpenPose-MA are outlined as follows:
- Cost savings: OpenPose-MA eliminates the need for expensive motion analysis equipment and software, making it more accessible and cost-effective for researchers. This affordability is expected to promote the widespread adoption of motion analysis technology.
- Time efficiency: By automating time-consuming tasks, such as processing high-frequency video images and handling large datasets, OpenPose-MA significantly improves efficiency. Researchers can allocate their time to more complex and creative aspects of analysis, accelerating the research process.
- High reproducibility: OpenPose-MA minimizes potential errors and biases associated with manual execution. It ensures consistent accuracy and reproducibility, enhancing the reliability of motion analysis results.
- Measurement flexibility: Unlike traditional 3D motion analysis that requires controlled laboratory settings and the attachment of skin markers, OpenPose-MA only relies on regular video recordings. This flexibility enables analysis of movements during everyday activities and sports competitions, expanding the scope of biomechanical research.
Based on these aforementioned advantages, OpenPose-MA holds the potential to greatly enhance the field of motion analysis in both clinical and sports settings. In this study, OpenPose-MA effectively highlighted distinct waveform pattern differences between males and females during the landing phase of the DVJ task. Males exhibited a tendency towards varus knee joint motion, while females showed significantly valgus-oriented knee joint motion. These findings align with previous research on sex differences (Hewett et al., 2005; Noyes et al., 2005; Peebles et al., 2020; Kawaguchi et al., 2021), further validating the suitability of OpenPose-MA for qualitative evaluation of landing patterns. Notably, this study is the first to investigate and identify the inherent errors in both OpenPose-MA and Human-MA. Measures were taken to eliminate potential sources of error, including the correction of camera lens distortion and synchronization of video and motion analysis data. The knee valgus angle calculation and digital low-pass filtering were standardized across both methods. Additionally, a zero-reference point was established in the participants' standing position. As a result, this experiment provides a comprehensive assessment of the identification errors associated with OpenPose-MA and Human-MA by minimizing confounding factors. This study successfully demonstrated the applicability of OpenPose-MA to prompt movements (e.g., jumping) as well as slow movements (e.g., squatting and walking), consistent with previous research (Ota et al., 2020; Ota et al., 2021; Ino et al., 2023). Ota and colleagues reported high validity for knee flexion-extension angles during the bilateral squat (R2 = 0.83, ICC = 0.80) (Ota et al., 2020), while our study reported strong correlations for knee valgus angles during the DVJ (Pearson’s r, 0.846-0.966), indicating comparable accuracy. Additionally, our study revealed good validity for knee valgus angle waveforms (CMC = 0.86 ± 0.14) and MAEs during the DVJ, which were not addressed in previous studies (Cao et al., 2019; Ota et al., 2020; Ota et al., 2021). These findings provide valuable insights for the clinical application of OpenPose-MA. However, this study has several limitations. First, the reference 3D-MA method used in this study may have inherent errors due to skin movement (Everaert et al., 1999). Second, the 2D nature of the analysis may have been influenced by the subject's orientation relative to the camera, highlighting the importance of careful experimental setup as emphasized in previous research (Zago et al., 2020). Third, the resolution of video images could potentially impact the results (Zago et al., 2020), but we minimized this concern by utilizing high-definition videos. Fourth, the resilience of the OpenPose algorithms to lighting conditions and extraneous noise needs to be verified. Fifth, Human-MA has the potential for inter-rater variability and bias. In this study, one well-trained analyst conducted the study. Finally, OpenPose algorithms are susceptible to biases and uncertainties arising from the training data and algorithm design. Future directions could involve developing specialized AI algorithms for biomechanics research and exploring the potential of 3D motion analysis using AI to address these challenges.
The results demonstrated that OpenPose-MA achieved acceptable accuracy relative to 3D-MA and exhibited no significant difference compared to Human-MA in measuring knee valgus angle. Notably, OpenPose-MA offers several advantages over conventional Human-MA, including cost and time efficiencies.
ACKNOWLEDGEMENTS |
We acknowledge funding support from JSPS KAKENHI Grant Number JP20K19317. We also thank Prof. Masanori Yamanaka working at Hokkaido Chitose College of Rehabilitation for his valuable advice on the project. We are grateful to Mr. Itsuki Takahashi (RPT) working at Koga Hospital for his technical assistance. The measurement complied with the current laws of the country in which they were performed. The authors have no conflicts of interest to declare. The datasets generated and analyzed during the current study are not publicly available, but are available from the corresponding author who was an organizer of the study. |
|
AUTHOR BIOGRAPHY |
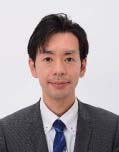 |
Takumi Ino |
Employment: Graduate School of Health Sciences, Hokkaido University, Sapporo, Japan. |
Degree: PhD |
Research interests: Rehabilitation and prevention for sports injuries, Biomechanics. |
E-mail: ino-t@hus.ac.jp |
|
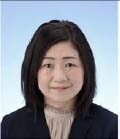 |
Mina Samukawa |
Employment: Faculty of Health Sciences, Hokkaido University, Sapporo, Japan |
Degree: PhD |
Research interests: Effects of therapeutic exercises and prevention for sports injuries. |
E-mail: mina@hs.hokudai.ac.jp |
|
 |
Tomoya Ishida |
Employment: Faculty of Health Sciences, Hokkaido University, Sapporo, Japan |
Degree: PhD |
Research interests: Rehabilitation and prevention for sports injuries. |
E-mail: t.ishida@hs.hokudai.ac.jp |
|
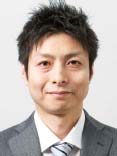 |
Naofumi Wada |
Employment: Department of Information and Computer Science, Faculty of Engineering, Hokkaido University of Science, Sapporo, Japan. |
Degree: PhD |
Research interests: Computer vision and image/video processing. |
E-mail: wada-n@hus.ac.jp |
|
 |
Yuta Koshino |
Employment: Faculty of Health Sciences, Hokkaido University, Sapporo, Japan |
Degree: PhD |
Research interests: Rehabilitation and prevention for sports injuries. |
E-mail: y-t-1-6@hs.hokudai.ac.jp |
|
 |
Satoshi Kasahara |
Employment: Faculty of Health Sciences, Hokkaido University, Sapporo, Japan |
Degree: PhD |
Research interests: Rehabilitation for neurological disease and motor control for aged adults |
E-mail: kasahara@hs.hokudai.ac.jp |
|
 |
Harukazu Tohyama |
Employment: Faculty of Health Sciences, Hokkaido University, Sapporo, Japan |
Degree: PhD |
Research interests: Rehabilitation for sports injuries and exercise therapy for musculoskeletal disorders. |
E-mail: tohyama@med.hokudai.ac.jp |
|
|
|
REFERENCES |
 Akbari H., Kuwano S., Shimokochi Y (2023) Effect of Heading a Soccer Ball as an External Focus During a Drop Vertical Jump Task. Orthopaedic Journal of Sports Medicine 11. Crossref
|
 Andriluka, M., Pishchulin, L., Gehler, P. and Schiele, B. (2014) 2D
Human Pose Estimation: New Benchmark and State of the Art
Analysis. In: 2014 IEEE Conference on Computer Vision and
Pattern Recognition 3686-3693. Crossref
|
 Baker R (2006) Gait analysis methods in rehabilitation. Journal of NeuroEngineering and Rehabilitation 3, 4. Crossref
|
 Bessone V., Hoschele N., Schwirtz A., Seiberl W (2022) Validation of a new inertial measurement unit system based on different dynamic movements for future in-field applications. Sports Biomechanics 21, 685-700. Crossref
|
 Bonnette S., DiCesare C.A., Kiefer A.W., Riley M.A., Foss K.D.B., Thomas S., Diekfuss J.A., Myer G.D (2020) A Technical Report on the Development of a Real-Time Visual Biofeedback System to Optimize Motor Learning and Movement Deficit Correction. Journal of Sports Science & Medicine 19, 84-94. Pubmed
|
 Cao Z., Hidalgo Martinez G., Simon T., Wei S.-E., Sheikh Y.A (2019) OpenPose: realtime multi-Person 2D pose estimation using part affinity fields. IEEE Transactions on Pattern Analysis and Machine Intelligence 43, 172-186. Crossref
|
 Cao, Z., Simon, T., Wei, S.E. and Sheikh, Y. (2017) Realtime multiperson 2d pose estimation using part affinity fields. Proceedings of the IEEE conference on computer vision and pattern
recognition, 7291-7299. Crossref
|
 Cappozzo A., Della Croce U., Leardini A., Chiari L (2005) Human movement analysis using stereophotogrammetry. Part 1: theoretical background. Gait & Posture 21, 186-196. Crossref
|
 Chester V.L., Biden E.N., Tingley M (2005) Gait analysis. Biomedical Instrumentation & Technology 39, 64-74.
|
 D’Antonio E., Taborri J., Mileti I., Rossi S., Patané F (2021) Validation of a 3D markerless system for gait analysis based on OpenPose and two RGB webcams. IEEE Sensors Journal 21, 17064-17075. Crossref
|
 Everaert D.G., Spaepen A.J., Wouters M.J., Stappaerts K.H., Oostendorp R.A (1999) Measuring small linear displacements with a three-dimensional video motion analysis system: determining its accuracy and precision. Archives of Physical Medicine and Rehabilitation 80, 1082-1089. Crossref
|
 Fan J., Gu F., Lv L., Zhang Z., Zhu C., Qi J., Wang H., Liu X., Yang J., Zhu Q (2022) Reliability of a human pose tracking algorithm for measuring upper limb joints: comparison with photography-based goniometry. BMC Musculoskeletal Disorders 23, 877. Crossref
|
 Faul F., Erdfelder E., Buchner A., Lang A.G (2009) Statistical power analyses using G*Power 3.1: tests for correlation and regression analyses. Behavior Research Methods 41, 1149-1160. Crossref
|
 Faul F., Erdfelder E., Lang A.G., Buchner A (2007) G*Power 3: a flexible statistical power analysis program for the social, behavioral, and biomedical sciences. Behavior Research Methods 39, 175-191. Crossref
|
 Ferrari A., Cutti A.G., Cappello A (2010a) A new formulation of the coefficient of multiple correlation to assess the similarity of waveforms measured synchronously by different motion analysis protocols. Gait & Posture 31, 540-542. Crossref
|
 Ferrari A., Cutti A.G., Garofalo P., Raggi M., Heijboer M., Cappello A., Davalli A (2010b) First in vivo assessment of "Outwalk": a novel protocol for clinical gait analysis based on inertial and magnetic sensors. Medical & Biological Engineering & Computing 48, 1-15. Crossref
|
 Fleiss, J.L. (1986) The design and analysis of clinical experiments. New
York: Wiley.
|
 Ford K.R., Myer G.D., Hewett T.E (2007) Reliability of landing 3D motion analysis: implications for longitudinal analyses. Medicine & Science in Sports & Exercise 39, 2021-2028. Crossref
|
 Harato K., Morishige Y., Kobayashi S., Niki Y., Nagura T (2022) Biomechanical features of drop vertical jump are different among various sporting activities. BMC Musculoskelet Disord 23, 331. Crossref
|
 Hensley C.P., Kontos D., Feldman C., Wafford Q.E., Wright A., Chang A.H (2022) Reliability and validity of 2-dimensional video analysis for a running task: a systematic review. Physical Therapy in Sport 58, 16-33. Crossref
|
 Hewett T.E., Ford K.R., Hoogenboom B.J., Myer G.D (2010) Understanding and preventing acl injuries: current biomechanical and epidemiologic considerations - update 2010. North American Journal of Sports Physical Therapy 5, 234-251.
|
 Hewett T.E., Myer G.D., Ford K.R., Heidt R.S., Colosimo A.J., McLean S.G., van den Bogert A.J., Paterno M.V., Succop P (2005) Biomechanical measures of neuromuscular control and valgus loading of the knee predict anterior cruciate ligament injury risk in female athletes: a prospective study. The American Journal of Sports Medicine 33, 492-501. Crossref
|
 Hidalgo, G., Raaj, Y., Idrees, H., Xiang, D., Joo, H., Simon, T. and
Sheikh, Y. (2019) Single-network whole-body pose estimation. Proceedings of the IEEE/CVF international conference on computer vision, 6982-6991.
|
 Ino T., Samukawa M., Ishida T., Wada N., Koshino Y., Kasahara S., Tohyama H (2023) Validity of AI-Based Gait Analysis for Simultaneous Measurement of Bilateral Lower Limb Kinematics Using a Single Video Camera. Sensors (Basel) 23, 9799. Crossref
|
 Ishida T., Ino T., Yamakawa Y., Wada N., Koshino Y., Samukawa M., Kasahara S., Tohyama H (2024) Estimation of Vertical Ground Reaction Force during Single-leg Landing Using Two-dimensional Video Images and Pose Estimation Artificial Intelligence. Physical Therapy Research 27, 35-41. Crossref
|
 Ishida T., Samukawa M., Suzuki M., Matsumoto H., Ito Y., Sakashita M., Aoki Y., Yamanaka M., Tohyama H (2023) Improvements in asymmetry in knee flexion motion during landing are associated with the postoperative period and quadriceps strength after anterior cruciate ligament reconstruction. Research in Sports Medicine 31, 285-295. Crossref
|
 Kawaguchi K., Taketomi S., Mizutani Y., Uchiyama E., Ikegami Y., Tanaka S., Haga N., Nakamura Y (2021) Sex-based differences in the drop vertical jump as revealed by video motion capture analysis using artificial intelligence. Orthopaedic Journal of Sports Medicine 9. Crossref
|
 Li, B., Williamson, J., Kelp, N., Dick, T. and Bo, A.P.L. (2021) Towards
balance assessment using Openpose. Annual International
Conference of the IEEE Engineering in Medicine and Biology
Society (EMBC), 7605-7608.
Crossref
|
 Lin, T.-Y., Maire, M., Belongie, S., Hays, J., Perona, P., Ramanan, D.,
Dollár, P. and Zitnick, C.L. (2014) Microsoft coco: Common
objects in context. In: Computer Vision–ECCV 2014: 13th
European Conference, Zurich, Switzerland, September 6-12,
2014, Proceedings, Part V 13, Springer, 740-755.
Crossref
|
 McGinley J.L., Baker R., Wolfe R., Morris M.E (2009) The reliability of three-dimensional kinematic gait measurements: a systematic review. Gait & Posture 29, 360-369. Crossref
|
 Noyes F.R., Barber-Westin S.D., Fleckenstein C., Walsh C., West J (2005) The drop-jump screening test: difference in lower limb control by gender and effect of neuromuscular training in female athletes. The American Journal of Sports Medicine 33, 197-207. Crossref
|
 Ota M., Tateuchi H., Hashiguchi T., Ichihashi N (2021) Verification of validity of gait analysis systems during treadmill walking and running using human pose tracking algorithm. Gait & Posture 85, 290-297. Crossref
|
 Ota M., Tateuchi H., Hashiguchi T., Kato T., Ogino Y., Yamagata M., Ichihashi N (2020) Verification of reliability and validity of motion analysis systems during bilateral squat using human pose tracking algorithm. Gait & Posture 80, 62-67. Crossref
|
 Peebles A.T., Dickerson L.C., Renner K.E., Queen R.M (2020) Sex-based differences in landing mechanics vary between the drop vertical jump and stop jump. Journal of Biomechanics 105, 109818. Crossref
|
 Piazza S.J., Cavanagh P.R (2000) Measurement of the screw-home motion of the knee is sensitive to errors in axis alignment. Journal of Biomechanics 33, 1029-1034. Crossref
|
 Plug-in Gait Reference Guide (2016) Nexus 2.15 Documentation, Vicon
Documentation. Available from URL: https://docs.vicon.com/display/Nexus215/Plug-in+Gait+Reference+Guide
[Accessed 28 June 2023].
|
 Rabin A., Einstein O., Kozol Z (2018) Agreement between visual assessment and 2-dimensional analysis during jump landing among healthy female athletes. Journal of Athletic Training 53, 386-394. Crossref
|
 Stenum J., Rossi C., Roemmich R.T (2021) Two-dimensional video-based analysis of human gait using pose estimation. PLOS Computational Biology 17, e1008935. Crossref
|
 Viswakumar A., Rajagopalan V., Ray T., Gottipati P., Parimi C (2021) Development of a Robust, Simple, and Affordable Human Gait Analysis System Using Bottom-Up Pose Estimation With a Smartphone Camera. Frontiers Physiology 12, 784865. Crossref
|
 Yamamoto M., Shimatani K., Hasegawa M., Kurita Y., Ishige Y., Takemura H (2021) Accuracy of temporo-spatial and lower limb joint kinematics parameters using OpenPose for various gait patterns with orthosis. IEEE Transactions on Neural Systems and Rehabilitation Engineering 29, 2666-2675. Crossref
|
 Zago M., Luzzago M., Marangoni T., De Cecco M., Tarabini M., Galli M (2020) 3D tracking of human motion using visual skeletonization and stereoscopic vision. Frontiers in Bioengineering and Biotechnology 8, 181. Crossref
|
|
|
|
|
|
|