|
|
|
ABSTRACT |
The purpose of the present study was to relate the training intensity distribution with performance in a Half-Ironman distance triathlon competition. A total of 18 recreational-level triathletes were divided into two training groups according to their training intensity distribution: Polarized (POL) and Pyramidal (PYR). Prior to the specific training period of the study, subjects performed a ramp-protocol test, running and cycling to determine ventilatory thresholds (VT) through gas-exchange analysis. For swimming, subjects performed an 800-metre test to establish their training zones. Training was quantified based on the cumulative time spent in 3 intensity zones: zone 1 (low intensity, <VT1), zone 2 (moderate intensity, VT1-VT2) and zone 3 (high intensity >VT2). POL competed 84.5%/4.2%/11.3% and PYR 77.9%/18.8%/3.3% of total training time for zones 1,2 and 3 respectively. The goal of the training period was a half Ironman distance triathlon. Training time in zone 2 inversely correlated with swimming and cycling race time in POL and with running and total race time in PYR. Power at VT2 on bike and speed at VT2 as well as maximum aerobic power and speed in the physiological post test inversely correlated with bike and run segment respectively and with total race time. These results suggest that training time in zone 2 was related with better performance on a Half-Ironman race in amateur triathletes. Future experimental research is needed to clarify the importance of training intensity distribution regarding performance. |
Key words:
Endurance training, triathlon, training zones, endurance performance
|
Key
Points
- POL and PYR training distributions showed a significant positive effect on the performance of the triathletes in the three segments.
- Training intensity in zone 2 was related with a better performance on a half-Ironman race in recreational triathletes.
- According to our results, coaches should not discard training time in zone 2 in recreational triathletes to train a Half-Ironman race.
|
An ultra-endurance event is defined as a continuous effort lasting more than four hours (Kreider, 1991). Thus, half-ironman triathlon can be included within this category especially for recreational participants. Success in these competitions is conditioned by several factors (physiological factors, hydration, nutrition, thermoregulation, climatic factors, distance, road profiles, etc.) (Laursen and Rhodes, 2001). Consequently, it is difficult to establish relations between the training and the triathletes’ performance in long endurance races, unless all the subjects have taken part in the same race or competition (Laursen and Rhodes, 2001; Muñoz et al., 2014b). The number of half-Ironman triathlon events (1.9-km swim, 90-km bike, 21.1-km run) and their participants has increased exponentially over the last decade (WTC, 2016). However, there is a lack of scientific evidence enabling to substantiate specific training recommendations to undertake these races, with training intensity distribution being one of the main variables that coaches must consider in their planning. This concept of training intensity distribution is defined as the time of exercise that the athlete spends at the different zones of training intensity (Stoggl and Sperlich, 2014). Usually, following the model of Skinner and McLellan (1980), training intensity distribution is found in three training zones: zone 1, under the first ventilatory threshold (<VT1); zone 2, between first and second ventilatory threshold (VT1-VT2); zone 3, above the second ventilatory threshold (>VT2). Intensity distribution known as polarized training is based on a high percentage of time or distance spent in both zone 1 and zone 3 and only a small portion of training in zone 2. An example of polarized training intensity distribution could be spend 80% in zone 1, 5% in zone 2 and 15% in zone 3, with percentages of zone 1 greater than zone 3 and zone 3 always greater than zone 2 (Schumacher and Mueller, 2002; Treff et al., 2019) (R3). This distribution has been described in many other endurance sports as rowing (Steinacker et al., 2000), running (Billat et al., 2001) or cross country skiing (Seiler and Kjerland, 2006). Several experimental studies have shown that polarized training maybe is a better model of training intensity distribution compared to other training intensity distribution models for endurance sports (Muñoz et al., 2014a; Stoggl and Sperlich, 2014). On the other hand, pyramidal training intensity distribution is characterised by accumulating a higher percentage of training time in zone 2 (15-20%) and less in zone 3, but as in the case of the polarized model, the highest percentage of training takes place in zone 1 (Lucia et al., 2000b; Treff et al., 2019). Prospective randomized-controlled trials have document similar improvements in this distribution compared to polarized (Ingham et al., 2008; Treff et al., 2017). Scientific evidence supports that a higher percentage of training time (from 70% to 90%) in zone 1 has a high impact on performance (Esteve-Lanao et al., 2007; Muñoz et al., 2014a; Tnønessen et al., 2014). An excess of high intensity training can lead the athlete to overtraining or a higher incidence of injuries (Maffetone and Laursen, 2016). Based on this previous research, it seems clear that high part of the training time should be in zone 1, but several questions arise such as, “how should the remaining of the training be distributed?” or “what is the best training intensity distribution model for recreational athletes?” There is no definite answer to these questions to date. Furthermore, studies are lacking on training intensity distribution, and a comparison of its effects in different specific competitions. Some studies have compared the training with a simulated races as a 10 km of running (Esteve-Lanao et al., 2007), a time trial 40 km of cycling (Neal et al., 2012) or an all-out 2000 m ergometer test (Treff et al., 2017). Muñoz et al. (2014b) are the only authors to have done so with a triathlon race (non-simulated): they analyzed training intensity distribution in amateur triathletes during a season and they found inverse correlations between training time and percentage time in zone 1 with regard to competition time in an Ironman race. Therefore, the aim of the present study is to relate training intensity distribution with performance during a Half-Ironman race in two groups that followed two different training intensity distributions: "polarized" 84.4% / 4.3% / 11.2% distribution and "pyramidal" 77.9% / 18.8% / 3.3% distribution of total training time for zones 1,2 and 3, respectively.
ParticipantsA total of 18 recreational-level male triathletes participated in the study. Their main goal for the training period was to prepare for a Half-Ironman distance event in Alicante, Spain. They all trained with the same coach (RC) within a supervised training program following the same periodization model but not the same training intensity distribution. The inclusion criteria were to have less than two years of previous training in triathlon and to have completed less than 95% of total training load. Furthermore, all subjects had to go through a transition period (3-week) before the training period started. These triathletes were divided into two groups: polarized (POL), and pyramidal (PYR) according to the training intensity distribution that subjects had followed. All participants provided written informed consent to take part in the study, which was approved by the Alicante University Ethics Committee (UA-2017-04-11 expedient). Data of the final sample is shown in Table 1. Figure 1 illustrates the design of the study in order to understand the procedures of the study.
Physiological testing and training zone settingsIncremental tests of volitional exhaustion were used to determine training zones in cycling and running. A ramp protocol was used for cycling on a roller (elite® Crono fluid elasto gel, Italy) starting at 50 W and increasing 5 W each 12 seconds (Muñoz et al., 2014b). Participants used their own bike for the test with a power wheel (powertap®). The running test was performed on a 400-metre homologated track. Participants started at 10 km/h and increased 0,3 km/h every 200 meters (Brue, 1985). Both tests were conducted using a portable gas-exchange analyzer (Cosmed® K4b 2, Italy). During the test, the following variables were measured: oxygen uptake (VO2); pulmonary ventilation (VE); ventilatory equivalent for oxygen (VE/VO2); ventilatory equivalent for carbon dioxide (VE/VCO2); and end-tidal partial pressure of oxygen (PETO2) and carbon dioxide (PETCO2). Maximal oxygen uptake (VO2max) was recorded as the highest VO2 value obtained for any continuous 1-minute period. VT1 was determined using the criteria of an increase in both VE/VO2 and PETO2 with no increase in VE/VCO2, whereas VT2 was determined using the criteria of an increase in both VE/VO2 and VE/VCO2 and a decrease in PETCO2. Two independent observers identified VT1 and VT2. In case of disagreement, the opinion of a third observer was obtained (Doherty et al., 2003). Heart rate (HR) was continuously monitored during the test using radio telemetry (Polar Electro®, Finland). Later, a range of HR and power or velocity for each training zone was established. Swimming training zones were calculated based on an 800-metre test in a 25-meter pool. Participants had to cover the distance in the shortest time possible. A difference greater than 5% in the part-time of each 100-metre was not allowed. To determine the training zones, the medium pace of the 100-metre was considered to be the pace associated with VT2 and a total of 120% of that medium pace was considered to be the pace associated with VT1 (Sweetenham and Atkinson, 2003). Both pre-test and post-test were performed during a week, with at least 48 hours of rest between tests. Three main training zones were defined for this study: zone 1 (at or below VT1), zone 2 (between VT1 and VT2) and zone 3 (at or beyond VT2) (Skinner and McLellan, 1980). These zones were subdivided into additional training zones for daily workouts. Participants thus trained with eight training zones (Cejuela and Esteve-Lanao, 2011) in order to improve accuracy in some workouts and to use the ECO-model to control the training load.
Main characteristics of the training periodAfter 3 weeks in the transition period, triathletes completed a 20-week training program broken down into a 7-week general training program and a 13-week specific training program with different training intensity distributions: a polarized training program or a pyramidal training program. A specific method to quantify the training load in triathlon, ECOs (Cejuela and Esteve-Lanao, 2011), was used in this study. Participants could not perform systematic training during the transition period. Tests took place during the last week of the general training period (week 7) and during week 12 of the specific training period. The results of the post-test were used to establish correlations with Half-Ironman performance. Both pre-tests and post-tests were performed during a week, with at least 48-hours of rest between tests. The general training program was the same for all triathletes during this time. Training intensity distribution was 88%, 10% and 2%, in zones 1, 2 and 3, respectively. Total training load distribution in each segment was 26% in swimming, 31% in bike and 43% in run. The main goal of this training period was to build general physical fitness. For that, the training load and total volume was progressively increased. Regarding the specific training program, POL and PYR followed the same training periodization, which was divided into three mesocycles. The first and second mesocycle consisted of 4 weeks and the third mesocycle consisted of 5 weeks of training. Training volume peaks were prescribed at the end of the first and second mesocycles (4th and 8th week). Training load peaks were prescribed at week 4 for swimming and cycling and at week 8 for running. Specific transition sessions were included in almost every week in this training period, generally bike-run transitions. In the first week in each mesocycle, participants completed a week with a lower training load for recovery purposes (week 1, 5 and 9). The third mesocycle included a tapering period of 3 weeks and a post-test (week 12). Hydration and nutritional guidelines were followed during the training period based on a personal interview with the same sports nutritionist. The training volume prescribed was the same for both groups (≈155 training hours) but training intensity distribution was different between groups during specific training time. POL completed 84.5 ± 1.3 /4.2 ± 1.5 /11.3 ± 0.5 in zones 1.2 and 3 respectively, and PYR 77.9 ± 0.5 in zone 1, 18.8 ± 0.7 in zone 2 and 3.3 ± 0.5 in zone 3 The PYR training plan consisted of 106 training sessions (28 for swimming, 34 for cycling and 44 for running). POL included one more training session for running in order to equate the training volume in both groups. The distribution of the training load in each segment was 28% for swimming, 38% for cycling and 34% for running. The following figures (Figure 1, Figure 2, Figure 3 and Figure 4) show a summary of the training load and the training intensity distribution by segment during the specific training period. Polarization index was calculated in order to quantify the individual level of Polarization in each triathlete (Treff et al., 2019). Strength training program: A total of 36 workouts (14 in the general training period and 22 in the specific training period) included a strength-training program, which was the same for both groups. It consisted of progressive workouts with resistance-training machines. To select the strength exercises, the recommendations of Klion and Jacobson (2014) were followed, combining exercises involving both upper and lower body muscles. In addition, core stability exercises were carried out in each workout. These sessions were commonly conducted twice a week, usually under the supervision of one of the coaches. Loads gradually increased from 40% of RM (RPE 3) and a moderate speed, to 70-75% (RPE 7) of RM and a high speed in concentric phases. Resistance training was gradually combined with specific strength methods in each sport (paddles for swimming, hills or harder gears for cycling and hills were also used for running).
Quantification of exercise load in trainingTriathletes were filling personal training logs with the information recorded in their HR monitors regarding the amount of time spent in each training zone. In order to control the intensity in each workout, the following strategies were used: on the one hand, during the general training period, RPE was used for training control, using a scale of perceived effort from 0 to 10 (Borg, 1982). On the other hand, HR and RPE were used for low intensity workouts in running and cycling during the specific training period. Speed and power were also used to control moderate and high intensity workouts for running and cycling in this period. RPE and the medium pace for 100-metre were used to control swimming workouts. Besides, swimming sessions and intensities of workouts for the run segments were always supervised by one of the coaches (SS or RC).
Statistical analysisA descriptive analysis was carried out using the means and standard deviation of the triathlete’s variables, of the volume and load variables, and of the training and performance variables. To detect any statistically significant differences between these two training groups’ variables, the nonparametric test of the Mann-Whitney U test was performed. After testing for normal distribution using a Shapiro–Wilkinson-Test, the nonparametric contrast of the Mann-Whitney U was calculated to detect statistically significant differences between the groups. A no parametric Wilcoxon test to calculate differences between pre and post test. Cohen’s d (dCohen) was calculated to estimate effect sizes (Cohen, 1988) of pre and post test. In addition, using Cohen's d, the results of the two training groups have also been compared. Cohen’s d is defined as follows: trivial: 0–|0.2|, small: |0.2|–|0.6|, moderate |0.6|–|1.2|, large: |1.2|–|2.0|, very large: |2.0|–|4.0|, and infinite: |4.0| (Hopkins, 2003). The Spearman correlation coefficient was used to study the correlation between the times for the three segments in the competition and for the total Half-Ironman time with the performance variables, the training times in the different total zones and according to the training group. The level of statistical significance was 0.05. The statistical package SPSS 24.0 and the Microsoft Excel spread sheet were used in the data analysis.
Five participants did not carry out the inclusion criteria. Thus, only 13 triathletes were included in the statistical analysis. Table 1 shows the results for the final sample. Table 2 presents a summary of the training parameters of the specific period. No differences in training volume and training load were found between groups. However, there were significant differences in each group’s training intensity distribution. Table 3 shows the training intensity distribution and polarized index in each athlete during the specific training period. The effect of the training is presented in Table 4 with the comparison with pre and post test. Both groups increase their performance significantly in every segment in almost all analyzed variables. PYR increased the speed associated at VT2 and MAS significantly but in POL this improvement was not statistically significant. Effect size was categorized as small or moderate in most variables in both PYR and POL. Only, it is observed a large effect size in power associated at VT1 in PYR. Correlations between performance in the test and Half-Ironman performance event are presented in Table 5. The swimming test time correlated with the swimming time in the race and with the total of the Half-Ironman time. Significant inverse correlations were found between power at VT2 and maximum aerobic power on bike with cycling segment performance and with total race time. Significant inverse correlations were also found between the pace at VT2 and maximum aerobic speed with the race running time and with the Half-ironman race performance. However, significant correlations were not found between V02Max in the cycling or running test with performance during the event. Table 6 shows the correlations between training time and the competition. There were no significant correlations between any of the subjects’ training time and the competition. However, training time <VT1 in POL correlated with more time in the swimming segment and training time between thresholds correlated with a faster time in the swimming and cycling segments. On the other hand, training time between thresholds in PYR inversely correlated with the time of the run segment and with the total race time. Conversely, training time between thresholds correlated with the running and total race time.
The percentage of training time spent in zones 1, 2 and 3 was 84,5%, 4,2% and 11,3%, respectively for POL and 77,9%, 18,8% and 3,3%, respectively for PYR. Previous studies have shown that endurance athletes should spend ≅80% of total training time in zone 1 (Lucía et al., 2000b; Billat et al., 2001; Seiler and Kjerland, 2006; Plews and Laursen, 2017). This emphasis in zone 1 rather than in zones 2 and 3 is associated with better performance in different endurance sport such as rowing (Ingham et al., 2008), running (Muñoz et al., 2014a) or triathlon (Muñoz et al., 2014b). Thus, both POL and PYR accumulated this high percentage of low intensity training time (zone 1). Weekly average training load was 785 ECOs for POL and 750 ECOs for PYR. These training load data differ from other reported values for preparing other endurance races. For example, 526 ECOs of weekly training load were reported during a specific period for a marathon and 834 ECOs were reported for an IM in recreational endurance athletes (Esteve-Lanao et al., 2017). The same applies to weekly average training time (≅12 hours), which is higher than marathon training time (≅5 hours) and slightly lower than in the case of Ironman (≅13 hours) (Esteve-Lanao et al., 2017). Despite the competition time for Half-Ironman being more similar to that of a marathon than to an Ironman’s (in athletes with the same performance levels), the need to train three segments instead of just one means that the weekly average training load and the weekly average training time brings Half-Ironman closer to Ironman training values than to marathon ones. However, if we analyse the concept of “ECOs per hour” (training load per training hour), it is significantly lower in Half-Ironman and in Ironman (≅65 ECOs per hour) than in Marathon training (≅100 ECOs per hour) (Esteve-Lanao et al., 2017). Naturally, this comparison of training data is between groups of recreational athletes. The reported average training load in elite triathletes exceeds 1000 ECOs (Saugy et al., 2016) and 20 hours of training per week (Mujika et al., 2017). Regarding the race performance, the participants of this study needed ≅30% more time to finish the race than the Half-Ironman competition time reported for elite triathletes (≅4 hours) (Knechtle et al., 2012), thus the sample was categorized as “recreational triathletes”. No significant differences were observed between the POL and PYR competition times. In fact, only two seconds differentiated both groups in the race that lasted over five hours. In this sense, other factors as total training volume (Muñoz et al; 2014b), previous experience (Knechtle et al.,2012) or even body composition (Knechtle et al., 2014) are more determinants than training intensity distribution in the final result of a long-distance triathlon race. Both training distributions showed a significant positive effect on the performance of the triathletes in the three segments. The only difference between groups was in running. PYR group showed a statistically significant improvement in the speed associated at VT2 and MAS in running and in POL this improvement was not statistically significant. These results are in line with Treff et al (2017), who did not find significant differences between polarized and pyramidal training in elite rowers. However, these authors also did not find significant differences between pre and post test in any performance measure with a similar period of intervention than our study. Perhaps, the difference of level in the sample is the key in the fact that we have found significant differences in the pre and post tests in almost all the variables and these differences have not been significant in the Treff et al (2017) study. On the other hand, our results differ to several experimental studies that suggested a greater improvements in endurance sports induced by a Polarized model instead of other training intensity distribution models (Esteve-Lanao et al., 2007; Muñoz et al., 2014a; Neal et al.,2011; Stoggl and Sperlich, 2014). It is difficult to compare our results with these studies, because the sample, time of intervention and training intensity distribution strategies were different in each investigation. Thus, the results of each study should be analyzed separately in base to the training intensity distribution used in each of one. The performance in the 800-metre swim test significantly correlated with the swim time in the race, although the distance in the competition was more than double and the triathletes swam in open water and were allowed drafting. Therefore, the 800-metre swim test could be used as a benchmark test to predict the performance of recreational athletes in the Half-Ironman swim segment. Curiously, performance in this test was also significantly correlated with the total final time in the race. Despite the fact that the swimming percentage of total race time is considerably lower than that of the other segments (≅12% swimming, ≅53% cycling, ≅35% running), it could be interesting to research in future studies how the energy cost during the swim segment affects the other two segments in recreational triathletes, as a poor technique may fatigue the triathlete excessively in the first segment and this would condition the athlete’s total performance in the test (Ferreira et al., 2016). Maximal aerobic power (MAP) in cycling and maximal aerobic speed (MAS) in running, as well as power and speed associated with VT2 correlated inversely with the time in the respective segments and with total competition time. Muñoz et al. (2014b) found similar correlations between power and power at VT2 in cycling and speed at VT2 and at VT1 in running but not with MAP and MAS. These differences can be explained by the duration of the effort: in the study of Muñoz et al. (2014b), the correlations were conducted with an Ironman race time and participants covered twice the distance (3.8 km swim, 180 km bike and 42 km run) of our triathletes. Despite this fact, it is difficult to maintain over ≅60 minutes of continuous effort at VT2 (Beneke, 2003), and it would be important to focus the training on increasing the power or velocity associated with this intensity in long endurance events. The relationship between VT2 and long distance triathlon performance could be explained by a higher fat oxidation rate deriving from the improvement of this parameter (Croci et al., 2014). No statistically significant correlations were found between training intensity distribution and race performance when analysing triathletes in the same group. These results are in line with Neal et al. (2011) who suggested that the effects of training intensity distribution were small in the adaptations and that other values can be more decisive for the performance of a group of Ironman distance triathletes. Previous research has shown that it is positive both for elite and sub-elite endurance athletes to adopt a polarized training model, with a low emphasis on moderate intensity (VT1-VT2) (Billat et al., 2001; Seiler and Kjerland, 2006; Stoggl and Sperlich, 2014). However, based on our results, POL triathletes who spent more training time in zone 2 showed the best performance in the swimming and cycling segments. In the same way, PYR triathletes who spent more VT1-VT2 training time obtained the best performance in the running segment as well as in the Half-Ironman. Surprisingly, PYR triathletes who spent more time >VT2 presented worse times in the running segment and in the Half-Ironman. In this respect, although most of the training volume was carried out at low intensity, moderate intensity is relevant and should not be discarded when prescribing training for popular long-distance triathlons, all the more so given that part of the competition is carried out at this intensity (Laursen and Rhodes, 2001). High intensity training could be necessary to obtain improvements on performance in highly trained athletes (Laursen and Jenkins, 2002). However, moderate intensity could conduct a similar effect, or even higher, in athletes with less training experience. In this respect, it is important to remark that the level of adaptations is clearly conditioned by the starting level of the athletes (Sellés-Pérez et al., 2019).
To the best of the authors' knowledge, this is the first study to have manipulated training intensity distribution in recreational Half-Ironman triathletes and to have performed correlations with performance in a competition. Despite the importance of a high percentage of training in zone 1, according to our results, coaches should not rule out the training prescription in zone 2, since the training time in this zone positively correlated with the performance in the Half-Ironman competition. However, some limitations, as a short time of specific training period or a reduce sample, should be considered to analyze the results. Also, the performance in a long-endurance race is conditioned by different aspects (weather conditions, nutrition, hydration…) that we could not take into account in the data analysis. Future research is needed on different training intensity distributions in groups with different levels of performance and their impact on diverse sports events.
ACKNOWLEDGEMENTS |
The experiments comply with the current laws of the country in which they were performed. The authors have no conflict of interest to declare. |
|
AUTHOR BIOGRAPHY |
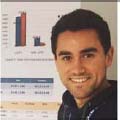 |
Sergio Selles-Perez |
Employment: Assistant professor in Alicante university. |
Degree: PhD |
Research interests: Recreational athletes in endurance sports. |
E-mail: Sergio.selles@ua.es |
|
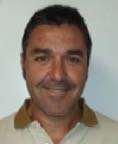 |
José Fernández-Sáez |
Employment: Assistant professor in the area of public health at the University of Alicante. |
Degree: PhD |
Research interests: Nutrition, optics, environmental epidemiology and politics and especially in public health and gender. |
E-mail: j.fernandez@ua.es |
|
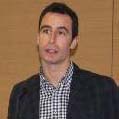 |
Roberto Cejuela |
Employment: Associate professor in Alicante University. |
Degree: PhD |
Research interests: The training load in both high performance athletes and amateurs, preferably in triathletes. |
E-mail: Roberto.cejuela@ua.es |
|
|
|
REFERENCES |
 Beneke R (2003) Methodological aspects of maximal lactate steady state implications for performance testing. European Journal of Applied Physiology 89, 95-99.
|
 Billat V. L, Demarle A, Slawinski J, Paiva M., Koralsztein J. P. (2001) Physical and training characteristics of top-class marathon runners. Medicine and Science in Sports and Exercise 33, 2089-2097.
|
 Borg G (1982) Psychophysical bases of perceived exertion’. Medicine and Science in Sports and Exercise 14, 377-381.
|
 Brue F (1985) A variant of the progressive and maximum runway test of Leger and Boucher for the precise and easy of the maximum aerobic speed. French Athletics Federation, 25-30 (In French).
|
 Cejuela R., Esteve-Lanao J. (2011) Training load quantification in triathlon. Journal of Human Sport and Exercise 6, 218-232.
|
 Cohen, J. (1988) Statistical power analysis for the behavioural sciences.
Hillside. NJ: Lawrence Earlbaum Associates.
|
 Croci I., Ingrid I. J, Wood R. E, Borrani F, Macdonald G. A., Byrne N. M. (2014) Fat oxidation over a range of exercise intensities: fitness versus fatness. Applied Physiology, Nutrition, and Metabolism 39, 1352-1359.
|
 Doherty M., Nobbs L., Noakes T. D. (2003) Low frequency of the “plateau phenomenon” during maximal exercise in elite British athletes. European Journal of Applied Physiology 89, 619-23.
|
 Esteve-Lanao J., Foster C., Seiler S., Lucia A. (2007) Impact of training intensity distribution on performance in endurance athletes. Journal of Strength and Conditioning Research 21, 943-949.
|
 Esteve-Lanao J., Moreno-Pérez D., Cardona C. A., Larumbe-Zabala E., Muñoz I., Selles S., Cejuela R. (2017) Is Marathon Training Harder than the Ironman Training? An ECO-method Comparison. Frontiers in Physiology. Frontiers 8, 298.
|
 Ferreira M. I, Barbosa T. M, Costa M. J, Neiva H. P., Marinho D. A. (2016) Energetics, biomechanics, and performance in masters’ swimmers: A systematic review. Journal of Strength and Conditioning Research 30, 2069-2081.
|
 Hopkins, W. G. (2003) A Scale of Magnitudes for Effect Statistics. A New
View of Statistics.
|
 Ingham S. A., Carter H., Whyte G. P., Doust H. (2008) Physiological and performance effects of low- versus mixed-intensity rowing training.’. Medicine and Science in Sports and Exercise 40, -579.
|
 Klion, M. and Jacobson, T. (2014) Triathlon anatomy. Champaign, Il:
Human Kinetics.
|
 Knechtle B (2014) Relationship of Anthropometric and Training Characteristics with Race Performance in Endurance and Ultra-Endurance Athletes. Asian Journal of Sports Medicine 5, 73.
|
 Knechtle B, Rüst C. A, Rosemann T., Lepers R. (2012) Age and gender differences in half-Ironman triathlon performances - the Ironman 70.3 Switzerland from 2007 to 2010. Open Access Journal of Sports Medicine 3, -59.
|
 Kreider R. B. (1991) Physiological considerations of ultraendurance performance. International Journal of Sport Nutrition 1, 3-27.
|
 Laursen P. B., Jenkins D. G. (2002) The scientific basis for high-intensity interval training. Sports Medicine 32, 53-73.
|
 Laursen P. B., Rhodes E. C. (2001) Factors affecting performance in an ultraendurance triathlon. Sports Medicine 31, 195-209.
|
 Lucía A, Hoyos J, Pérez M., Chicharro J L. (2000a) Metabolic and Neuromuscular Adaptations to Endurance Training in Professional Cyclists: A Longitudinal Study’. The Japanesse Journal of Physiology 50, 381-388.
|
 Lucía A, Hoyos J, Pérez M., Chicharro J L. (2000b) Heart rate and performance parameters in elite cyclists: a longitudinal study. Medicine and Science in Sports and Exercise 32, 1777-1782.
|
 Maffetone, P. B. and Laursen, P. B. (2016) 'Athletes: Fit but Unhealthy?'
Sports Medicine - Open. Springer, 2(1), 24
|
 Mujika I, da silveira F. P., Nosaka K. (2017) Blood markers of recovery from Ironman distance races in an elite triathlete. Journal of Sports Medicine and Physical Fitness 57, 1057-1061.
|
 Muñoz I, Cejuela R, Seiler S, Larumbe E., Esteve-Lanao J. (2014b) Training-intensity distribution during an ironman season: Relationship with competition performance. International Journal of Sports Physiology and Performance 9, 332-339.
|
 Muñoz I., Seiler S., Bautista J., España J., Esteve-Lanao J. (2014a) Does Polarized Training Improve Performance in Recreational Runners ?. International Journal Sport Physiology and Performance , 265-272.
|
 Neal C. M., Hunter A. M., Brennan L., O'Sullivan A., Hamilton D. L., DeVito G., Galloway S. D. R. (2012) Six weeks of a polarized training-intensity distribution leads to greater physiological and performance adaptations than a threshold model in trained cyclists. Journal of Applied Physiology 114, 461-471.
|
 Neal C. M., Hunter A. M., Galloway S. D. R. (2011) A 6-month analysis of training-intensity distribution and physiological adaptation in Ironman triathletes. Journal of Sports Sciences 29, 1515-1523.
|
 Plews D. J., Laursen P. B. (2017) Training Intensity Distribution Over a Four-Year Cycle in Olympic Champion Rowers: Different Roads Lead to Rio’. International Journal of Sports Physiology and Performance , 1-24.
|
 Saugy J. J., Schmitt L., Hauser A., Constantin G., Cejuela R., Faiss R., Wehrlin J. P., Rosset J., Robinson N., Millet G. P. (2016) Same performance changes after live high-train low in normobaric vs. hypobaric hypoxia. Frontiers in Physiology 7, 1-10.
|
 Schumacher Y. O., Mueller P. (2002) The 4000-m team pursuit cycling world record: theoretical and practical aspects. Medicine and Science in Sports and Exercise 34, 1029-36.
|
 Seiler K. S., Kjerland G. Ø. (2006) Quantifying training intensity distribution in elite endurance athletes: Is there evidence for an “optimal” distribution?. Scandinavian Journal of Medicine and Science in Sports 16, 49-56.
|
 Sellés-Pérez S., Fernández-Sáez J, Férriz-Valero A, Esteve-Lanao J., Cejuela R. (2019) Changes in triathletes’ performance and body composition during a specific training period for a Half-Ironman race. Journal of Human Kinetic 67, 185-198.
|
 Skinner J., McLellan T. (1980) The transition from aerobic to anaerobic metabolism. Research Quarterly for Exercise and Sport 51, 234-248.
|
 Stoggl T., Sperlich B. (2014) ‘Polarized training has greater impact on key endurance variables than threshold, high intensity, or high volume training’. Frontiers in Physiology.
|
 Sweetenham, B. and Atkinson, J. (2003) Championship swim training.
Champaing, IL. Human Kinetics.
|
 Tnønessen E., Sylta Ø., Haugen T. A., Erlend H., Suendsen I., Seiler S. (2014) The road to gold: Training and peaking characteristics in the year prior to a gold medal endurance performance. Plos One 9, 12-17.
|
 Treff G, Winkert K, Sareban M, Steinacker J. M, Becker M., Sperlich B. (2017) Eleven-week preparation involving polarized intensity distribution is not superior to pyramidal distribution in national elite rowers. Frontiers in Physiology 8, 515.
|
 Treff G., Winkert K, Sareban M, Steinacker J. M., Sperlich Billy (2019) The Polarization-Index: A Simple Calculation to Distinguish Polarized from Non-Polarized Training Intensity Distributions. Frontiers in Physiology 10, 707.
|
 WTC .(2016) World triathlon corporation, Tampa, FL.
|
|
|
|
|
|
|