|
|
|
ABSTRACT |
The aim of this study was to compare the assessment of swimming speed processed as a discrete variable and as a continuous variable in young swimmers. One-hundred and twenty young swimmers (60 boys: age = 12.91 ± 0.86 years; 60 girls: age = 12.46 ± 0.94 years) were analysed. The dataset for each sex was divided into three tiers: (i) tier #1 - best-performing swimmers; (ii) tier #2: intermediate-performing swimmers, and; (iii) tier #3 - poorest-performing swimmers. As a discrete variable, swimming speed showed significant sex and tier effects, and a significant sex*tier interaction (p < 0.001). Speed fluctuation showed a non-significant sex effect (p > 0.05), a significant tier effect (p < 0.001), and a non-significant sex*tier interaction (p > 0.05). As a continuous variable, the swimming speed time-curve presented significant sex and tier effects (p < 0.001) throughout the stroke cycle, and a significant sex*tier interaction (p < 0.05) in some moments of the stroke cycle. Swimming speed fluctuation analysed as a discrete variable and as a continuous variable can be used in a complementary way. Nonetheless, SPM can provide deeper insight into differences within the stroke cycle. Thus, coaches and practitioners should be aware that different knowledge about the swimmers’ stroke cycle can be learned by assessing swimming speed using both methods. |
Key words:
Assessment, biomechanics, modelling, performance, youth
|
Key
Points
- dv analysis provides insightful details about the swimmer’s net balance between thrust and drag. Less speed fluctuation is related to better performances.
- dv and SPM of swim speed can be used complementary. The former gives an overall bird view of the stroke-cycle, of the motor behavior. The latter drills down allowing to pinpoint in which key-moment of the cycle differences can be observed.
- Through dv, coaches and practitioners can quickly get an overall profile of the stroke-cycle. Conversely, choosing a continuous analysis (SPM) helps to pinpoint what are the strongest and weakest key-moments of the stroke-cycle.
- The selection of both dv and SPM of swim speed concurrently enables coaches and support staff to customize training programs and drills to improve technique.
|
As in other time-based sports, the goal in competitive swimming is to complete a given distance in the shortest possible time. Front-crawl, as the most popular swimming stroke, receives a significant amount of attention by researchers, coaches, and support staff (Kwok et al., 2021; Morais et al., 2018; Nikolaidis and Knechtle, 2017). Swimming speed is characterised by a periodically accelerated motion (Barbosa et al., 2010). Intra-cyclic variations of horizontal speed are commonly known as ‘speed fluctuation’ (dv). Thus, dv quantifies the variation in instantaneous speed around its mean value over a full stroke cycle. Dv can be quantified as a discrete variable of the speed curve by calculating the coefficient of variation (CV) (Barbosa et al., 2005):
in which CV is the coefficient of variation (in %), SD is the one standard deviation of a given time-series, and mean is the average of a given time-series. Due to the high complexity of data handling and analysis of time-series data, practitioners often refer to dv as a simple discrete value to assess the mechanical efficiency and performance of the swimmer (Barbosa et al., 2019; Seifert et al., 2010). Moreover, it is also used to get an overview of the magnitude of change observed by swimming speed (Barbosa et al., 2016). Previously, in swimming by age group, it was shown that faster swimmers in an event were more likely to have a lower dv than their slower counterparts (Barbosa et al., 2019; Figueiredo et al., 2016). Furthermore, Matsuda and co-workers (2014) observed that elite swimmers had significantly lower dv than beginners at various swimming speeds. The best-performing pubescent swimmers of both sexes also had a lower dv than the worst-performing swimmers (Silva et al., 2019a). However, the use of the CV to give an understanding about the dv can be misleading if the standard deviation is not directly proportional to the mean (Reed et al., 2002). Therefore, the CV can be influenced by the mean when decreases and increases of the mean are much greater than those observed by the standard deviation (Gonjo et al., 2023). Discrete data decreases the amount of potential information available, which may omit or overlook insightful details that could explain performance constraints (Richter et al., 2014). The CV of the dv falls in this rational. Based on the previously mentioned assumptions, it can be argued that the sensitivity to detect differences in swimming speed over an entire stroke cycle decreases when discrete categorisation and data compressing occur (as when using the dv). Discrete variables are unable to provide detailed information about behaviour across the speed-time series during a complete stroke cycle, as they only provide an average value of the entire stroke cycle. It does not allow verifying hypothetical differences at a given moment in the stroke cycle. On the other hand, analysing continuous time-series data has significant advantages by providing more sensitive details (Preatoni et al., 2013; Tassignon et al., 2018). 1D Statistical Parametric Mapping (SPM) is a statistical procedure that allows a continuous time-series analysis. It uses random field theory to perform topological inference by directly mapping the conventional Gaussian distribution to smooth n-dimensional data (Pataky, 2010). More specifically, SPM is an n-dimensional methodology for the topological analysis of smooth continuous changes associated with experimental interventions (Pataky, 2012). SPM can lessen the limitations indicated by discrete values, providing a framework for continuous statistical analysis of smooth bounded n-dimensional fields (Pataky, 2010). It has been stated that the SPM approach has two main advantages: (1) statistical results are presented directly in the original sampling space, so their space-time biomechanical context is immediately apparent, and; (2) there is no need to make assumptions regarding the spatial-temporal focus of the analysed signals (Pataky, 2010; 2012). Hence, SPM can be employed to maintain the integrity and sensitivity of time-series data. SPM is increasingly being used in sports science, contributing to a more detailed analysis of movement in specific performance settings (Bertozzi et al., 2022; Warmenhoven et al., 2018). In the case of the swimming speed time-curve, it will allow a better explanation of which moments of the stroke cycle differences may occur. In the context of swimming, continuous analysis was used to compare the arm-stroke kinematics between maximal and sub-maximal breaststroke swimming (Gourgoulis and Nikodelis, 2022), understand the underwater undulatory swimming kinematics (Ruiz-Navarro et al., 2021), and investigate inter-individual variability in muscle activation patterns during front crawl swimming (Martens et al., 2016). Thus, using continuous procedures to compare and analyse the swimming speed between the best and worst-performing swimmers during a complete stroke cycle can provide researchers and coaches with more detailed information about the differences within the stroke cycle. Therefore, this study aimed to compare swimming speed during a complete stroke cycle in young swimmers of both sexes as a discrete variable and as a continuous variable (SPM). For this, comparisons were made between performance levels (three tiers) according to sex. It was hypothesised that swimmers included in the fastest tier (both sexes) would present a significantly faster swimming speed and the dv would be significantly lower. Furthermore, analysis through SPM would allow the identification of specific key moments within the stroke cycle in which differences may occur.
ParticipantsOne hundred and twenty young swimmers participated (60 boys: age = 12.91 ± 0.86 years, height = 162.00 ± 11.07 cm, body mass = 51.23 ± 9.01 kg, arm span = 167.45 ± 11.07 cm, FINA points = 283.49 ± 85.18 points at the 100 m freestyle event in short course meter; 60 girls: age = 12.46 ± 0.94 years; height = 158.42 ± 5.87 cm, body mass = 49.51 ± 7.22 kg, arm span = 161.09 ± 6.10 cm, FINA points = 315.05 ± 85.72 points at the 100 m freestyle event in short course meter) who, at the time of data collection, were participating in regional and national levels competitions (tier 2 swimmers, McKay et al., 2022). Swimmers had more than three years of competitive background and included age-group national record holders, age-group national champions, and swimmers enrolled in a national talent-identification scheme. Swimmers were taking part in five to six training sessions per week (90 minutes each session). Parents or guardians, as well as swimmers, provided informed consent. All procedures were in accordance with the Declaration of Helsinki regarding human research, and the University Ethics Board approved the research design (N.º 72/2022). The dataset for each sex was divided into three tiers (each one with 20 swimmers) based on the fastest swimming speed performed during an all-out trial of 25 m front-crawl: (i) tier #1 - swimmers with the best performances (i.e., fastest swimming speed); (ii) tier #2 - swimmers with intermediate performances (i.e., intermediate swimming speed), and; (iii) tier #3 - swimmers with the poorest performances (i.e., slowest swimming speed). Prior to data collection, swimmers underwent familiarisation sessions in a 25 m indoor swimming pool and performed a standardised warm-up (Neiva et al., 2017). Data were collected on all 25 m trials and analysed over three consecutive stroke cycles between the 11th and 24th-meter marks. Swimmers were instructed to perform breathless stroke cycles during the 11th and 24th-meter marks to avoid disruptions/changes in stroke coordination or technique that could have a negative effect on swimming velocity (McCabe et al., 2015). A cohort comparison was performed between the three tiers by sex.
Anthropometrics assessmentHeight was measured with a digital stadiometer (Seca 242, Seca) at the nearest 0.1 cm, and body mass with a digital scale (BC-730, Tanita) at the nearest 0.1 kg. Arm span was measured by digital photogrammetry (Morais, et al., 2020a). For this, the swimmers were photographed next to a 2D calibration frame, in an orthostatic position, with both arms in lateral abduction at an angle of 90° in relation to the trunk. Both arms and fingers were fully extended. The distance between the tip of each third finger was measured with a dedicated software (Universal Desktop Ruler, AVPSoft, Pittsburgh, USA).
Swimming speed and speed fluctuation assessmentSwimmers were requested to perform three all-out trials in front-crawl with a push-off start. The best trial (i.e., the fastest) was used for further analysis. A mechanical apparatus (Swim speedo-meter, Swimsportec, Hildesheim, Germany) was attached to the hip (Morais et al., 2018). An in-house built software (LabVIEW®, v. 2010) was used to acquire (f = 50Hz) and stream speed-time series over the trial. Data was exported from the speedometer to an interface by a 12-bit resolution acquisition card (USB-6008, National Instruments, Austin, Texas, USA). Afterward, data was imported into a signal processing software (AcqKnowledge v. 3.9.0, Biopac Systems, Santa Barbara, USA). The signal was filtered with a Butterworth 4th order low-pass filter (cut-off: 5Hz) upon residual analysis (Winter, 2009). A video camera (Sony FDR-X3000, Japan) synchronised with the mechanical apparatus recorded the swimmer’s performance in the sagittal plane to identify the hand’s underwater motor path at a sampling rate of 60 Hz. It was placed 5 meters away and 20 cm deep, allowing the capture of three consecutive stroke cycles. Swimming speed (m/s) was retrieved from the software during each three consecutive stroke cycles (i.e., based on the hand entry that was closest to the video camera). The stroke frequency (SF, in Hz) was calculated as the number of cycles per unit of time from the time it takes to complete a full cycle (f = 1/P; in which P is the period). The stroke length was calculated as SL = v · SF (Craig and Pendergast, 1979), in which SL is the stroke length (m), v is the swimming speed (m/s), and SF is the stroke frequency (HZ). Figure 1 shows the speed-time series of each tier by sex. The stroke cycle is characterised by five key events: (i) the left hand catch; (ii) the transition between the downsweep and the insweep of the left upper limb; (iii) the transition between the left upper limb upsweep and the exit, and the right-hand catch; (iv) the transition between the downsweep and the insweep of the right upper limb; (v) the transition between the right upper limb upsweep and the exit, and the left-hand catch (Morais et al., 2020b). As mentioned earlier, the dv was calculated as being the CV.
Statistical analysisThe Kolmogorov-Smirnov test indicated a normal distribution for both swimming speed and dv. Mean plus one standard deviation, mean differences between tiers, and 95% confidence intervals (95CI) were computed as descriptive statistics. A two-way ANOVA (p < 0.05) was used to analyse the variance of the swimming speed and the dv as discrete variables. Thus, two factors were considered: (i) “sex”, and; (ii) “tier”. Simple main effects were used to check for significant differences between pairs (p < 0.05). For the variance effect size index, the eta square (η2) was computed and interpreted as: (i) without effect if 0 < η2 < 0.04; (ii) minimum if 0.04 < η2 < 0.25; (iii) moderate if 0.25 < η2 < 0.64 and; (iv) strong if η2 > 0.64 (Ferguson, 2009). Cohen’s d was used to estimate the standardised effect sizes between pairs, and deemed as: (i) trivial if 0 ≤ d < 0.20; (ii) small if 0.20 ≤ d < 0.60; (iii) moderate if 0.60 ≤ d < 1.20; (iv) large if 1.20 ≤ d < 2.00; (v) very large if 2.00 ≤ d < 4.00; (vi) nearly perfect if d ≥ 4.00 (Hopkins, 2019). Based on continuous variables, a two-way SPM ANOVA was used (Pataky, 2010). Once again, two factors were considered: (i) “sex”, and; (ii) “tier”. Simple main effects were used to verify for significant differences between pairs (p < 0.05). Prior to this analysis, each stroke cycle was normalised to its duration in an R routine (R Core Team, 2017). Cycle normalization is a method used to obtain a uniform representation of any cycle (i.e., standardisation) with the purpose of comparing or averaging data across participants. The usual method is based on representation of a percentage of the complete cycle. Otherwise, cycles between subjects could not be directly compared as they would present temporal differences. SPM analyses were implemented using the open-source spm1d code on Matlab (v.M0.1, www.spm1d.org).
Table 1 summarises the descriptive statistics according to sex and tiers. In addition to better performances, the boys in tier #1 also had lower dv, bigger anthropometric characteristics, faster SF, and greater SL, followed by swimmers in tier #2 and tier #3, respectively. As for girls, the trend observed in swimming speed was the same as for boys. However, girls in tier #2 were the ones with the lowest dv and fastest SF. The two-way ANOVA for the swimming speed revealed a significant sex effect (F1,114 = 151.813, p < 0.001, η2 = 0.57), a significant tier effect (F2,114 = 416.126, p < 0.001, η2 = 0.88), and a significant sex*tier interaction (F2,114 = 10.633, p < 0.001, η2 = 0.16). Regarding the dv, sex revealed a non-significant effect (F1,114 = 0.718, p = 0.399, η2 = 0.01), and tier a significant effect (F2,114 = 5.875, p = 0.004, η2 = 0.09). Notwithstanding, a non-significant sex*tier interaction was observed (F2,114 = 1.059, p = 0.350, η2 = 0.02). As a significant sex*tier interaction was noted for swimming speed, simple main effects were calculated. Table 2 presents the tier comparison by sex and by sex for each tier. In boys, the greatest difference in swimming speed was observed between swimmers in tier #1 and tier #3 (mean difference = 0.354 m/s, 95CI: 0.324 m/s to 0.383 m/s, p < 0.001, d = 6.52). The same trend was observed in girls (mean difference = 0.256 m/s, 95CI: 0.226 m/s to 0.286 m/s, p < 0.001, d = 4.64). Regarding the difference between sexes of the same tier, the greatest difference was noted in tier #1 (mean difference = 0.156 m/s, 95CI: 0.127 m/s to 0.186 m/s, p < 0.001, d = 3.37). Despite a non-significant sex*tier interaction observed by the dv, simple main effects were also calculated as shown in Table 2. Figure 2 shows the time-series comparison of swimming speed by SPM. As discrete values, SPM indicated a significant sex effect (Panel A: F = 9.272, p < 0.001) and a significant tier effect (Panel B: F = 6.215, p < 0.001) throughout the entire stroke cycle. A significant sex*tier interaction (Panel C) was observed between ~6% and ~18% (p = 0.001; corresponding to the downsweep phase of the left upper limb), between ~62% and ~72% (p = 0.005; corresponding to the downsweep phase of the right upper limb), and between ~88% and ~92% (p = 0.024; the upsweep phase of the right upper limb) of the stroke cycle. As a significant sex*tier interaction was observed in swimming speed, simple main effects were calculated. Figure 3 depicts these simple main effects by sex and tier. For boys (Panels A) and girls (Panels B), a significant difference (p < 0.05) was observed during the entire stroke cycle. Regarding the comparison between sexes of the same tier (Panels C), boys and girls in tiers #1 and #2 showed a significant difference (p < 0.05) throughout the entire stroke cycle. As for swimmers in tier #3, boys and girls differed significantly between ~2% and 3% (p = 0.043; the beginning of the downsweep phase of the left upper limb), and between ~44% and ~56% (p = 0.004; the exit of the left hand and the catch of the right hand) of the stroke cycle.
This study aimed to compare swimming speed during a complete stroke cycle in young swimmers of both sexes as a discrete variable and as a continuous variable (SPM). Swimming speed as a discrete variable presented a significant sex and tier effect, as well as a significant sex*tier interaction. By contrast, dv presented a non-significant sex effect, a significant tier effect, and a non-significant sex*tier interaction. SPM revealed that significant sex and tier effects were observed throughout the entire stroke cycle. As for the sex*tier interaction, SPM analysis allowed a more sensitive analysis of the swimming speed-time series. Significant differences were observed between ~6% and ~18%, ~62% and ~72%, and ~88% and ~92% of the stroke cycle. Thus, both hypotheses were accepted. Swimming research in both adult/elite and young swimmers has historically been performed based on discrete variables (Denadai et al., 2000; Psycharakis et al., 2010; Silva et al., 2019a). Swimming speed is one such case. The literature provides substantial evidence about a sex effect regarding swimming speed in both adult/elite (Seifert et al., 2007) and young swimmers (Dormehl and Osborough, 2015). However, research groups are still trying to understand what differentiates swimmers of the same competitive level or age group in both sexes (Barbosa et al., 2019; Seffrin et al., 2021; Silva et al., 2019b). Indeed, the data of the present study revealed a significant sex*tier interaction in swimming speed. Moreover, simple main effects (tiers in each sex, and sex by tier) indicated a significant difference between pairs. In general, the literature reports that within the same competitive level or age-group, swimmers with larger body dimensions present better stroke kinematics (i.e., faster SF and larger SL) and, consequently, faster swimming speeds (Barbosa et al., 2019). As observed in the present study, boys and girls included in the fastest tier presented bigger anthropometrics, faster SF, and larger SL. Moreover, boys presented bigger anthropometrics, faster SF, and larger SL than girls in a tier-by-tier comparison. For a similar age-group as the one assessed in the present study, it was observed that anthropometrics (namely arm span and height) (Alves et al., 2022; Morais et al., 2017) and stroke kinematics (Morais et al., 2017; Silva et al., 2019) were the main predictors of swimming speed. Thus, within the same competitive level or age-group, swimmers with larger anthropometric features, faster SF, and larger SL are more likely to present better performances. Regarding the dv, it is generally used as an overall indicator of the swimming speed variation/fluctuation over the stroke cycle (Barbosa et al., 2010). In the present study, the dv was higher in the poorest performance tiers (tier #3), regardless of sex, which is in accordance with the literature (Morais et al., 2013; Silva et al., 2019a). There is also evidence indicating that swimmers with lower dv present larger anthropometrics (Barbosa et al., 2019; Silva et al., 2019a). Thus, it can be stated that anthropometry has a positive and significant relationship with both swimming speed and dv. However, and in contrast with the swimming speed outputs, the dv did not present a significant sex*tier interaction. Consequently, all pairwise (tiers in each sex, and sex by tier) delivered by calculating simple main effects did not present significant differences. It can be argued that the dv based on the hypothesis raised in the introduction section can provide opposite findings to those verified by swimming speed. Indeed, it was reported that the dv may not always present a significant and negative effect on swimming speed (i.e., lower dv promotes faster swimming speeds) at least in maximal trials (Fernandes et al., 2022). Unlike discrete variables, continuous analyses can provide information about each time point in the time-series (Pataky, 2010). It turns out that, in human swimming, SPM (as a statistical procedure to analyse continuous variables) can identify significant differences at crucial moments in the stroke cycle that the dv is not able to provide. In this study, through SPM, it was possible to observe that the swimming speed presented significant sex and tier effects (during the entire stroke cycle), and a significant sex*tier interaction in some specific moments of the stroke cycle. Additionally, all pairwise (tiers in each sex) indicated a significant difference throughout the entire stroke cycle. This means that faster swimmers of both sexes were faster throughout the stroke cycle than their slower counterparts, and the same trend was observed when comparing tiers by sex. The transition between the downsweep and the insweep in both upper limbs was the key moment responsible for the fastest swimming speed in all tiers in both sexes. Therefore, it can be argued that this key moment could be the main factor that determines the difference in swimming speed between groups. However, comparison through SPM between all tiers by sex showed significant differences across the entire stroke cycle and not just at a specific key period. Nonetheless, it was argued that the transition between the pull and push (i.e., key moments #2 and #4) and the exit and early recovery phase were the most discriminating moments in muscle activity during the stroke cycle when analysed by SPM (Martens et al., 2016). Thus, it can be indicated that the differences observed between tiers within the stroke cycle may be related to different thrust or drag values at every single point of the stroke cycle. That is, swimmers with greater thrust during the entire stroke cycle or at specific key moments (Morais et al., 2020a) and with less drag (Morais et al., 2020b) can achieve faster swimming speeds. Regarding the comparison between boys and girls at similar performance tiers (i.e., tier-by-tier comparison between sexes), the dv as a discrete value showed non-significant differences between them based on simple main effects. Unlike comparisons between age-groups of the same sex, less information can be found about the comparison between boys and girls of the same competitive level. Nonetheless, the data of this study is in line with the study by Silva et al. (2019), which did not observe significant differences in the dv (as a discrete value) between boys and girls of the same competitive level (i.e., experts: boys vs girls; non-experts: boys vs girls). On the other hand, SPM identified significant differences throughout the stroke cycle between boys and girls in tiers #1 and #2 (boys being faster). Boys and girls are morphologically different, the former having bigger leverages and narrower waists (Nevill et al., 2020). Therefore, boys are likely to produce more thrust (Oliveira et al., 2021) and less active drag coefficient (Barbosa et al., 2015) than girls, leading to differences in speed. As mentioned earlier, measuring dv as a discrete variable was not sensitive enough to identify a significant sex*tier interaction (despite a significant tier effect). On the other hand, SPM was sensitive enough to do so between boys and girls in tiers #1 and #2, which occurred throughout the stroke cycle. Regarding the comparison between boys and girls with the poorest performances (tier #3, boys being faster), the SPM analysis revealed significant differences between ~2% and 3%, and between ~44% and ~56% (as opposed to discrete variables). This corresponds to the beginning of the downsweep phase of the left upper limb and the exit of the left hand and the catch of the right hand, respectively. Regarding the latter, at least in pubescent swimmers, it was shown that, despite having a greater hand surface area, the left upper limb (considered as non-dominant for most) provided less propulsive force (Morais et al., 2020c). Consequently, a slower swimming speed is achieved by this upper limb motion (Morais et al., 2020a). Notwithstanding, it should be mentioned that this was only observed during a short period of the stroke cycle. The former is related to the transition of propulsive phases between upper limbs. These swimmers followed the same training program regarding the motor control pattern in sprinting events or maximal trials. Nonetheless, it could be argued that measuring a motor control pattern could add deeper and more sensitive information to better understand the observed differences between tiers at that specific time. The index of coordination is a tool that measures the coordination of arm stroking by quantifying the lag time between the start of propulsion by one arm and the end of propulsion by the other (Chollet et al., 2000). Once again, when comparing pubescent boys and girls of the same competitive level, Silva et al. (2019) observed that boys had an index of coordination closer to zero than girls (despite non-significant differences). This indicates that boys tend to decrease the lag between their propulsive phases more than girls, leading them to a faster swimming speed. Using the swimming speed and dv as discrete variables can provide an overall understanding of the swimmers’ forward displacement and of the magnitude of acceleration and deceleration, respectively. Smaller speed fluctuation is related to mechanical efficiency and better performances (Barbosa et al., 2005). On the other hand, comparing swimming speed by SPM was more sensitive to differences between competitive levels in both sexes than by the CV of the dv. The former provides an overview of the stroke cycle motor behaviour. The latter provides details that allow identifying at which key moments of the cycle differences can be observed. Thus, swimming speed analysed as a discrete variable and as a continuous variable (SPM) can be used in a complementary way. By assessing swimming speed and dv as discrete variables, coaches and practitioners can quickly obtain an overall profile of the stroke cycle. On the other hand, choosing a continuous variable helps to identify which are the strongest and weakest key moments of the stroke cycle. Simultaneous selection of both discrete and continuous variables allows coaches and support staff to customise training programs and drills that each swimmer can perform to improve technique. As main limitations of this study, the following can be addressed: (i) the present assumptions are only for these age-groups; (ii) sprinting swimmers or trials in front-crawl; (iii) the study does not consider a hypothetical effect of breathing on swimming speed fluctuation, and; (iv) the measurement of the index of coordination could help to better understand the differences observed between tiers and sexes. Future studies about this topic should be carried out in different age-groups and events (swimming strokes and distances). Moreover, machine learning techniques can also help to statistically compare speed-time curves with another level of detail. Based on existing data, these algorithms can identify trends and patterns and draw conclusions for new unlabelled data sets.
Swimming speed compared as a discrete variable in age-group swimmers showed significant sex and tier effects and a significant sex*tier interaction. On the other hand, the dv as a discrete variable of swimming speed fluctuations indicated non-significant effects and interaction. Comparing the swimming speed time-curve as a continuous variable by SPM revealed significant sex and tier effects and a significant sex*tier interaction in some moments of the stroke cycle. Thus, it made it possible to understand where within the stroke cycle boys and girls of different tiers differed. In summary, swimming speed and dv as discrete variables and through SPM (continuous variables) provide additional details about the swimmers’ stroke cycle. Both should be considered in swimming research and sports science services for swimmers. Furthermore, SPM, which has been increasingly employed in various land-based human movements, has also shown its usefulness in human swimming.
ACKNOWLEDGEMENTS |
This work is supported by national funds (FCT - Portuguese Foundation for Science and Technology) under the project UIDB/DTP/04045/2020. The experiments comply with the current laws of the country in which they were performed. The authors have no conflict of interest to declare. The datasets generated and analyzed during the current study are not publicly available, but are available from the corresponding author who was an organizer of the study. |
|
AUTHOR BIOGRAPHY |
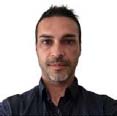 |
Jorge E. Morais |
Employment: Researcher at the University of Beira Interior, Professor in the Polytechnic Institute of Bragança and Member of the Research Centre in Sports, Health and Human Development (Portugal). |
Degree: PhD. |
Research interests: The biomechanical and physiological determinant factors of aquatic activities. |
E-mail: morais.jorgestrela@gmail.com |
|
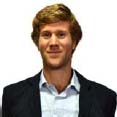 |
Daniel A. Marinho |
Employment: Professor in the University of Beira Interior and Member of the Research Centre in Sports, Health and Human Development (Portugal). |
Degree: PhD. |
Research interests: The biomechanical and physiological determinant factors of aquatic activities. |
E-mail: marinho.d@gmail.com |
|
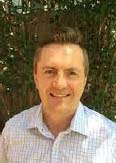 |
Stephen Cobley |
Employment: Associate Professor - Discipline of Exercise & Sport, Faculty of Medicine & Health, The University of Sydney. |
Degree: PhD. |
Research interests: Multi-disciplinary Athlete Development; Psychology; Adolescence; Youth. |
E-mail: stephen.cobley@sydney.edu.au |
|
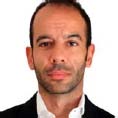 |
Tiago M. Barbosa |
Employment: Professor at the Polytechnic Institute of Bragança and Member of the Research Centre in Sports, Health and Human Development (Portugal). |
Degree: PhD, Dr Habil. |
Research interests: The biomechanical and physiological determinant factors of aquatic activities. |
E-mail: barbosa@ipb.pt |
|
|
|
REFERENCES |
 Alves M., Carvalho D.D., Fernandes R.J., Vilas-Boas J.P. (2022) How anthropometrics of young and adolescent swimmers influence stroking parameters and performance? A systematic review. International Journal of Environmental Research and Public Health 19, 2543. Crossref
|
 Barbosa T.M., Bartolomeu R., Morais J.E., Costa M.J. (2019) Skillful swimming in age-groups is determined by anthropometrics, biomechanics and energetics. Frontiers in Physiology 10, 73. Crossref
|
 Barbosa T.M., Bragada J.A., Reis V.M., Marinho D.A., Carvalho C., Silva A.J. (2010) Energetics and biomechanics as determining factors of swimming performance: updating the state of the art. Journal of Science and Medicine in Sport 13, 262-269. Crossref
|
 Barbosa T.M., Goh W.X., Morais J.E., Costa M.J., Pendergast D. (2016) Comparison of classical kinematics, entropy and fractal properties as measures of complexity of the motor system in swimming. Frontiers in Psychology 7, 1566. Crossref
|
 Barbosa T.M., Keskinen K.L., Fernandes R., Colaço P., Lima A.B., Vilas-Boas J.P. (2005) Energy cost and intracyclic variation of the velocity of the centre of mass in butterfly stroke. European Journal of Applied Physiology 93, 519-523. Crossref
|
 Barbosa T.M., Morais J.E., Marques M.C., Silva A.J., Marinho D.A., Kee Y.H. (2015) Hydrodynamic profile of young swimmers: changes over a competitive season. Scandinavian Journal of Medicine and Science in Sports 25, 184-196. Crossref
|
 Bertozzi F., Porcelli S., Marzorati M., Pilotto A.M., Galli M., Sforza C., Zago M. (2022) Whole-body kinematics during a simulated sprint in flat-water kayakers. European Journal of Sport Science 22, 817-825. Crossref
|
 Chollet D., Chalies S., Chatard J.C. (2000) A new index of coordination for the crawl: description and usefulness. International Journal of Sports Medicine 21, 54-59. Crossref
|
 Craig A.B., Pendergast D.R. (1979) Relationships of stroke rate, distance per stroke and velocity in competitive swimming. Medicine and Science in Sports 11, 278-283. Crossref
|
 Denadai B.S., Greco C.C., Teixeira M. (2000) Blood lactate response and critical speed in swimmers aged 10-12 years of different standards. Journal of Sports Sciences 18, 779-784. Crossref
|
 Dormehl S., Osborough C. (2015) Effect of age, sex and race distance on front crawl stroke parameters in subelite adolescent swimmers during competition. Pediatric Exercise Science 27, 334-344. Crossref
|
 Ferguson C.J. (2009) An effect size primer: A guide for clinicians and researchers. Professional Psychology: Research and Practice 40, 532-538. Crossref
|
 Fernandes A., Mezêncio B., Soares S., Duarte Carvalho D., Silva A., Vilas-Boas J.P., Fernandes R.J. (2022) Intra-and inter-cycle velocity variations in sprint front crawl swimming. Sports Biomechanics. [Epub ahead of print]. Crossref
|
 Figueiredo P., Sanders R., Gorski T., Vilas-Boas J.P., Fernandes R. J. (2013) Kinematic and electromyographic changes during 200 m front crawl at race pace. International Journal of Sports Medicine 34, 49-55. Crossref
|
 Figueiredo P., Silva A., Sampaio A., Vilas-Boas J.P., Fernandes R.J. (2016) Front crawl sprint performance: A cluster analysis of biomechanics, energetics, coordinative and anthropometric determinants in young swimmers. Motor Control 20, 209-221. Crossref
|
 Gonjo T., Fernandes R. J., Vilas-Boas J. P., Sanders R. (2023) Is the use of coefficient of variation a valid way to assess the swimming intra-cycle velocity fluctuation?. Journal of Science and Medicine in Sport. Crossref
|
 Gourgoulis V., Aggeloussis N., Vezos N., Kasimatis P., Antoniou P., Mavromatis G. (2008) Estimation of hand forces and propelling efficiency during front crawl swimming with hand paddles. Journal of Biomechanics 41, 208-215. Crossref
|
 Gourgoulis V., Nikodelis T. (2022) Comparison of the arm-stroke kinematics between maximal and sub-maximal breaststroke swimming using discrete data and time series analysis. Journal of Biomechanics 142, 111255. Crossref
|
 Hopkins, W.G. (2019) A scale of magnitudes for effect statistics. A new view of statistics. 2002. Internet http://sportsci. org/resource/stats/effectmag. html (10 October 2013).
|
 Kwok W.Y., So B.C.L., Tse D.H.T., Ng S.S.M. (2021) A systematic review and meta-analysis: biomechanical evaluation of the effectiveness of strength and conditioning training programs on front crawl swimming performance. Journal of Sports Science and Medicine 20, 564-585. Crossref
|
 Martens J., Daly D., Deschamps K., Staes F., Fernandes R.J. (2016) Inter-individual variability and pattern recognition of surface electromyography in front crawl swimming. Journal of Electromyography and Kinesiology 31, 14-21. Crossref
|
 Matsuda Y., Yamada Y., Ikuta Y., Nomura T., Oda S. (2014) Intracyclic velocity variation and arm coordination for different skilled swimmers in the front crawl. Journal of Human Kinetics 44, 67. Crossref
|
 McCabe C.B., Sanders R.H., Psycharakis S.G. (2015) Upper limb kinematic differences between breathing and non-breathing conditions in front crawl sprint swimming. Journal of Biomechanics 48, 3995-4001. Crossref
|
 McKay A.K., Stellingwerff T., Smith E.S., Martin D.T., Mujika I., Goosey-Tolfrey V.L., Sheppard J., Burke L. M. (2022) Defining training and performance caliber: a participant classification framework. International Journal of Sports Physiology and Performance 17, 317-331. Crossref
|
 Morais J.E., Forte P., Nevill A.M., Barbosa T.M., Marinho D.A. (2020a) Upper-limb kinematics and kinetics imbalances in the determinants of front-crawl swimming at maximal speed in young international level swimmers. Scientific Reports 10, 1-8. Crossref
|
 Morais J.E., Garrido N.D., Marques M.C., Silva A.J., Marinho D.A., Barbosa T.M. (2013) The influence of anthropometric, kinematic and energetic variables and gender on swimming performance in youth athletes. Journal of Human Kinetics 39, 203. Crossref
|
 Morais J.E., Marques M.C., Rodríguez-Rosell D., Barbosa T.M., Marinho D.A. (2020c) Relationship between thrust, anthropometrics and dry-land strength in a national junior swimming team. The Physician and Sportsmedicine 48, 304-311. Crossref
|
 Morais J.E., Sanders R.H., Papic C., Barbosa T.M., Marinho D.A. (2020b) The influence of the frontal surface area and swim velocity variation in front crawl active drag. Medicine and Science in Sports and Exercise 52, 2357-2364. Crossref
|
 Morais J.E., Silva A.J., Garrido N.D., Marinho D.A., Barbosa T.M. (2018) The transfer of strength and power into the stroke biomechanics of young swimmers over a 34-week period. European Journal of Sport Science 18, 787-795. Crossref
|
 Morais J.E., Silva A.J., Marinho D.A., Lopes V.P., Barbosa T.M. (2017) Determinant factors of long-term performance development in young swimmers. International Journal of Sports Physiology and Performance 12, 198-205. Crossref
|
 Neiva H.P., Marques M.C., Barbosa T.M., Izquierdo M., Viana J.L., Marinho D.A. (2017) Effects of 10 min vs. 20 min passive rest after warm-up on 100 m freestyle time-trial performance: A randomized crossover study. Journal of Science and Medicine in Sport 20, 81-86. Crossref
|
 Nevill A.M., Negra Y., Myers T.D., Sammoud S., Chaabene H. (2020) Key somatic variables associated with and differences between the 4 swimming strokes. Journal of Sports Sciences 38, 787-794. Crossref
|
 Nikolaidis P.T., Knechtle B. (2017) Pacing in age-group freestyle swimmers at The XV FINA World Masters Championships in Montreal 2014. Journal of Sports Sciences 35, 1165-1172. Crossref
|
 Oliveira M., Henrique R.S., Queiroz D.R., Salvina M., Melo W.V., Moura dos Santos M.A. (2021) Anthropometric variables, propulsive force and biological maturation: A mediation analysis in young swimmers. European Journal of Sport Science 21, 507-514. Crossref
|
 Pataky T.C. (2010) Generalized n-dimensional biomechanical field analysis using statistical parametric mapping. Journal of Biomechanics 43, 1976-1982. Crossref
|
 Pataky T.C. (2012) One-dimensional statistical parametric mapping in Python. Computer Methods in Biomechanics and Biomedical Engineering 15, 295-301. Crossref
|
 Preatoni E., Hamill J., Harrison A.J., Hayes K., Van Emmerik R.E., Wilson C., Rodano R. (2013) Movement variability and skills monitoring in sports. Sports Biomechanics 12, 69-92. Crossref
|
 Psycharakis S.G., Naemi R., Connaboy C., McCabe C., Sanders R.H. (2010) Three-dimensional analysis of intracycle velocity fluctuations in frontcrawl swimming. Scandinavian Journal of Medicine and Science in Sports 20, 128-135. Crossref
|
 R Core Team. (2017) R: A language and environment for statistical computing. R Foundation for Statistical Computing.
|
 Reed G.F., Lynn F., Meade B.D. (2002) Use of coefficient of variation in assessing variability of quantitative assays. Clinical and Vaccine Immunology 9, 1235-1239. Crossref
|
 Richter C., Marshall B., Moran K. (2014) Comparison of discrete-point vs. dimensionality-reduction techniques for describing performance-related aspects of maximal vertical jumping. Journal of Biomechanics 47, 3012-3017. Crossref
|
 Ruiz-Navarro J.J., Cano-Adamuz M., Andersen J.T., Cuenca-Fernández F., López-Contreras G., Vanrenterghem J., Arellano R. (2021) Understanding the effects of training on underwater undulatory swimming performance and kinematics. Sports Biomechanics. Crossref
|
 Seffrin A., Barbosa de Lira C.A., Nikolaidis P.T., Knechtle B., Andrade M.S. (2021) Age-related performance determinants of young swimmers in 100-and 400-m events. Journal of Sports Medicine and Physical Fitness. [Epub ahead of print]
|
 Seifert L., Chollet D., Chatard J. C. (2007) Kinematic changes during a 100-m front crawl: effects of performance level and gender. Medicine and Science in Sports and Exercise 39, 1784. Crossref
|
 Seifert L., Toussaint H.M., Alberty M., Schnitzler C., Chollet D. (2010) Arm coordination, power and swim efficiency in national and regional front crawl swimmers. Human Movement Science 29, 426-439. Crossref
|
 Silva A.F., Figueiredo P., Ribeiro J., Alves F., Vilas-Boas J.P., Seifert L., Fernandes R.J. (2019) Integrated analysis of young swimmers’ sprint performance. Motor Control 23, 354-364. Crossref
|
 Tassignon B., Serrien B., De Pauw K., Baeyens J.P., Meeusen R. (2018) Continuous knee cooling affects functional hop performance-A randomized controlled trial. Journal of Sports Science and Medicine 17, 322-329. Crossref
|
 Warmenhoven J., Harrison A., Robinson M.A., Vanrenterghem J., Bargary N., Smith R., Cobley S., Draper C., Connelly T., Pataky T. (2018) A force profile analysis comparison between functional data analysis, statistical parametric mapping and statistical non-parametric mapping in on-water single sculling. Journal of Science and Medicine in Sport 21, 1100-1105. Crossref
|
 Winter, D.A. (2009) Biomechanics and motor control of human movement. John Wiley & Sons.
|
|
|
|
|
|
|